کد مقاله | کد نشریه | سال انتشار | مقاله انگلیسی | نسخه تمام متن |
---|---|---|---|---|
497402 | 862891 | 2009 | 8 صفحه PDF | دانلود رایگان |
عنوان انگلیسی مقاله ISI
Evolutionary multi-feature construction for data reduction: A case study
دانلود مقاله + سفارش ترجمه
دانلود مقاله ISI انگلیسی
رایگان برای ایرانیان
کلمات کلیدی
موضوعات مرتبط
مهندسی و علوم پایه
مهندسی کامپیوتر
نرم افزارهای علوم کامپیوتر
پیش نمایش صفحه اول مقاله
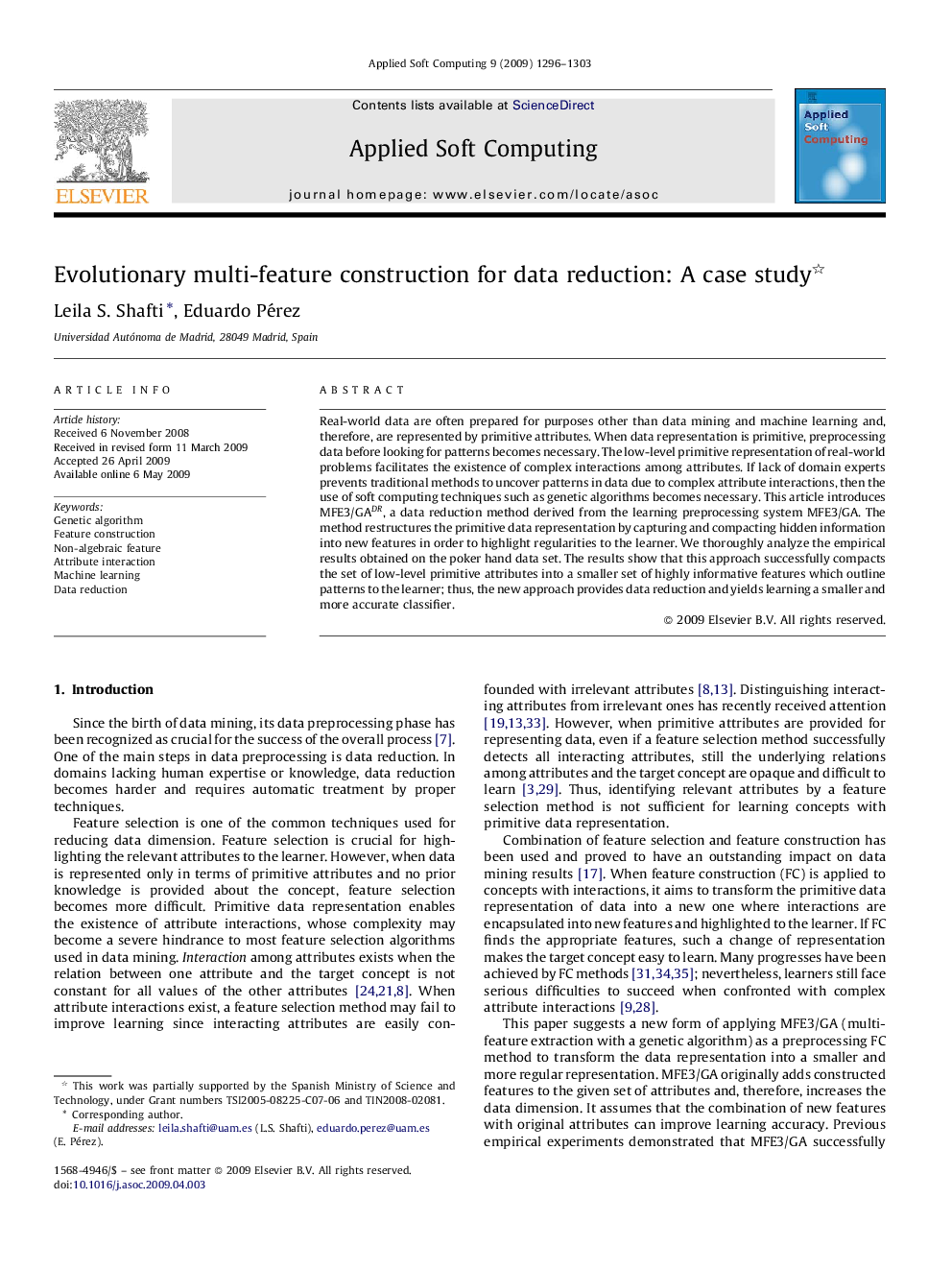
چکیده انگلیسی
Real-world data are often prepared for purposes other than data mining and machine learning and, therefore, are represented by primitive attributes. When data representation is primitive, preprocessing data before looking for patterns becomes necessary. The low-level primitive representation of real-world problems facilitates the existence of complex interactions among attributes. If lack of domain experts prevents traditional methods to uncover patterns in data due to complex attribute interactions, then the use of soft computing techniques such as genetic algorithms becomes necessary. This article introduces MFE3/GADR, a data reduction method derived from the learning preprocessing system MFE3/GA. The method restructures the primitive data representation by capturing and compacting hidden information into new features in order to highlight regularities to the learner. We thoroughly analyze the empirical results obtained on the poker hand data set. The results show that this approach successfully compacts the set of low-level primitive attributes into a smaller set of highly informative features which outline patterns to the learner; thus, the new approach provides data reduction and yields learning a smaller and more accurate classifier.
ناشر
Database: Elsevier - ScienceDirect (ساینس دایرکت)
Journal: Applied Soft Computing - Volume 9, Issue 4, September 2009, Pages 1296-1303
Journal: Applied Soft Computing - Volume 9, Issue 4, September 2009, Pages 1296-1303
نویسندگان
Leila S. Shafti, Eduardo Pérez,