کد مقاله | کد نشریه | سال انتشار | مقاله انگلیسی | نسخه تمام متن |
---|---|---|---|---|
5009711 | 1462049 | 2017 | 11 صفحه PDF | دانلود رایگان |
عنوان انگلیسی مقاله ISI
A novel framework for analyzing MOS E-nose data based on voting theory: Application to evaluate the internal quality of Chinese pecans
دانلود مقاله + سفارش ترجمه
دانلود مقاله ISI انگلیسی
رایگان برای ایرانیان
کلمات کلیدی
موضوعات مرتبط
مهندسی و علوم پایه
شیمی
شیمی آنالیزی یا شیمی تجزیه
پیش نمایش صفحه اول مقاله
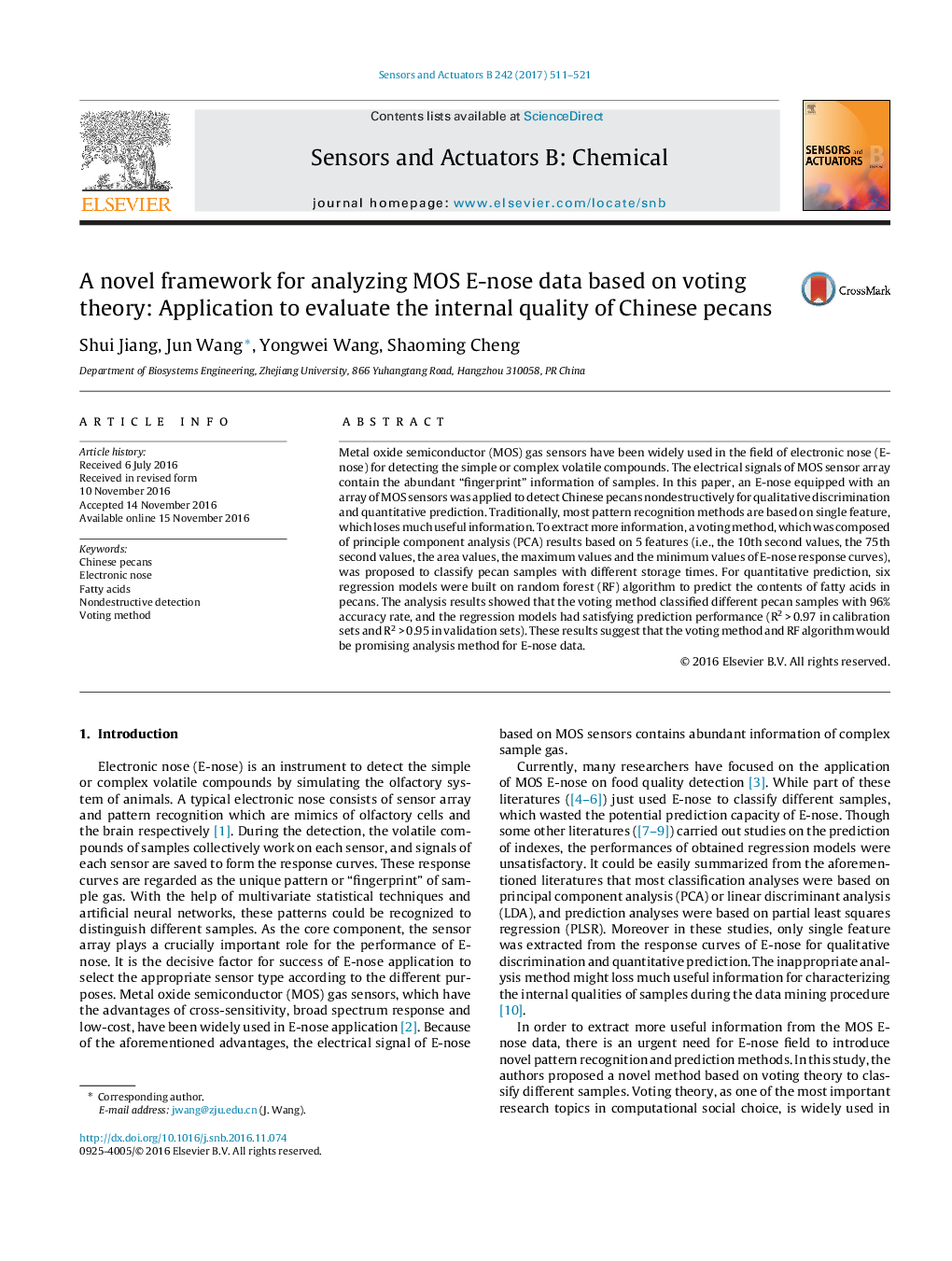
چکیده انگلیسی
Metal oxide semiconductor (MOS) gas sensors have been widely used in the field of electronic nose (E-nose) for detecting the simple or complex volatile compounds. The electrical signals of MOS sensor array contain the abundant “fingerprint” information of samples. In this paper, an E-nose equipped with an array of MOS sensors was applied to detect Chinese pecans nondestructively for qualitative discrimination and quantitative prediction. Traditionally, most pattern recognition methods are based on single feature, which loses much useful information. To extract more information, a voting method, which was composed of principle component analysis (PCA) results based on 5 features (i.e., the 10th second values, the 75th second values, the area values, the maximum values and the minimum values of E-nose response curves), was proposed to classify pecan samples with different storage times. For quantitative prediction, six regression models were built on random forest (RF) algorithm to predict the contents of fatty acids in pecans. The analysis results showed that the voting method classified different pecan samples with 96% accuracy rate, and the regression models had satisfying prediction performance (R2Â >Â 0.97 in calibration sets and R2Â >Â 0.95 in validation sets). These results suggest that the voting method and RF algorithm would be promising analysis method for E-nose data.
ناشر
Database: Elsevier - ScienceDirect (ساینس دایرکت)
Journal: Sensors and Actuators B: Chemical - Volume 242, April 2017, Pages 511-521
Journal: Sensors and Actuators B: Chemical - Volume 242, April 2017, Pages 511-521
نویسندگان
Shui Jiang, Jun Wang, Yongwei Wang, Shaoming Cheng,