کد مقاله | کد نشریه | سال انتشار | مقاله انگلیسی | نسخه تمام متن |
---|---|---|---|---|
529090 | 869629 | 2010 | 17 صفحه PDF | دانلود رایگان |
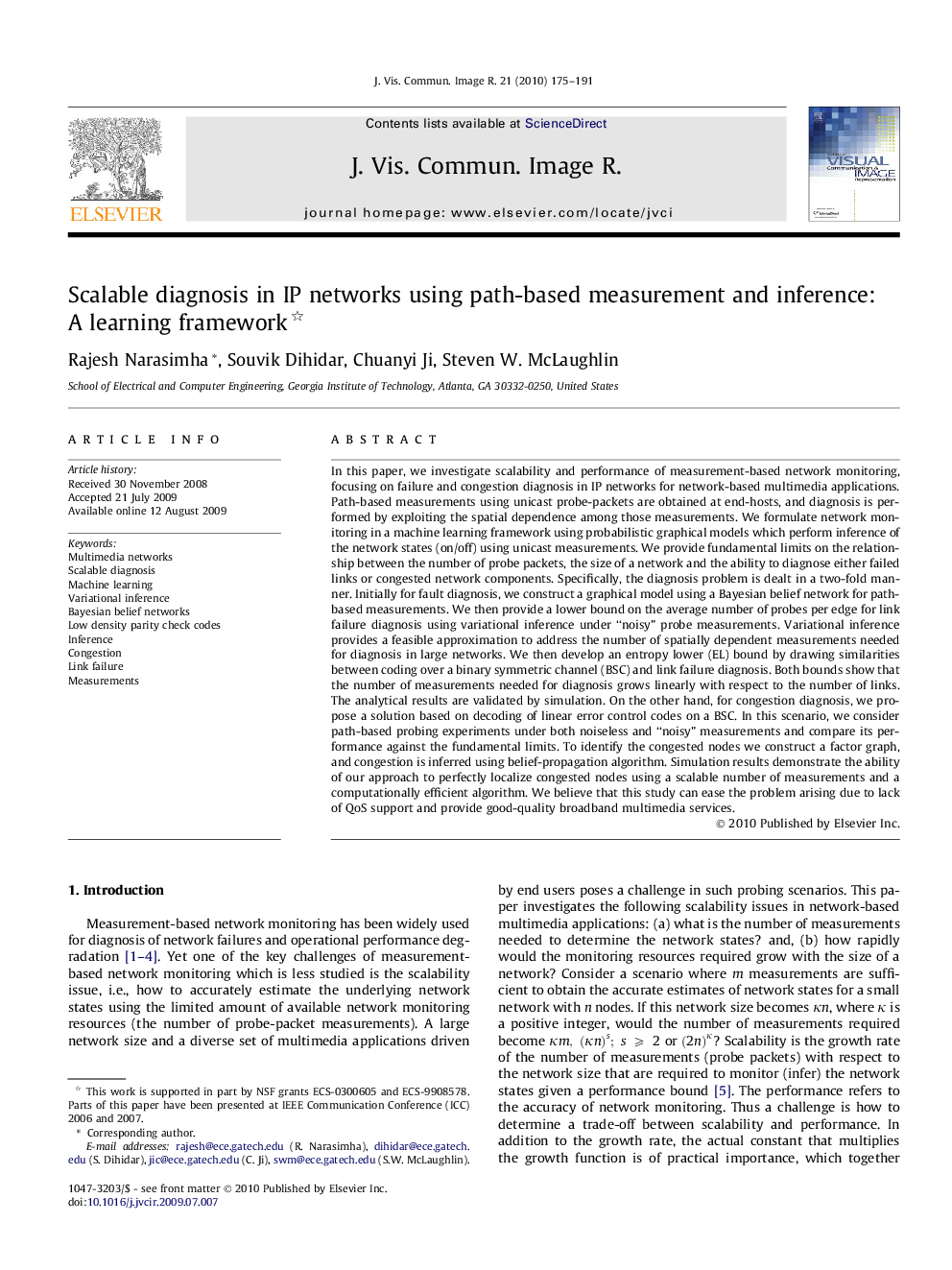
In this paper, we investigate scalability and performance of measurement-based network monitoring, focusing on failure and congestion diagnosis in IP networks for network-based multimedia applications. Path-based measurements using unicast probe-packets are obtained at end-hosts, and diagnosis is performed by exploiting the spatial dependence among those measurements. We formulate network monitoring in a machine learning framework using probabilistic graphical models which perform inference of the network states (on/off) using unicast measurements. We provide fundamental limits on the relationship between the number of probe packets, the size of a network and the ability to diagnose either failed links or congested network components. Specifically, the diagnosis problem is dealt in a two-fold manner. Initially for fault diagnosis, we construct a graphical model using a Bayesian belief network for path-based measurements. We then provide a lower bound on the average number of probes per edge for link failure diagnosis using variational inference under “noisy” probe measurements. Variational inference provides a feasible approximation to address the number of spatially dependent measurements needed for diagnosis in large networks. We then develop an entropy lower (EL) bound by drawing similarities between coding over a binary symmetric channel (BSC) and link failure diagnosis. Both bounds show that the number of measurements needed for diagnosis grows linearly with respect to the number of links. The analytical results are validated by simulation. On the other hand, for congestion diagnosis, we propose a solution based on decoding of linear error control codes on a BSC. In this scenario, we consider path-based probing experiments under both noiseless and “noisy” measurements and compare its performance against the fundamental limits. To identify the congested nodes we construct a factor graph, and congestion is inferred using belief-propagation algorithm. Simulation results demonstrate the ability of our approach to perfectly localize congested nodes using a scalable number of measurements and a computationally efficient algorithm. We believe that this study can ease the problem arising due to lack of QoS support and provide good-quality broadband multimedia services.
Journal: Journal of Visual Communication and Image Representation - Volume 21, Issue 2, February 2010, Pages 175–191