کد مقاله | کد نشریه | سال انتشار | مقاله انگلیسی | نسخه تمام متن |
---|---|---|---|---|
5027511 | 1470635 | 2017 | 11 صفحه PDF | دانلود رایگان |
عنوان انگلیسی مقاله ISI
Evaluating the Suitability of Existing Rock Mass Classification Systems for TBM Performance Prediction by using a Regression Tree
دانلود مقاله + سفارش ترجمه
دانلود مقاله ISI انگلیسی
رایگان برای ایرانیان
کلمات کلیدی
موضوعات مرتبط
مهندسی و علوم پایه
سایر رشته های مهندسی
مهندسی (عمومی)
پیش نمایش صفحه اول مقاله
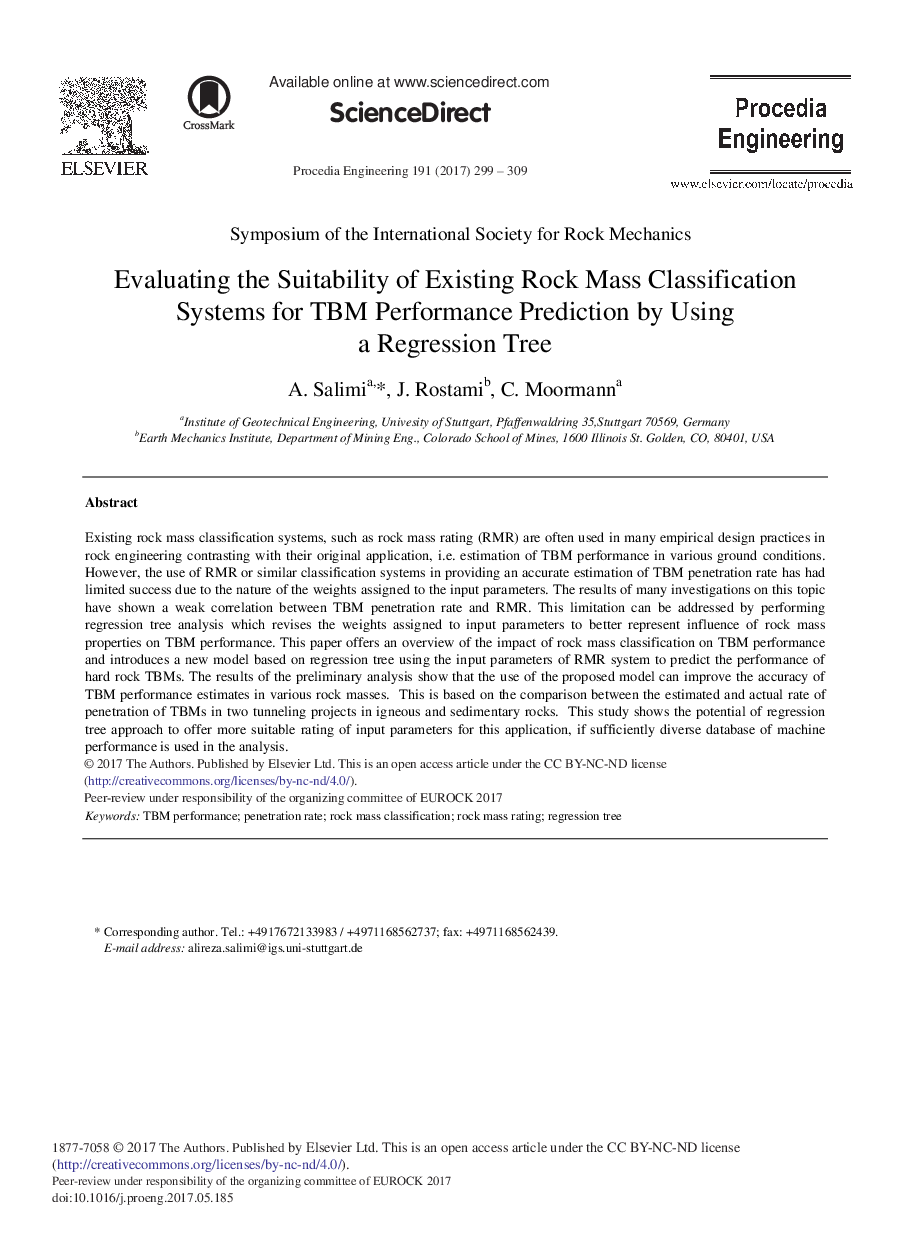
چکیده انگلیسی
Existing rock mass classification systems, such as rock mass rating (RMR) are often used in many empirical design practices in rock engineering contrasting with their original application, i.e. estimation of TBM performance in various ground conditions. However, the use of RMR or similar classification systems in providing an accurate estimation of TBM penetration rate has had limited success due to the nature of the weights assigned to the input parameters. The results of many investigations on this topic have shown a weak correlation between TBM penetration rate and RMR. This limitation can be addressed by performing regression tree analysis which revises the weights assigned to input parameters to better represent influence of rock mass properties on TBM performance. This paper offers an overview of the impact of rock mass classification on TBM performance and introduces a new model based on regression tree using the input parameters of RMR system to predict the performance of hard rock TBMs. The results of the preliminary analysis show that the use of the proposed model can improve the accuracy of TBM performance estimates in various rock masses. This is based on the comparison between the estimated and actual rate of penetration of TBMs in two tunneling projects in igneous and sedimentary rocks. This study shows the potential of regression tree approach to offer more suitable rating of input parameters for this application, if sufficiently diverse database of machine performance is used in the analysis.
ناشر
Database: Elsevier - ScienceDirect (ساینس دایرکت)
Journal: Procedia Engineering - Volume 191, 2017, Pages 299-309
Journal: Procedia Engineering - Volume 191, 2017, Pages 299-309
نویسندگان
A. Salimi, J. Rostami, C. Moormann,