کد مقاله | کد نشریه | سال انتشار | مقاله انگلیسی | نسخه تمام متن |
---|---|---|---|---|
507878 | 865152 | 2013 | 10 صفحه PDF | دانلود رایگان |
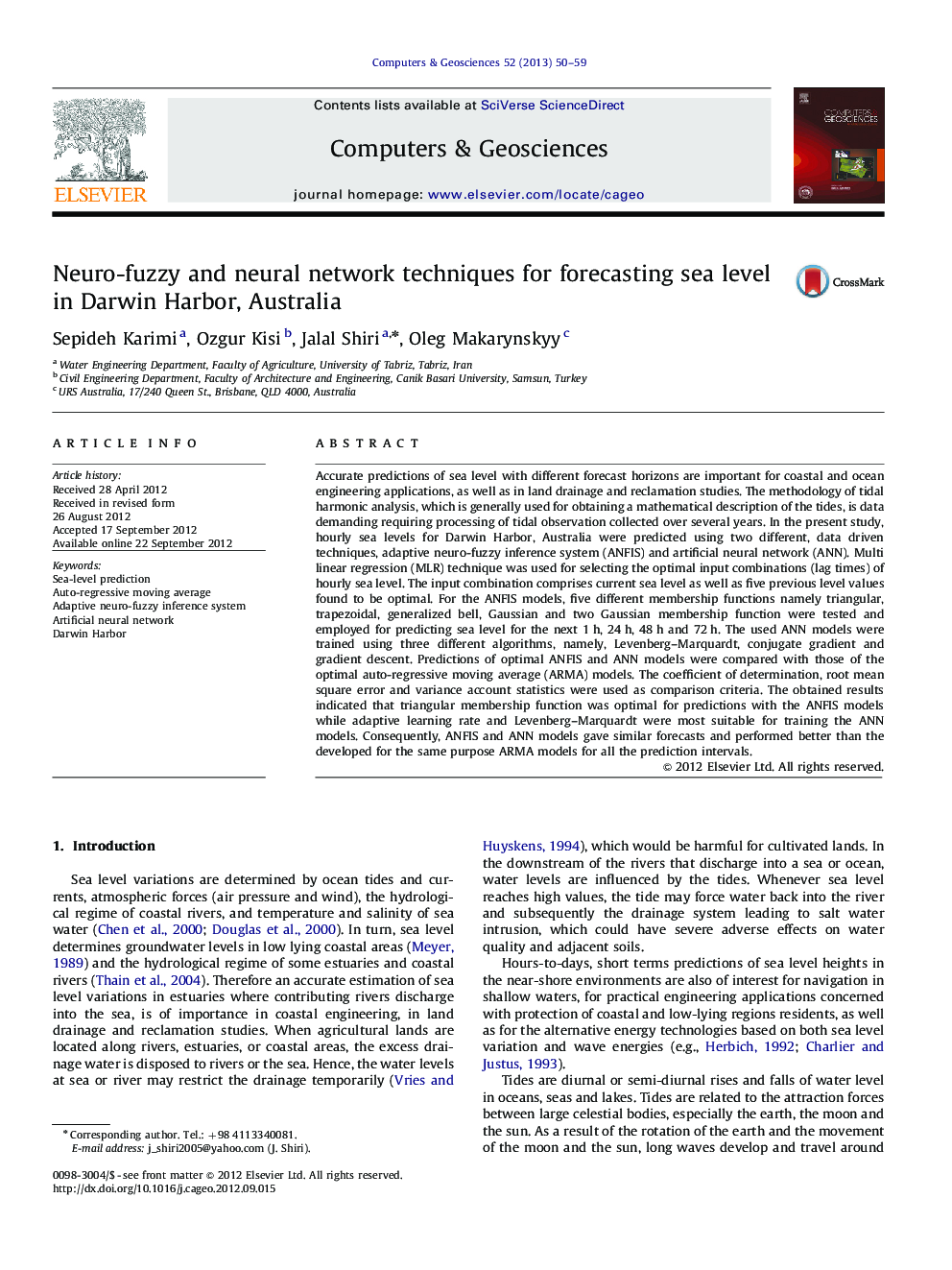
Accurate predictions of sea level with different forecast horizons are important for coastal and ocean engineering applications, as well as in land drainage and reclamation studies. The methodology of tidal harmonic analysis, which is generally used for obtaining a mathematical description of the tides, is data demanding requiring processing of tidal observation collected over several years. In the present study, hourly sea levels for Darwin Harbor, Australia were predicted using two different, data driven techniques, adaptive neuro-fuzzy inference system (ANFIS) and artificial neural network (ANN). Multi linear regression (MLR) technique was used for selecting the optimal input combinations (lag times) of hourly sea level. The input combination comprises current sea level as well as five previous level values found to be optimal. For the ANFIS models, five different membership functions namely triangular, trapezoidal, generalized bell, Gaussian and two Gaussian membership function were tested and employed for predicting sea level for the next 1 h, 24 h, 48 h and 72 h. The used ANN models were trained using three different algorithms, namely, Levenberg–Marquardt, conjugate gradient and gradient descent. Predictions of optimal ANFIS and ANN models were compared with those of the optimal auto-regressive moving average (ARMA) models. The coefficient of determination, root mean square error and variance account statistics were used as comparison criteria. The obtained results indicated that triangular membership function was optimal for predictions with the ANFIS models while adaptive learning rate and Levenberg–Marquardt were most suitable for training the ANN models. Consequently, ANFIS and ANN models gave similar forecasts and performed better than the developed for the same purpose ARMA models for all the prediction intervals.
► We model sea water level variations using neuro-fuzzy (NF) and neural networks (NN).
► The prediction intervals are hourly, daily, 2-daily and 3-daily horizons.
► The NF and NN are compared with auto regressive moving average (ARMA) model. NF and NN models show similar accuracy and they perform better than the ARMA.
Journal: Computers & Geosciences - Volume 52, March 2013, Pages 50–59