کد مقاله | کد نشریه | سال انتشار | مقاله انگلیسی | نسخه تمام متن |
---|---|---|---|---|
517485 | 867457 | 2009 | 12 صفحه PDF | دانلود رایگان |
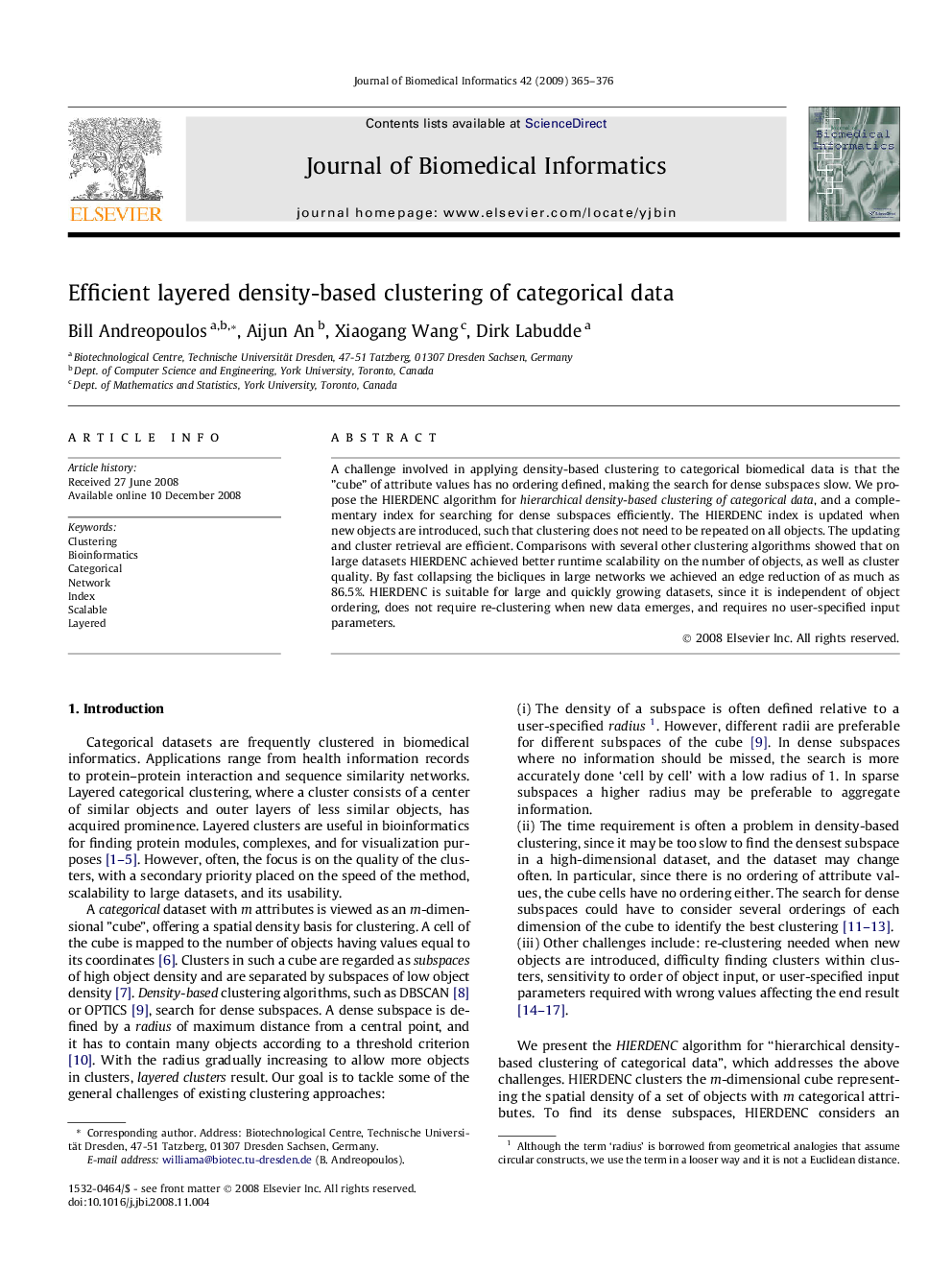
A challenge involved in applying density-based clustering to categorical biomedical data is that the ”cube” of attribute values has no ordering defined, making the search for dense subspaces slow. We propose the HIERDENC algorithm for hierarchical density-based clustering of categorical data, and a complementary index for searching for dense subspaces efficiently. The HIERDENC index is updated when new objects are introduced, such that clustering does not need to be repeated on all objects. The updating and cluster retrieval are efficient. Comparisons with several other clustering algorithms showed that on large datasets HIERDENC achieved better runtime scalability on the number of objects, as well as cluster quality. By fast collapsing the bicliques in large networks we achieved an edge reduction of as much as 86.5%. HIERDENC is suitable for large and quickly growing datasets, since it is independent of object ordering, does not require re-clustering when new data emerges, and requires no user-specified input parameters.
Journal: Journal of Biomedical Informatics - Volume 42, Issue 2, April 2009, Pages 365–376