کد مقاله | کد نشریه | سال انتشار | مقاله انگلیسی | نسخه تمام متن |
---|---|---|---|---|
516418 | 1449210 | 2007 | 14 صفحه PDF | دانلود رایگان |
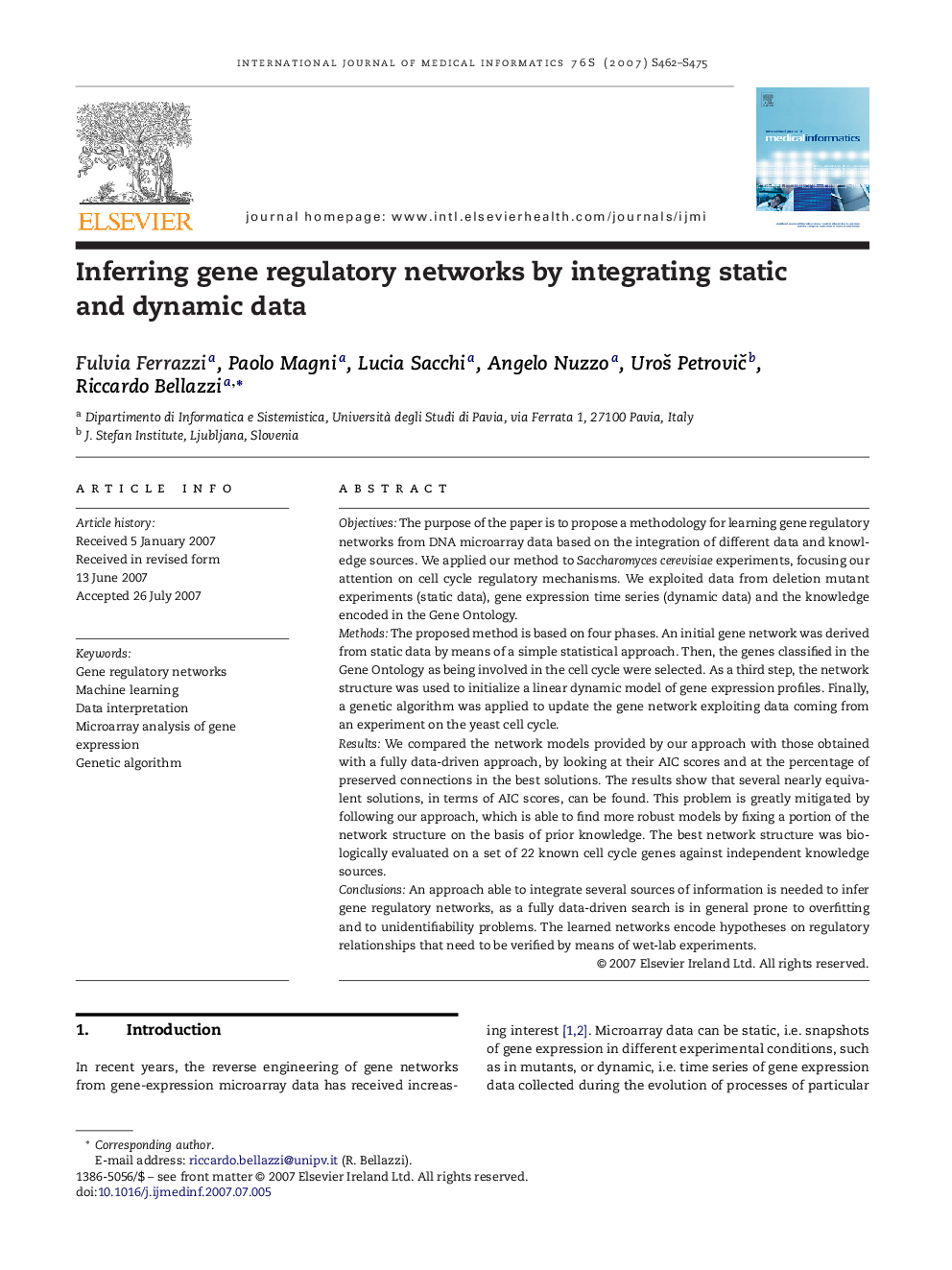
ObjectivesThe purpose of the paper is to propose a methodology for learning gene regulatory networks from DNA microarray data based on the integration of different data and knowledge sources. We applied our method to Saccharomyces cerevisiae experiments, focusing our attention on cell cycle regulatory mechanisms. We exploited data from deletion mutant experiments (static data), gene expression time series (dynamic data) and the knowledge encoded in the Gene Ontology.MethodsThe proposed method is based on four phases. An initial gene network was derived from static data by means of a simple statistical approach. Then, the genes classified in the Gene Ontology as being involved in the cell cycle were selected. As a third step, the network structure was used to initialize a linear dynamic model of gene expression profiles. Finally, a genetic algorithm was applied to update the gene network exploiting data coming from an experiment on the yeast cell cycle.ResultsWe compared the network models provided by our approach with those obtained with a fully data-driven approach, by looking at their AIC scores and at the percentage of preserved connections in the best solutions. The results show that several nearly equivalent solutions, in terms of AIC scores, can be found. This problem is greatly mitigated by following our approach, which is able to find more robust models by fixing a portion of the network structure on the basis of prior knowledge. The best network structure was biologically evaluated on a set of 22 known cell cycle genes against independent knowledge sources.ConclusionsAn approach able to integrate several sources of information is needed to infer gene regulatory networks, as a fully data-driven search is in general prone to overfitting and to unidentifiability problems. The learned networks encode hypotheses on regulatory relationships that need to be verified by means of wet-lab experiments.
Journal: International Journal of Medical Informatics - Volume 76, Supplement 3, December 2007, Pages S462–S475