کد مقاله | کد نشریه | سال انتشار | مقاله انگلیسی | نسخه تمام متن |
---|---|---|---|---|
507733 | 865141 | 2012 | 10 صفحه PDF | دانلود رایگان |
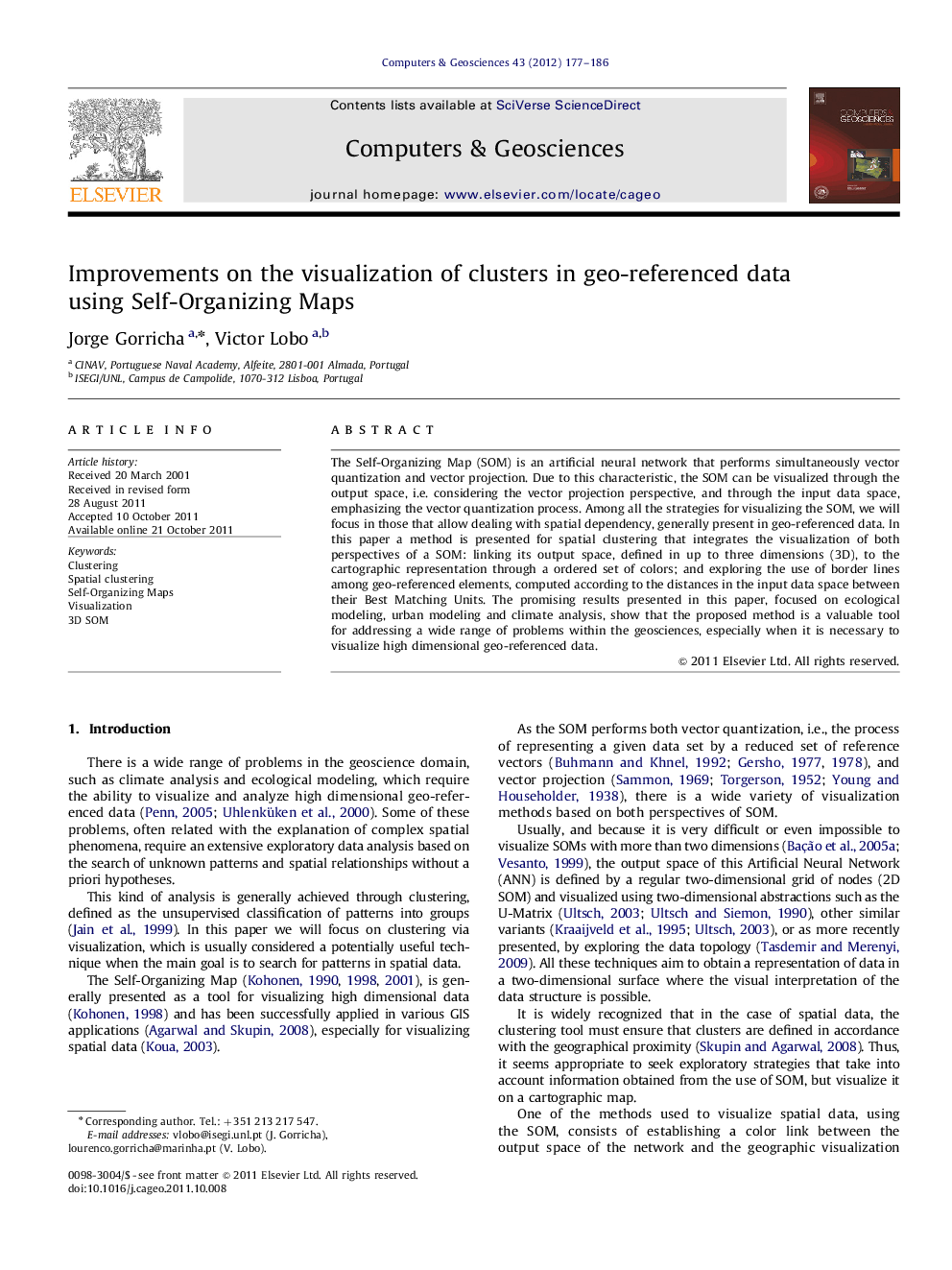
The Self-Organizing Map (SOM) is an artificial neural network that performs simultaneously vector quantization and vector projection. Due to this characteristic, the SOM can be visualized through the output space, i.e. considering the vector projection perspective, and through the input data space, emphasizing the vector quantization process. Among all the strategies for visualizing the SOM, we will focus in those that allow dealing with spatial dependency, generally present in geo-referenced data. In this paper a method is presented for spatial clustering that integrates the visualization of both perspectives of a SOM: linking its output space, defined in up to three dimensions (3D), to the cartographic representation through a ordered set of colors; and exploring the use of border lines among geo-referenced elements, computed according to the distances in the input data space between their Best Matching Units. The promising results presented in this paper, focused on ecological modeling, urban modeling and climate analysis, show that the proposed method is a valuable tool for addressing a wide range of problems within the geosciences, especially when it is necessary to visualize high dimensional geo-referenced data.
► A new method for visualizing clusters in geo-referenced data that is proposed.
► Self-Organizing Map (SOM) output space is linked to the cartographic map.
► Border lines between geo-referenced elements are proposed for clustering.
► The results show an increase in the clustering quality due to use of 3D SOMs.
► The inclusion of border lines proved to be very effective for clustering.
Journal: Computers & Geosciences - Volume 43, June 2012, Pages 177–186