کد مقاله | کد نشریه | سال انتشار | مقاله انگلیسی | نسخه تمام متن |
---|---|---|---|---|
5129531 | 1489738 | 2017 | 13 صفحه PDF | دانلود رایگان |
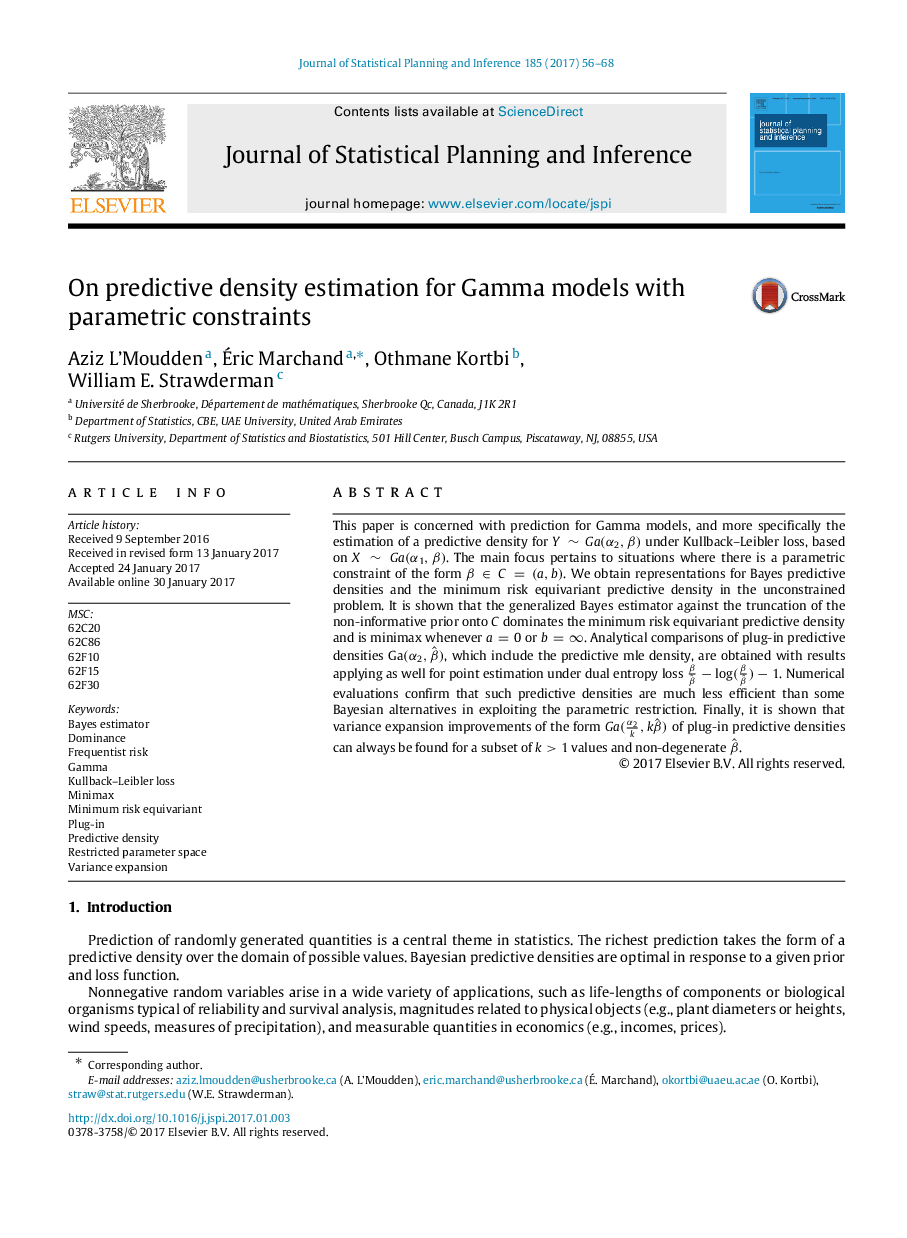
- Unified and general improvements on plug-in Gamma predictive densities.
- A general Kullback-Leibler dominance result for restricted parameter spaces.
- Further findings on minimaxity and on the performance of plug-in predictive densities.
This paper is concerned with prediction for Gamma models, and more specifically the estimation of a predictive density for Yâ¼Ga(α2,β) under Kullback-Leibler loss, based on Xâ¼Ga(α1,β). The main focus pertains to situations where there is a parametric constraint of the form βâC=(a,b). We obtain representations for Bayes predictive densities and the minimum risk equivariant predictive density in the unconstrained problem. It is shown that the generalized Bayes estimator against the truncation of the non-informative prior onto C dominates the minimum risk equivariant predictive density and is minimax whenever a=0 or b=â. Analytical comparisons of plug-in predictive densities Ga(α2,βË), which include the predictive mle density, are obtained with results applying as well for point estimation under dual entropy loss ββËâlog(ββË)â1. Numerical evaluations confirm that such predictive densities are much less efficient than some Bayesian alternatives in exploiting the parametric restriction. Finally, it is shown that variance expansion improvements of the form Ga(α2k,kβË) of plug-in predictive densities can always be found for a subset of k>1 values and non-degenerate βË.
Journal: Journal of Statistical Planning and Inference - Volume 185, June 2017, Pages 56-68