کد مقاله | کد نشریه | سال انتشار | مقاله انگلیسی | نسخه تمام متن |
---|---|---|---|---|
526814 | 869235 | 2015 | 10 صفحه PDF | دانلود رایگان |
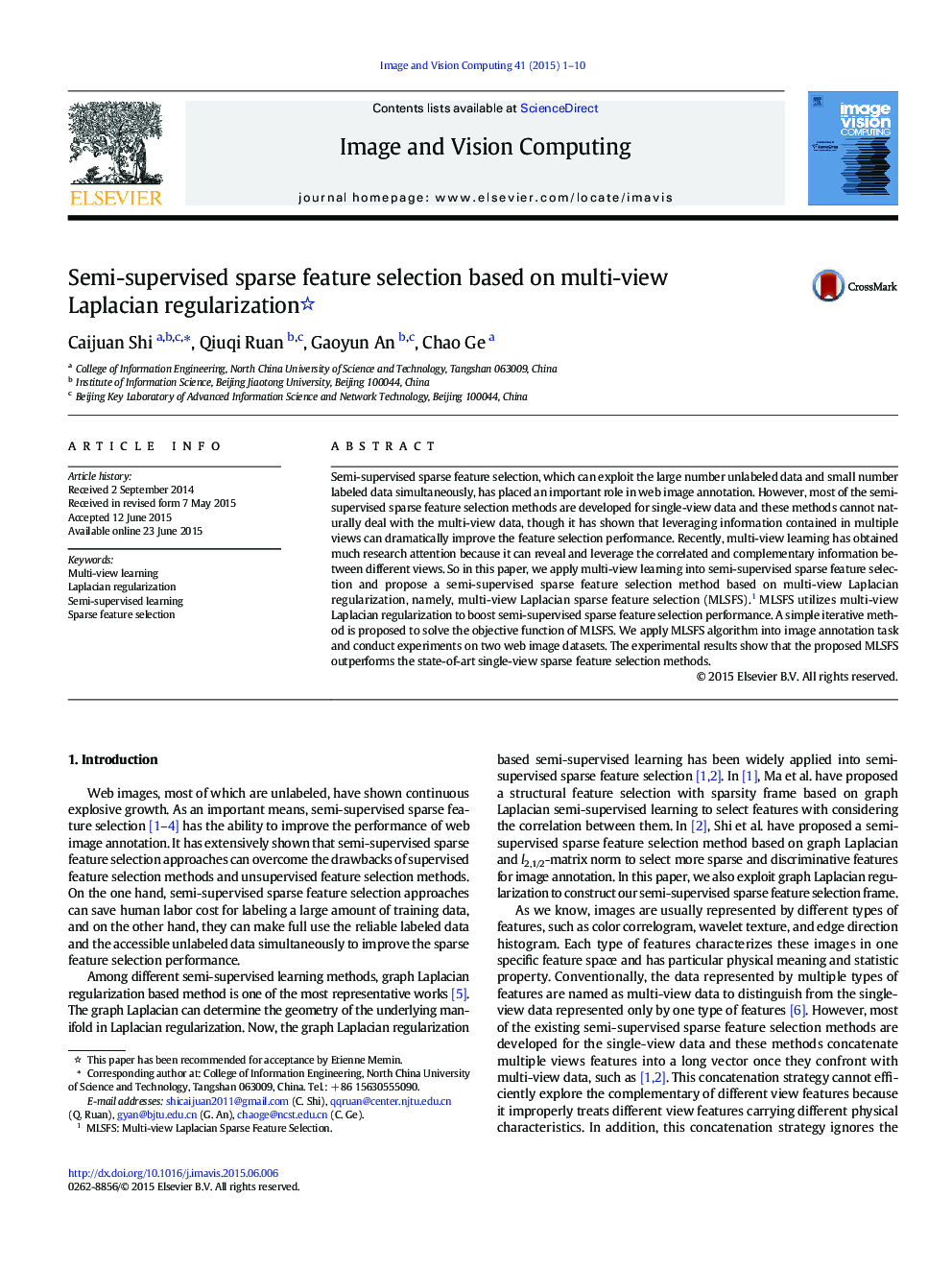
• Multi-view Laplacian sparse feature selection (MLSFS) algorithm is proposed.
• Multi-view learning is utilized to exploit the complementation of different views features.
• A effective iterative algorithm is introduced to optimize the objective function.
• The convergence of the algorithm is proven.
• Experiments demonstrate MLSFS has good performance of feature selection.
Semi-supervised sparse feature selection, which can exploit the large number unlabeled data and small number labeled data simultaneously, has placed an important role in web image annotation. However, most of the semi-supervised sparse feature selection methods are developed for single-view data and these methods cannot naturally deal with the multi-view data, though it has shown that leveraging information contained in multiple views can dramatically improve the feature selection performance. Recently, multi-view learning has obtained much research attention because it can reveal and leverage the correlated and complementary information between different views. So in this paper, we apply multi-view learning into semi-supervised sparse feature selection and propose a semi-supervised sparse feature selection method based on multi-view Laplacian regularization, namely, multi-view Laplacian sparse feature selection (MLSFS).1 MLSFS utilizes multi-view Laplacian regularization to boost semi-supervised sparse feature selection performance. A simple iterative method is proposed to solve the objective function of MLSFS. We apply MLSFS algorithm into image annotation task and conduct experiments on two web image datasets. The experimental results show that the proposed MLSFS outperforms the state-of-art single-view sparse feature selection methods.
Figure optionsDownload high-quality image (144 K)Download as PowerPoint slide
Journal: Image and Vision Computing - Volume 41, September 2015, Pages 1–10