کد مقاله | کد نشریه | سال انتشار | مقاله انگلیسی | نسخه تمام متن |
---|---|---|---|---|
528413 | 869566 | 2014 | 12 صفحه PDF | دانلود رایگان |
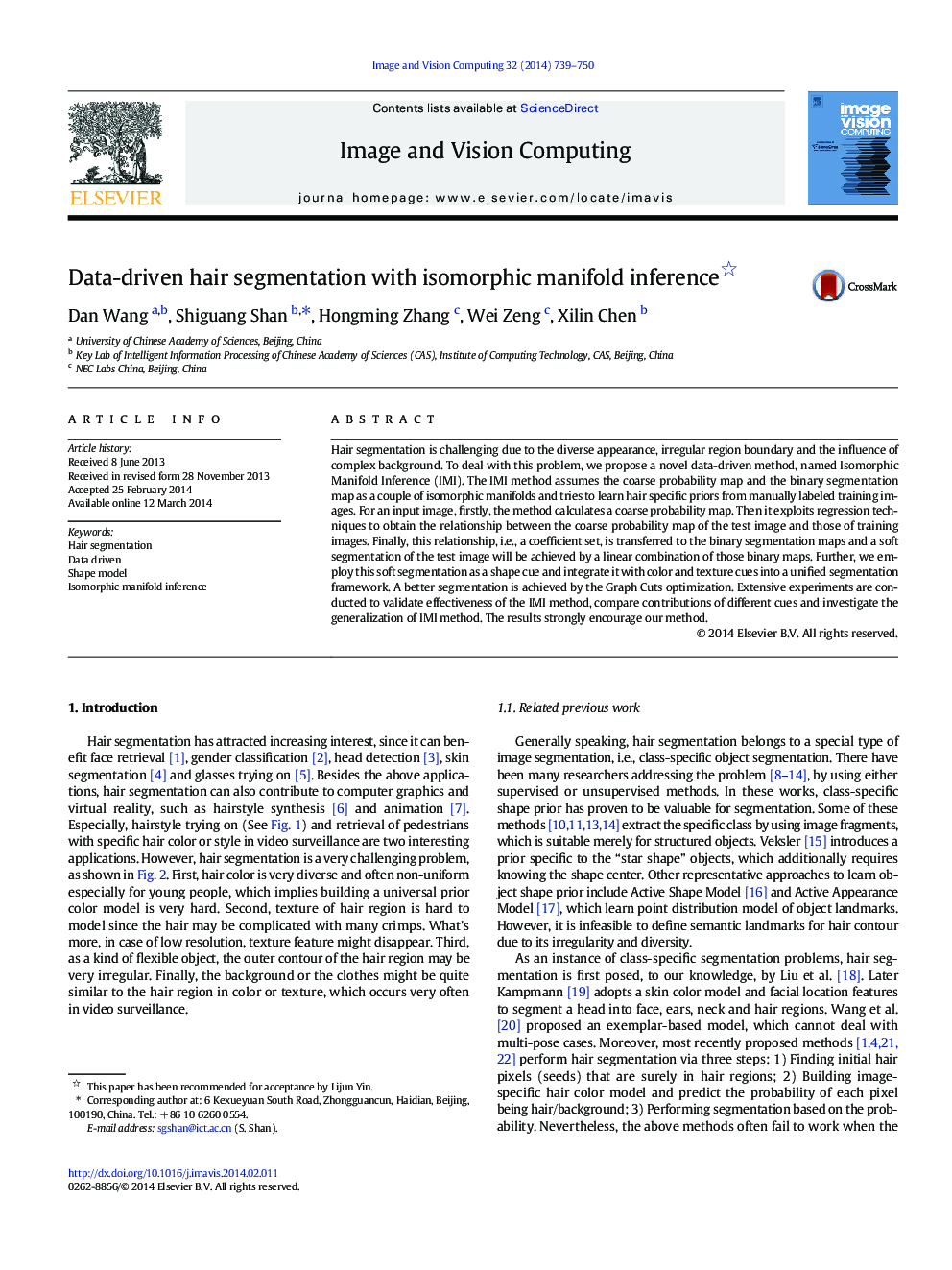
• Similar coarse hair probability maps should correspond to similar segmentations.
• Data-driven Isomorphic Manifold Inference is proposed to exploit the shape priors.
• We integrate the inferred shape, color and texture into a unified framework.
• Besides hair segmentation, we also validate our IMI on horse class segmentation.
• Experiments on hair and horse databases show impressive performances.
Hair segmentation is challenging due to the diverse appearance, irregular region boundary and the influence of complex background. To deal with this problem, we propose a novel data-driven method, named Isomorphic Manifold Inference (IMI). The IMI method assumes the coarse probability map and the binary segmentation map as a couple of isomorphic manifolds and tries to learn hair specific priors from manually labeled training images. For an input image, firstly, the method calculates a coarse probability map. Then it exploits regression techniques to obtain the relationship between the coarse probability map of the test image and those of training images. Finally, this relationship, i.e., a coefficient set, is transferred to the binary segmentation maps and a soft segmentation of the test image will be achieved by a linear combination of those binary maps. Further, we employ this soft segmentation as a shape cue and integrate it with color and texture cues into a unified segmentation framework. A better segmentation is achieved by the Graph Cuts optimization. Extensive experiments are conducted to validate effectiveness of the IMI method, compare contributions of different cues and investigate the generalization of IMI method. The results strongly encourage our method.
Journal: Image and Vision Computing - Volume 32, Issue 10, October 2014, Pages 739–750