کد مقاله | کد نشریه | سال انتشار | مقاله انگلیسی | نسخه تمام متن |
---|---|---|---|---|
508047 | 865167 | 2012 | 10 صفحه PDF | دانلود رایگان |
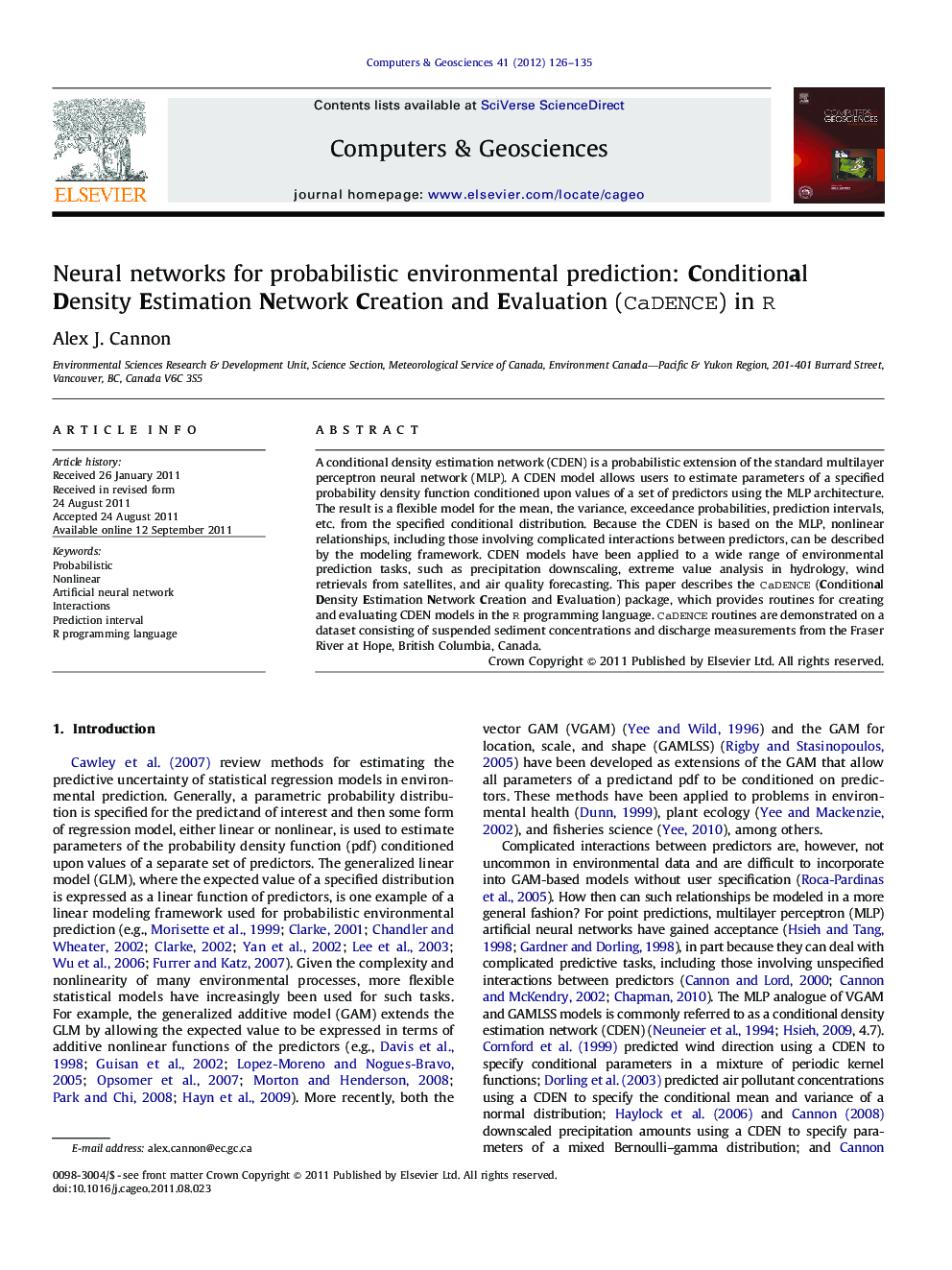
A conditional density estimation network (CDEN) is a probabilistic extension of the standard multilayer perceptron neural network (MLP). A CDEN model allows users to estimate parameters of a specified probability density function conditioned upon values of a set of predictors using the MLP architecture. The result is a flexible model for the mean, the variance, exceedance probabilities, prediction intervals, etc. from the specified conditional distribution. Because the CDEN is based on the MLP, nonlinear relationships, including those involving complicated interactions between predictors, can be described by the modeling framework. CDEN models have been applied to a wide range of environmental prediction tasks, such as precipitation downscaling, extreme value analysis in hydrology, wind retrievals from satellites, and air quality forecasting. This paper describes the CaDENCE (Conditional Density Estimation Network Creation and Evaluation) package, which provides routines for creating and evaluating CDEN models in the R programming language. CaDENCE routines are demonstrated on a dataset consisting of suspended sediment concentrations and discharge measurements from the Fraser River at Hope, British Columbia, Canada.
► A conditional density estimation network is developed for the R scripting language.
► Parameters of a predictand distribution can be conditioned on predictors.
► The model is nonlinear and can represent complicated relationships.
► Continuous, discrete, and mixed discrete-continuous distributions can be modeled.
► The method is demonstrated on a discharge–sediment rating curve dataset.
Journal: Computers & Geosciences - Volume 41, April 2012, Pages 126–135