کد مقاله | کد نشریه | سال انتشار | مقاله انگلیسی | نسخه تمام متن |
---|---|---|---|---|
507067 | 865089 | 2008 | 9 صفحه PDF | دانلود رایگان |
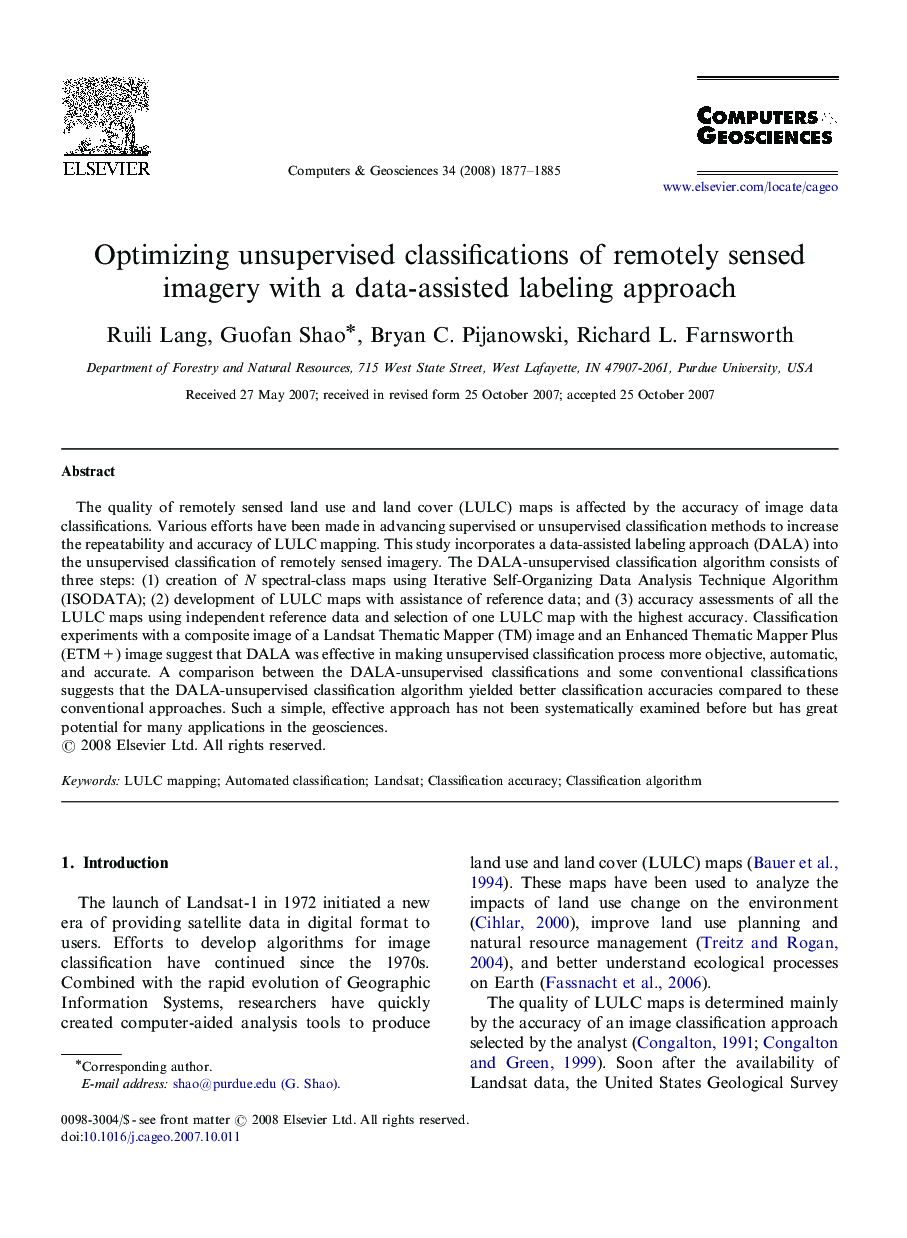
The quality of remotely sensed land use and land cover (LULC) maps is affected by the accuracy of image data classifications. Various efforts have been made in advancing supervised or unsupervised classification methods to increase the repeatability and accuracy of LULC mapping. This study incorporates a data-assisted labeling approach (DALA) into the unsupervised classification of remotely sensed imagery. The DALA-unsupervised classification algorithm consists of three steps: (1) creation of N spectral-class maps using Iterative Self-Organizing Data Analysis Technique Algorithm (ISODATA); (2) development of LULC maps with assistance of reference data; and (3) accuracy assessments of all the LULC maps using independent reference data and selection of one LULC map with the highest accuracy. Classification experiments with a composite image of a Landsat Thematic Mapper (TM) image and an Enhanced Thematic Mapper Plus (ETM+) image suggest that DALA was effective in making unsupervised classification process more objective, automatic, and accurate. A comparison between the DALA-unsupervised classifications and some conventional classifications suggests that the DALA-unsupervised classification algorithm yielded better classification accuracies compared to these conventional approaches. Such a simple, effective approach has not been systematically examined before but has great potential for many applications in the geosciences.
Journal: Computers & Geosciences - Volume 34, Issue 12, December 2008, Pages 1877–1885