کد مقاله | کد نشریه | سال انتشار | مقاله انگلیسی | نسخه تمام متن |
---|---|---|---|---|
5129413 | 1489643 | 2017 | 12 صفحه PDF | دانلود رایگان |
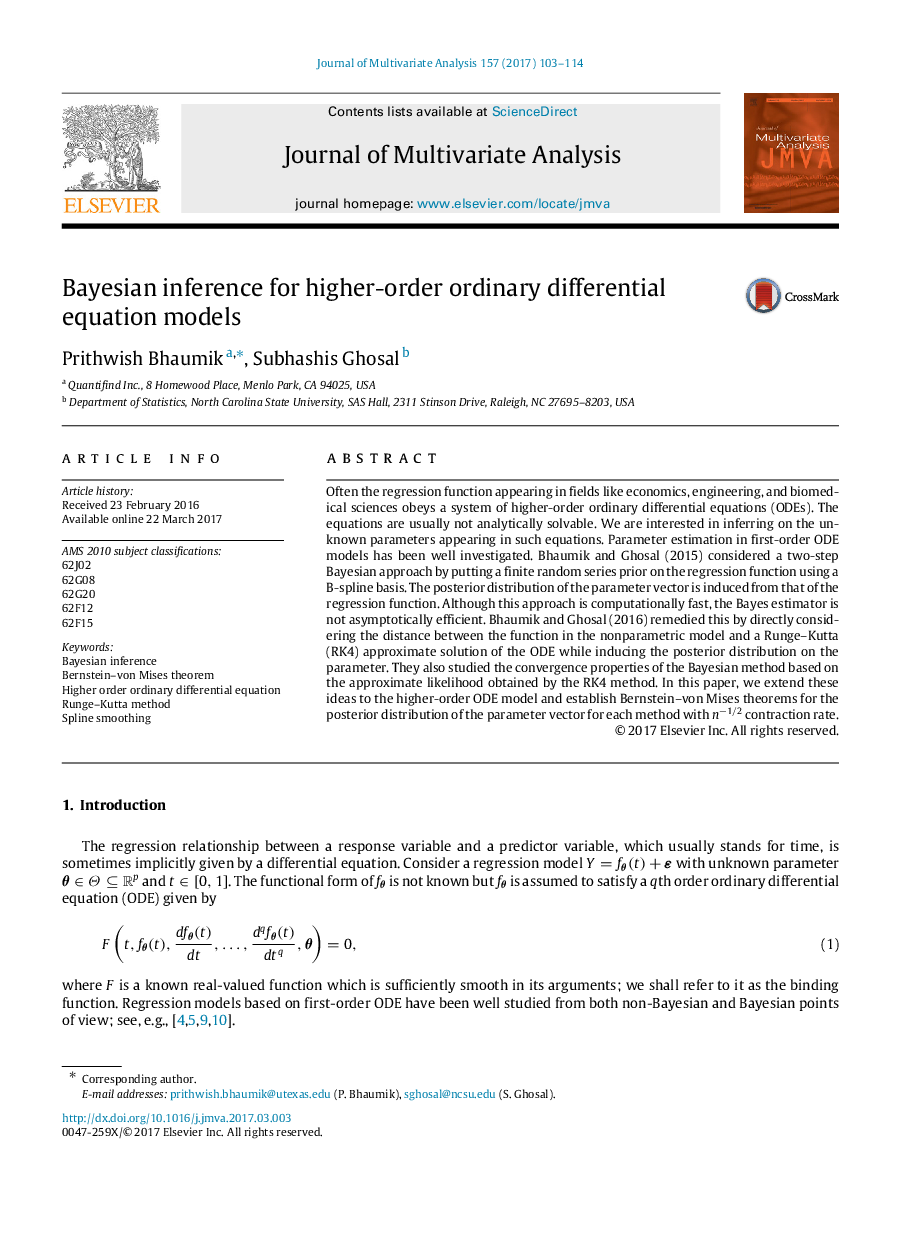
Often the regression function appearing in fields like economics, engineering, and biomedical sciences obeys a system of higher-order ordinary differential equations (ODEs). The equations are usually not analytically solvable. We are interested in inferring on the unknown parameters appearing in such equations. Parameter estimation in first-order ODE models has been well investigated. Bhaumik and Ghosal (2015) considered a two-step Bayesian approach by putting a finite random series prior on the regression function using a B-spline basis. The posterior distribution of the parameter vector is induced from that of the regression function. Although this approach is computationally fast, the Bayes estimator is not asymptotically efficient. Bhaumik and Ghosal (2016) remedied this by directly considering the distance between the function in the nonparametric model and a Runge-Kutta (RK4) approximate solution of the ODE while inducing the posterior distribution on the parameter. They also studied the convergence properties of the Bayesian method based on the approximate likelihood obtained by the RK4 method. In this paper, we extend these ideas to the higher-order ODE model and establish Bernstein-von Mises theorems for the posterior distribution of the parameter vector for each method with nâ1/2 contraction rate.
Journal: Journal of Multivariate Analysis - Volume 157, May 2017, Pages 103-114