کد مقاله | کد نشریه | سال انتشار | مقاله انگلیسی | نسخه تمام متن |
---|---|---|---|---|
4976958 | 1451842 | 2017 | 14 صفحه PDF | دانلود رایگان |
عنوان انگلیسی مقاله ISI
Bearing performance degradation assessment based on a combination of empirical mode decomposition and k-medoids clustering
دانلود مقاله + سفارش ترجمه
دانلود مقاله ISI انگلیسی
رایگان برای ایرانیان
کلمات کلیدی
موضوعات مرتبط
مهندسی و علوم پایه
مهندسی کامپیوتر
پردازش سیگنال
پیش نمایش صفحه اول مقاله
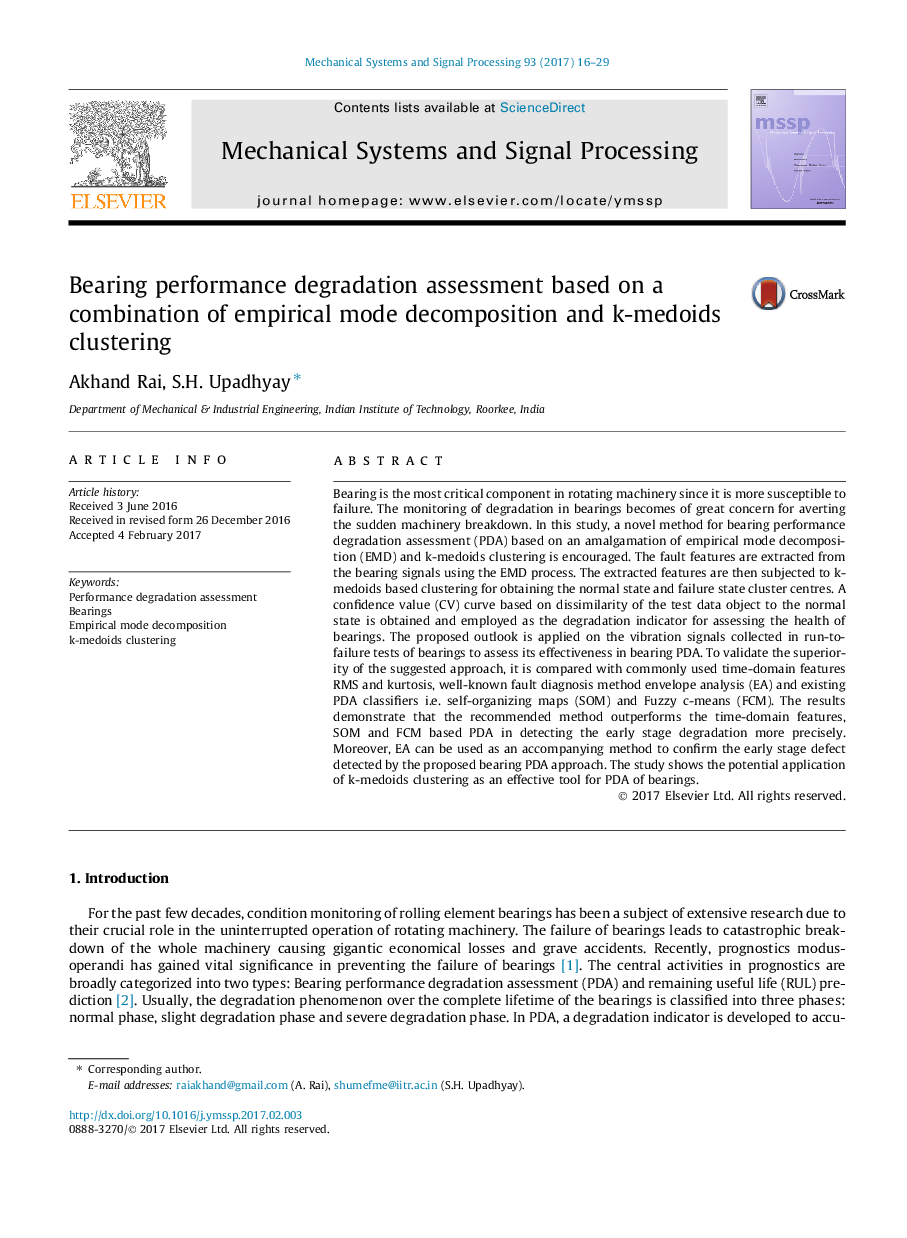
چکیده انگلیسی
Bearing is the most critical component in rotating machinery since it is more susceptible to failure. The monitoring of degradation in bearings becomes of great concern for averting the sudden machinery breakdown. In this study, a novel method for bearing performance degradation assessment (PDA) based on an amalgamation of empirical mode decomposition (EMD) and k-medoids clustering is encouraged. The fault features are extracted from the bearing signals using the EMD process. The extracted features are then subjected to k-medoids based clustering for obtaining the normal state and failure state cluster centres. A confidence value (CV) curve based on dissimilarity of the test data object to the normal state is obtained and employed as the degradation indicator for assessing the health of bearings. The proposed outlook is applied on the vibration signals collected in run-to-failure tests of bearings to assess its effectiveness in bearing PDA. To validate the superiority of the suggested approach, it is compared with commonly used time-domain features RMS and kurtosis, well-known fault diagnosis method envelope analysis (EA) and existing PDA classifiers i.e. self-organizing maps (SOM) and Fuzzy c-means (FCM). The results demonstrate that the recommended method outperforms the time-domain features, SOM and FCM based PDA in detecting the early stage degradation more precisely. Moreover, EA can be used as an accompanying method to confirm the early stage defect detected by the proposed bearing PDA approach. The study shows the potential application of k-medoids clustering as an effective tool for PDA of bearings.
ناشر
Database: Elsevier - ScienceDirect (ساینس دایرکت)
Journal: Mechanical Systems and Signal Processing - Volume 93, 1 September 2017, Pages 16-29
Journal: Mechanical Systems and Signal Processing - Volume 93, 1 September 2017, Pages 16-29
نویسندگان
Akhand Rai, S.H. Upadhyay,