کد مقاله | کد نشریه | سال انتشار | مقاله انگلیسی | نسخه تمام متن |
---|---|---|---|---|
5129004 | 1489607 | 2016 | 14 صفحه PDF | دانلود رایگان |
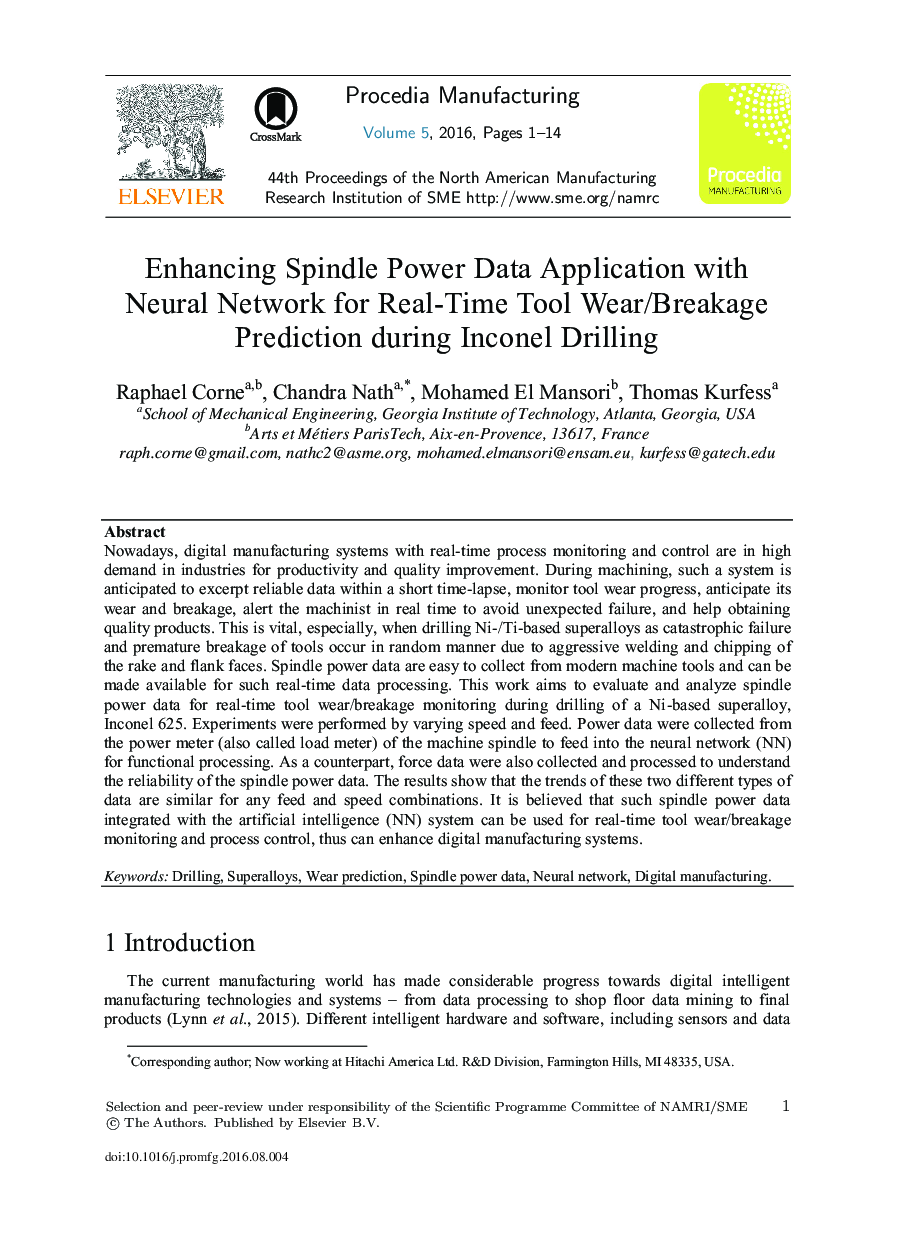
Nowadays, digital manufacturing systems with real-time process monitoring and control are in high demand in industries for productivity and quality improvement. During machining, such a system is anticipated to excerpt reliable data within a short time-lapse, monitor tool wear progress, anticipate its wear and breakage, alert the machinist in real time to avoid unexpected failure, and help obtaining quality products. This is vital, especially, when drilling Ni-/Ti-based superalloys as catastrophic failure and premature breakage of tools occur in random manner due to aggressive welding and chipping of the rake and flank faces. Spindle power data are easy to collect from modern machine tools and can be made available for such real-time data processing. This work aims to evaluate and analyze spindle power data for real-time tool wear/breakage monitoring during drilling of a Ni-based superalloy, Inconel 625. Experiments were performed by varying speed and feed. Power data were collected from the power meter (also called load meter) of the machine spindle to feed into the neural network (NN) for functional processing. As a counterpart, force data were also collected and processed to understand the reliability of the spindle power data. The results show that the trends of these two different types of data are similar for any feed and speed combinations. It is believed that such spindle power data integrated with the artificial intelligence (NN) system can be used for real-time tool wear/breakage monitoring and process control, thus can enhance digital manufacturing systems.
Journal: Procedia Manufacturing - Volume 5, 2016, Pages 1-14