کد مقاله | کد نشریه | سال انتشار | مقاله انگلیسی | نسخه تمام متن |
---|---|---|---|---|
527003 | 869271 | 2015 | 15 صفحه PDF | دانلود رایگان |
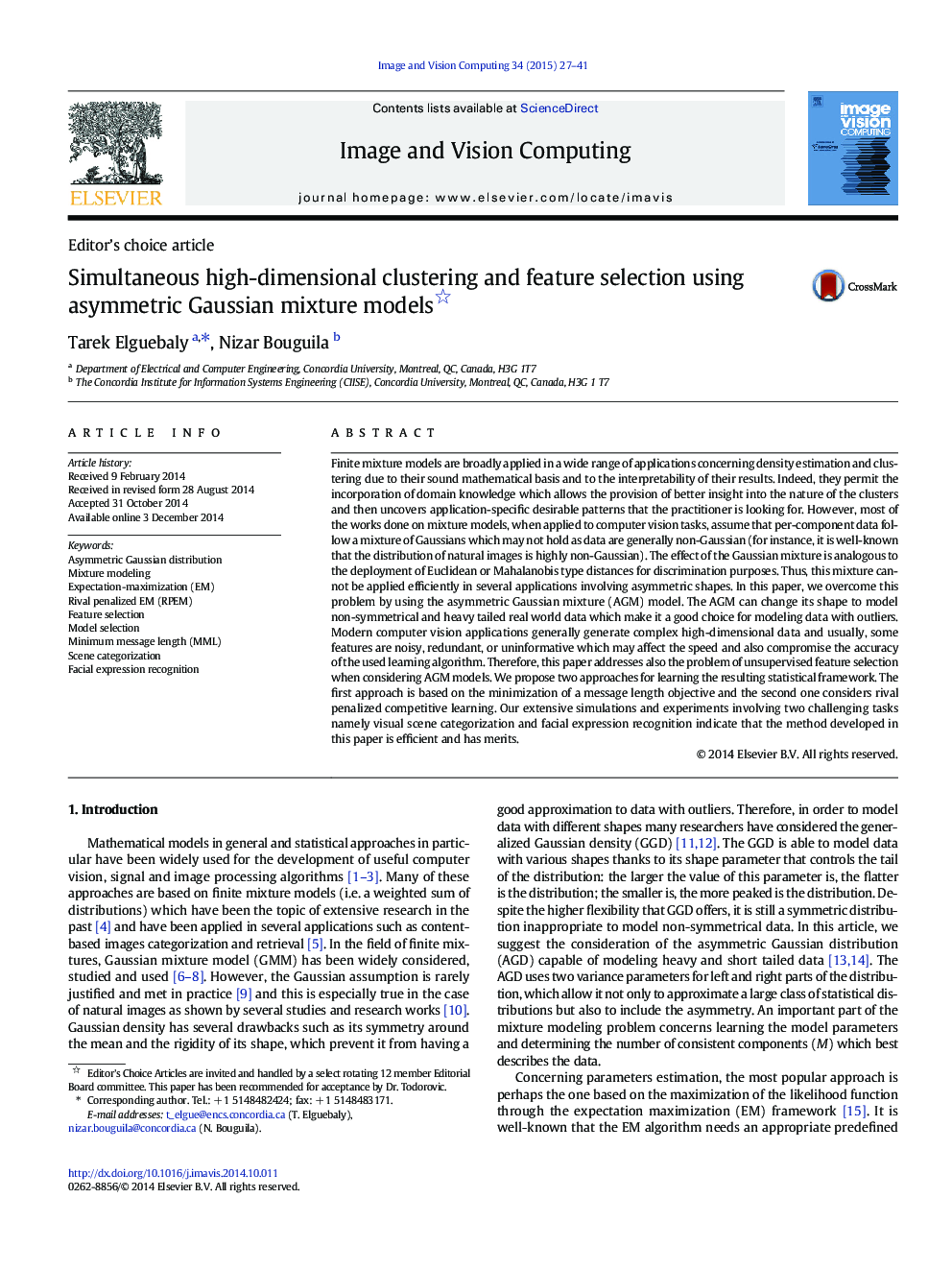
• We introduce the multidimensional asymmetric Gaussian mixture (AGGM).
• We propose two novel inference frameworks for unsupervised non-Gaussian feature selection.
• We have used these frameworks for challenging applications.
Finite mixture models are broadly applied in a wide range of applications concerning density estimation and clustering due to their sound mathematical basis and to the interpretability of their results. Indeed, they permit the incorporation of domain knowledge which allows the provision of better insight into the nature of the clusters and then uncovers application-specific desirable patterns that the practitioner is looking for. However, most of the works done on mixture models, when applied to computer vision tasks, assume that per-component data follow a mixture of Gaussians which may not hold as data are generally non-Gaussian (for instance, it is well-known that the distribution of natural images is highly non-Gaussian). The effect of the Gaussian mixture is analogous to the deployment of Euclidean or Mahalanobis type distances for discrimination purposes. Thus, this mixture cannot be applied efficiently in several applications involving asymmetric shapes. In this paper, we overcome this problem by using the asymmetric Gaussian mixture (AGM) model. The AGM can change its shape to model non-symmetrical and heavy tailed real world data which make it a good choice for modeling data with outliers. Modern computer vision applications generally generate complex high-dimensional data and usually, some features are noisy, redundant, or uninformative which may affect the speed and also compromise the accuracy of the used learning algorithm. Therefore, this paper addresses also the problem of unsupervised feature selection when considering AGM models. We propose two approaches for learning the resulting statistical framework. The first approach is based on the minimization of a message length objective and the second one considers rival penalized competitive learning. Our extensive simulations and experiments involving two challenging tasks namely visual scene categorization and facial expression recognition indicate that the method developed in this paper is efficient and has merits.
Journal: Image and Vision Computing - Volume 34, February 2015, Pages 27–41