کد مقاله | کد نشریه | سال انتشار | مقاله انگلیسی | نسخه تمام متن |
---|---|---|---|---|
515379 | 867002 | 2015 | 24 صفحه PDF | دانلود رایگان |
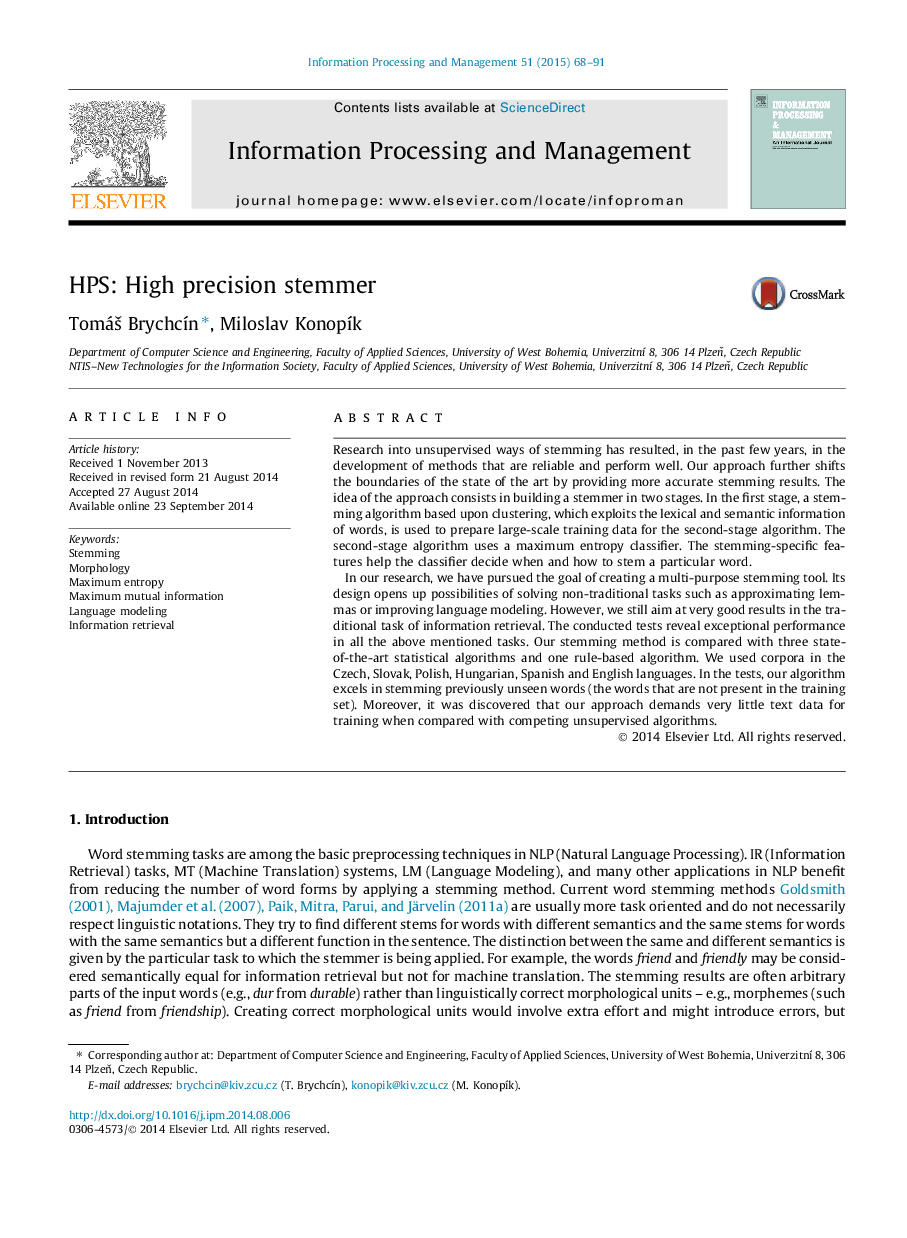
• New unsupervised stemming algorithm is introduced in this article.
• The algorithm exploits lexical as well as semantic information of words.
• Performance of stemming is measured on several languages (Czech, Slovak, Polish, Hungarian, Spanish and English).
• We outperform competing stemmers in inflection removal test, information retrieval task and language modeling task.
Research into unsupervised ways of stemming has resulted, in the past few years, in the development of methods that are reliable and perform well. Our approach further shifts the boundaries of the state of the art by providing more accurate stemming results. The idea of the approach consists in building a stemmer in two stages. In the first stage, a stemming algorithm based upon clustering, which exploits the lexical and semantic information of words, is used to prepare large-scale training data for the second-stage algorithm. The second-stage algorithm uses a maximum entropy classifier. The stemming-specific features help the classifier decide when and how to stem a particular word.In our research, we have pursued the goal of creating a multi-purpose stemming tool. Its design opens up possibilities of solving non-traditional tasks such as approximating lemmas or improving language modeling. However, we still aim at very good results in the traditional task of information retrieval. The conducted tests reveal exceptional performance in all the above mentioned tasks. Our stemming method is compared with three state-of-the-art statistical algorithms and one rule-based algorithm. We used corpora in the Czech, Slovak, Polish, Hungarian, Spanish and English languages. In the tests, our algorithm excels in stemming previously unseen words (the words that are not present in the training set). Moreover, it was discovered that our approach demands very little text data for training when compared with competing unsupervised algorithms.
Journal: Information Processing & Management - Volume 51, Issue 1, January 2015, Pages 68–91