کد مقاله | کد نشریه | سال انتشار | مقاله انگلیسی | نسخه تمام متن |
---|---|---|---|---|
5139158 | 1494862 | 2017 | 27 صفحه PDF | دانلود رایگان |
عنوان انگلیسی مقاله ISI
Multivariate classification of disease phenotypes of esophageal adenocarcinoma by pattern recognition analysis of MALDI-TOF mass spectra of serum N-linked glycans
دانلود مقاله + سفارش ترجمه
دانلود مقاله ISI انگلیسی
رایگان برای ایرانیان
کلمات کلیدی
موضوعات مرتبط
مهندسی و علوم پایه
شیمی
شیمی آنالیزی یا شیمی تجزیه
پیش نمایش صفحه اول مقاله
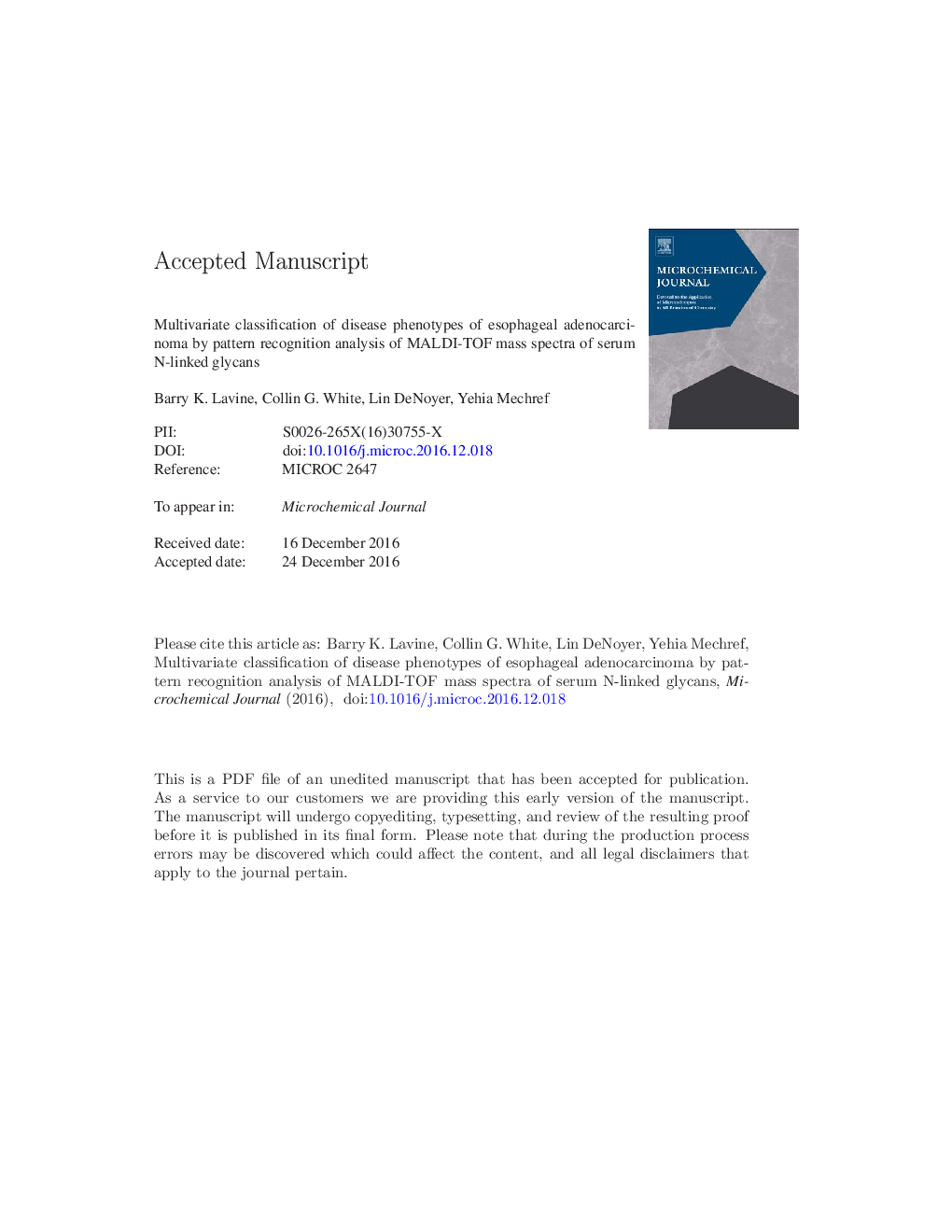
چکیده انگلیسی
The development of a novel two-step data analysis methodology to uncover signatures of potential cancer biomarkers in matrix assisted laser desorption ionization (MALDI) time of flight (TOF) mass spectra of large serum peptidomes is described. First, raw spectral data are processed using QceAlign, which exploits Bayesian and maximum entropy methods for peak identification and calibration. The raw spectral data are baseline corrected and normalized. Peak identification is based on a Bayesian second derivative of the baseline-corrected and normalized raw data, with peak S/N statistics provided by a maximum entropy smoothing function. A reference MALDI-TOF reference spectrum is created from the data and each spectrum is slid by n data points to the right or left along the x axis of the reference file. At each relative position n, the Shannon entropy of the sum of the two files is computed. Optimal alignment is associated with the shift that produces the minimum Shannon entropy. Second, a genetic algorithm (GA) for pattern recognition analysis is applied to the peak matched data. The pattern recognition GA selects features that optimize the separation of the sample classes in a plot of the two or three largest principal components of the data. Because the largest principal components capture the bulk of the variance in the data, the spectral features chosen by the pattern recognition GA convey information primarily about the differences between classes in the data. In addition, the algorithm focuses on those classes and or samples that are difficult to classify as it trains by boosting the sample and class weights. Samples that consistently classify correctly are not as heavily weighted as those samples that are difficult to classify. The pattern recognition GA integrates aspects of artificial intelligence and evolutionary computations to yield a “smart” one -pass procedure for features selection, classification, and prediction in a single step.
ناشر
Database: Elsevier - ScienceDirect (ساینس دایرکت)
Journal: Microchemical Journal - Volume 132, May 2017, Pages 83-88
Journal: Microchemical Journal - Volume 132, May 2017, Pages 83-88
نویسندگان
Barry K. Lavine, Collin G. White, Lin DeNoyer, Yehia Mechref,