کد مقاله | کد نشریه | سال انتشار | مقاله انگلیسی | نسخه تمام متن |
---|---|---|---|---|
530284 | 869755 | 2015 | 12 صفحه PDF | دانلود رایگان |
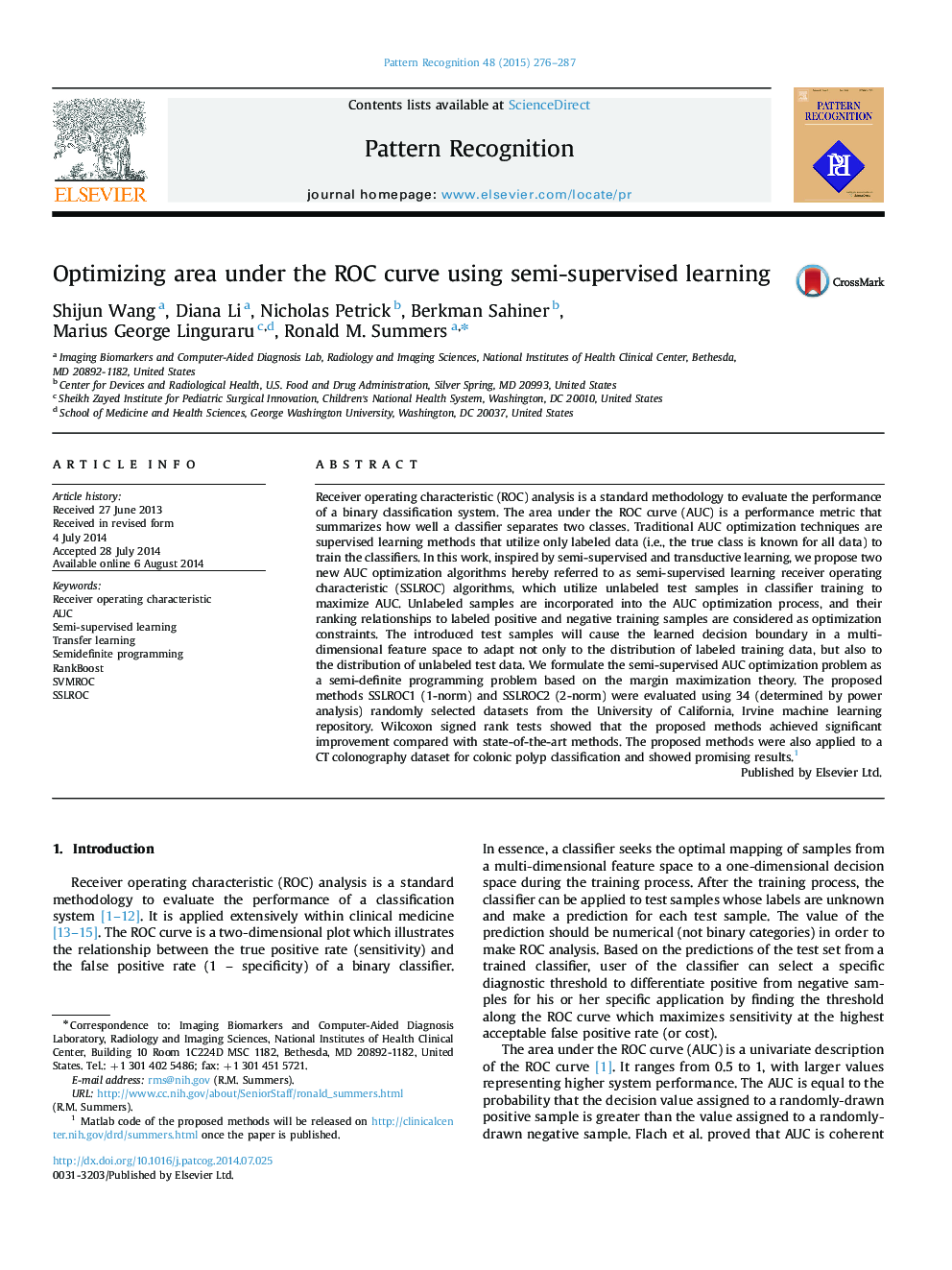
• Optimizing area under the ROC curve using semi-supervised learning.
• A large margin maximization semi-supervised learning framework for AUC maximization.
• Closed-form solution based on semi-definite programming.
• Superior performance on 34 UCI machine learning datasets determined by power analysis.
• Showed efficacy on a CT colonography dataset for colonic polyp classification.
Receiver operating characteristic (ROC) analysis is a standard methodology to evaluate the performance of a binary classification system. The area under the ROC curve (AUC) is a performance metric that summarizes how well a classifier separates two classes. Traditional AUC optimization techniques are supervised learning methods that utilize only labeled data (i.e., the true class is known for all data) to train the classifiers. In this work, inspired by semi-supervised and transductive learning, we propose two new AUC optimization algorithms hereby referred to as semi-supervised learning receiver operating characteristic (SSLROC) algorithms, which utilize unlabeled test samples in classifier training to maximize AUC. Unlabeled samples are incorporated into the AUC optimization process, and their ranking relationships to labeled positive and negative training samples are considered as optimization constraints. The introduced test samples will cause the learned decision boundary in a multi-dimensional feature space to adapt not only to the distribution of labeled training data, but also to the distribution of unlabeled test data. We formulate the semi-supervised AUC optimization problem as a semi-definite programming problem based on the margin maximization theory. The proposed methods SSLROC1 (1-norm) and SSLROC2 (2-norm) were evaluated using 34 (determined by power analysis) randomly selected datasets from the University of California, Irvine machine learning repository. Wilcoxon signed rank tests showed that the proposed methods achieved significant improvement compared with state-of-the-art methods. The proposed methods were also applied to a CT colonography dataset for colonic polyp classification and showed promising results.1
Journal: Pattern Recognition - Volume 48, Issue 1, January 2015, Pages 276–287