کد مقاله | کد نشریه | سال انتشار | مقاله انگلیسی | نسخه تمام متن |
---|---|---|---|---|
530014 | 869729 | 2015 | 12 صفحه PDF | دانلود رایگان |
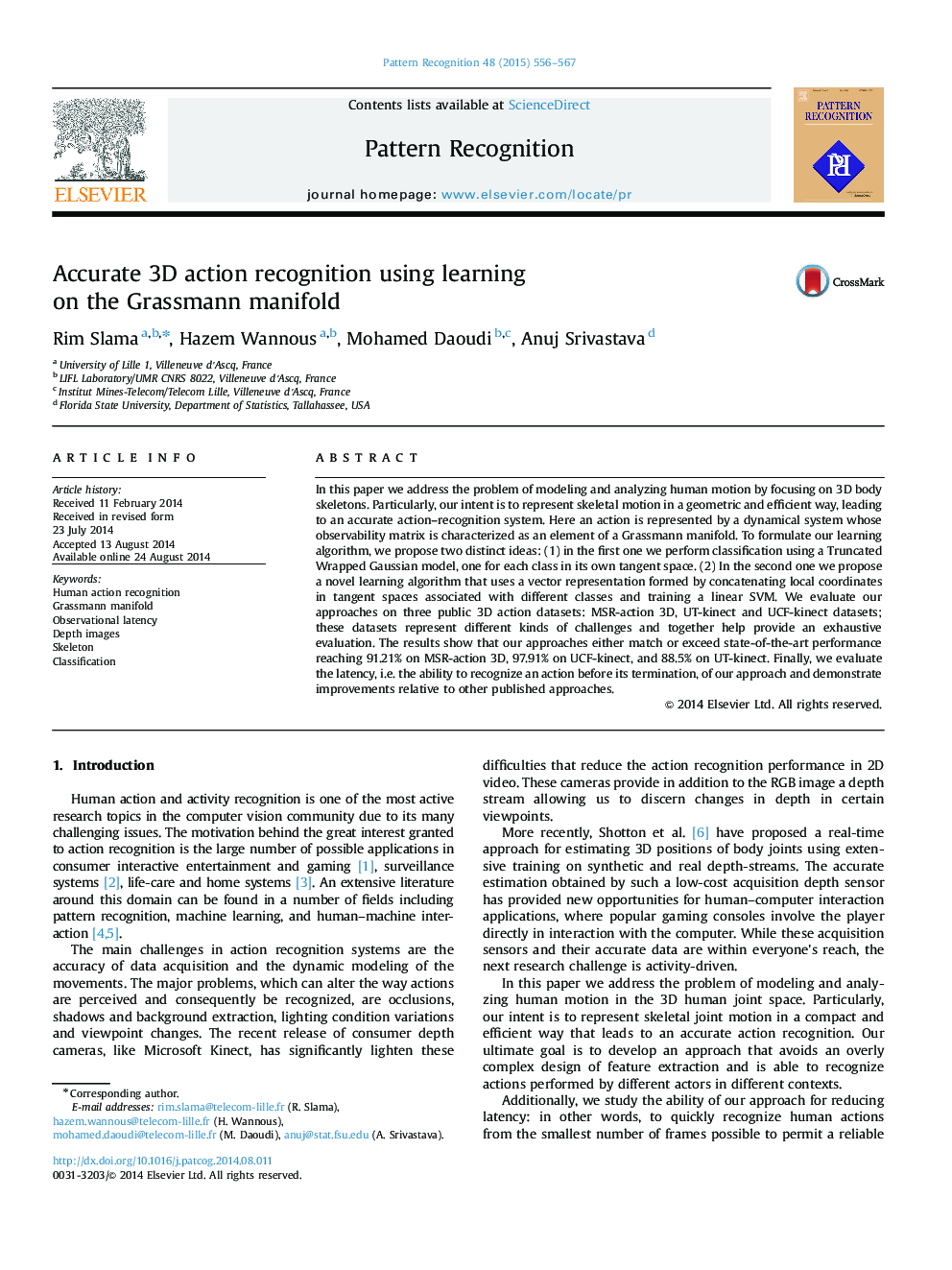
• A human action recognition approach which represents skeletal sequence as point on the Grassmann manifold.
• A new learning algorithm is introduced for learning human actions.
• Experiments are performed on three public datasets.
• Promising success rates are achieved, showing accuracy and better latency performances.
In this paper we address the problem of modeling and analyzing human motion by focusing on 3D body skeletons. Particularly, our intent is to represent skeletal motion in a geometric and efficient way, leading to an accurate action–recognition system. Here an action is represented by a dynamical system whose observability matrix is characterized as an element of a Grassmann manifold. To formulate our learning algorithm, we propose two distinct ideas: (1) in the first one we perform classification using a Truncated Wrapped Gaussian model, one for each class in its own tangent space. (2) In the second one we propose a novel learning algorithm that uses a vector representation formed by concatenating local coordinates in tangent spaces associated with different classes and training a linear SVM. We evaluate our approaches on three public 3D action datasets: MSR-action 3D, UT-kinect and UCF-kinect datasets; these datasets represent different kinds of challenges and together help provide an exhaustive evaluation. The results show that our approaches either match or exceed state-of-the-art performance reaching 91.21% on MSR-action 3D, 97.91% on UCF-kinect, and 88.5% on UT-kinect. Finally, we evaluate the latency, i.e. the ability to recognize an action before its termination, of our approach and demonstrate improvements relative to other published approaches.
Journal: Pattern Recognition - Volume 48, Issue 2, February 2015, Pages 556–567