کد مقاله | کد نشریه | سال انتشار | مقاله انگلیسی | نسخه تمام متن |
---|---|---|---|---|
514981 | 866931 | 2012 | 11 صفحه PDF | دانلود رایگان |
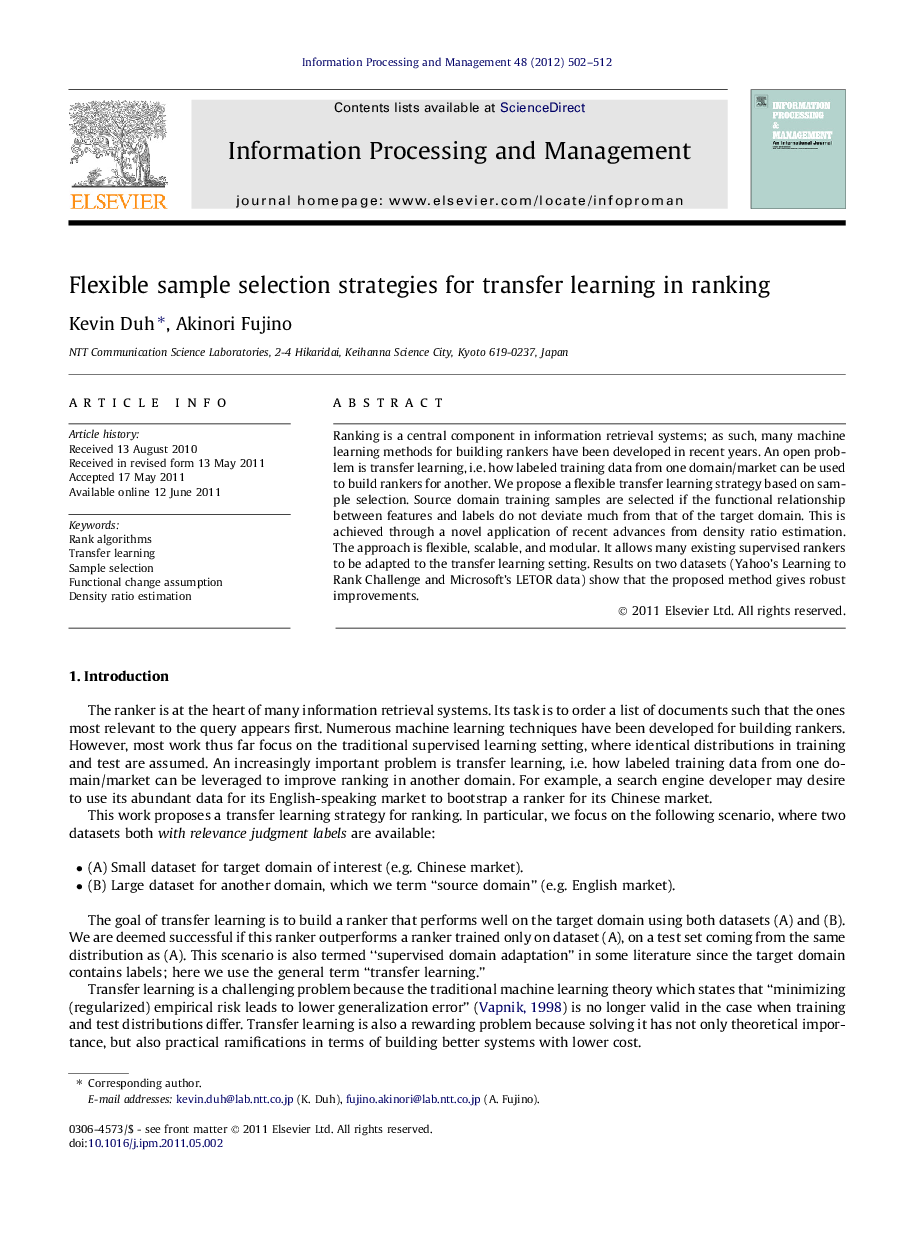
Ranking is a central component in information retrieval systems; as such, many machine learning methods for building rankers have been developed in recent years. An open problem is transfer learning, i.e. how labeled training data from one domain/market can be used to build rankers for another. We propose a flexible transfer learning strategy based on sample selection. Source domain training samples are selected if the functional relationship between features and labels do not deviate much from that of the target domain. This is achieved through a novel application of recent advances from density ratio estimation. The approach is flexible, scalable, and modular. It allows many existing supervised rankers to be adapted to the transfer learning setting. Results on two datasets (Yahoo’s Learning to Rank Challenge and Microsoft’s LETOR data) show that the proposed method gives robust improvements.
► Problem setting: Transfer learning for ranking.
► Approach: Sample selection of out-domain data with density ratio estimation.
► Novelty: Address the “Functional Change Assumption” in transfer learning.
► Result: Outperform conventional rankers on Yahoo & Microsoft public datasets.
Journal: Information Processing & Management - Volume 48, Issue 3, May 2012, Pages 502–512