کد مقاله | کد نشریه | سال انتشار | مقاله انگلیسی | نسخه تمام متن |
---|---|---|---|---|
4969835 | 1449984 | 2017 | 14 صفحه PDF | دانلود رایگان |
عنوان انگلیسی مقاله ISI
Comparing two classes of end-to-end machine-learning models in lung nodule detection and classification: MTANNs vs. CNNs
دانلود مقاله + سفارش ترجمه
دانلود مقاله ISI انگلیسی
رایگان برای ایرانیان
کلمات کلیدی
موضوعات مرتبط
مهندسی و علوم پایه
مهندسی کامپیوتر
چشم انداز کامپیوتر و تشخیص الگو
پیش نمایش صفحه اول مقاله
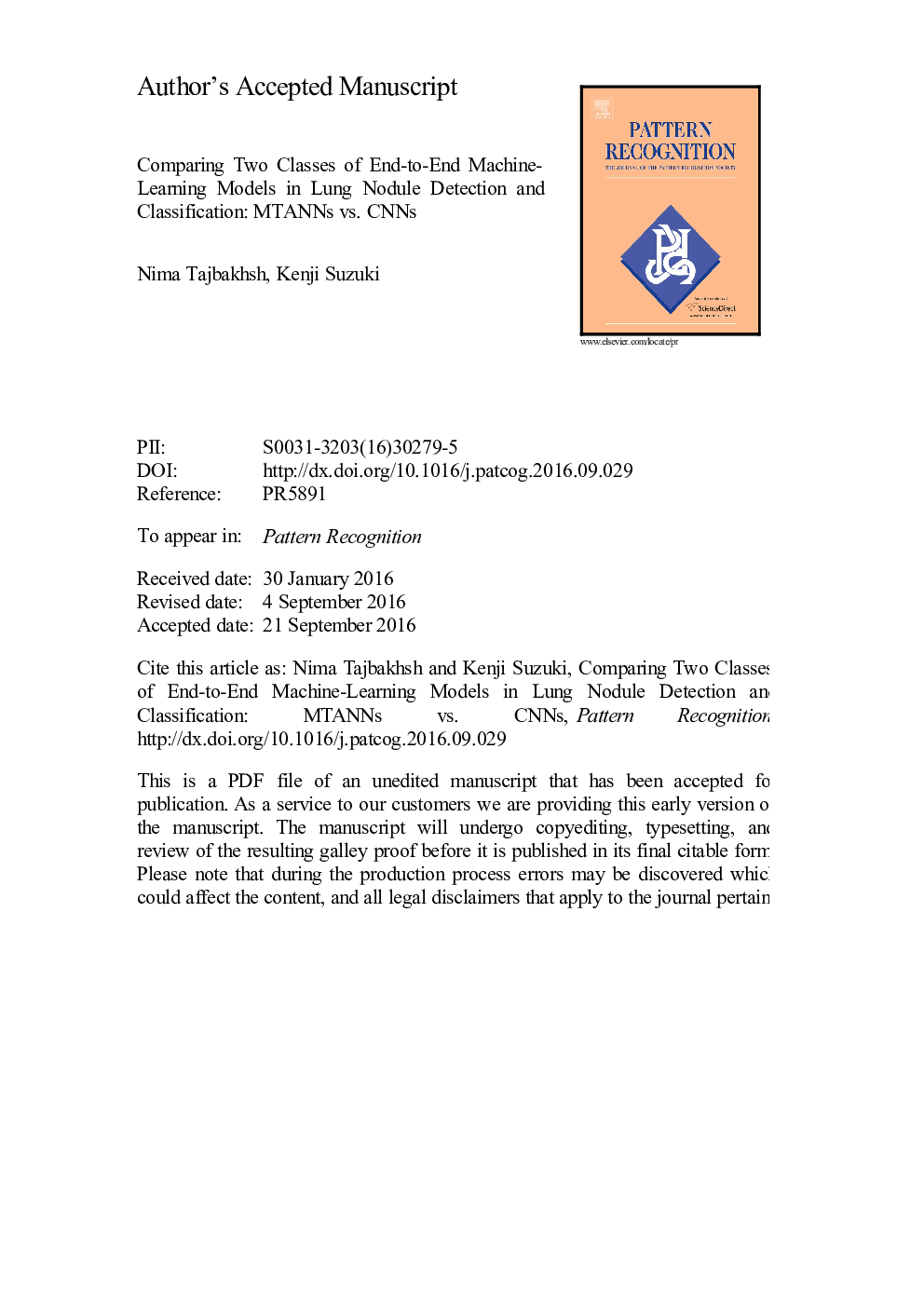
چکیده انگلیسی
End-to-end learning machines enable a direct mapping from the raw input data to the desired outputs, eliminating the need for hand-crafted features. Despite less engineering effort than the hand-crafted counterparts, these learning machines achieve extremely good results for many computer vision and medical image analysis tasks. Two dominant classes of end-to-end learning machines are massive-training artificial neural networks (MTANNs) and convolutional neural networks (CNNs). Although MTANNs have been actively used for a number of medical image analysis tasks over the past two decades, CNNs have recently gained popularity in the field of medical imaging. In this study, we have compared these two successful learning machines both experimentally and theoretically. For that purpose, we considered two well-studied topics in the field of medical image analysis: detection of lung nodules and distinction between benign and malignant lung nodules in computed tomography (CT). For a thorough analysis, we used 2 optimized MTANN architectures and 4 distinct CNN architectures that have different depths. Our experiments demonstrated that the performance of MTANNs was substantially higher than that of CNN when using only limited training data. With a larger training dataset, the performance gap became less evident even though the margin was still significant. Specifically, for nodule detection, MTANNs generated 2.7 false positives per patient at 100% sensitivity, which was significantly (p<0.05) lower than the best performing CNN model with 22.7 false positives per patient at the same level of sensitivity. For nodule classification, MTANNs yielded an area under the receiver-operating-characteristic curve (AUC) of 0.8806 (95% CI: 0.8389-0.9223), which was significantly (p<0.05) greater than the best performing CNN model with an AUC of 0.7755 (95% CI: 0.7120-0.8270). Thus, with limited training data, MTANNs would be a suitable end-to-end machine-learning model for detection and classification of focal lesions that do not require high-level semantic features.
ناشر
Database: Elsevier - ScienceDirect (ساینس دایرکت)
Journal: Pattern Recognition - Volume 63, March 2017, Pages 476-486
Journal: Pattern Recognition - Volume 63, March 2017, Pages 476-486
نویسندگان
Nima Tajbakhsh, Kenji Suzuki,