کد مقاله | کد نشریه | سال انتشار | مقاله انگلیسی | نسخه تمام متن |
---|---|---|---|---|
530320 | 869756 | 2012 | 13 صفحه PDF | دانلود رایگان |
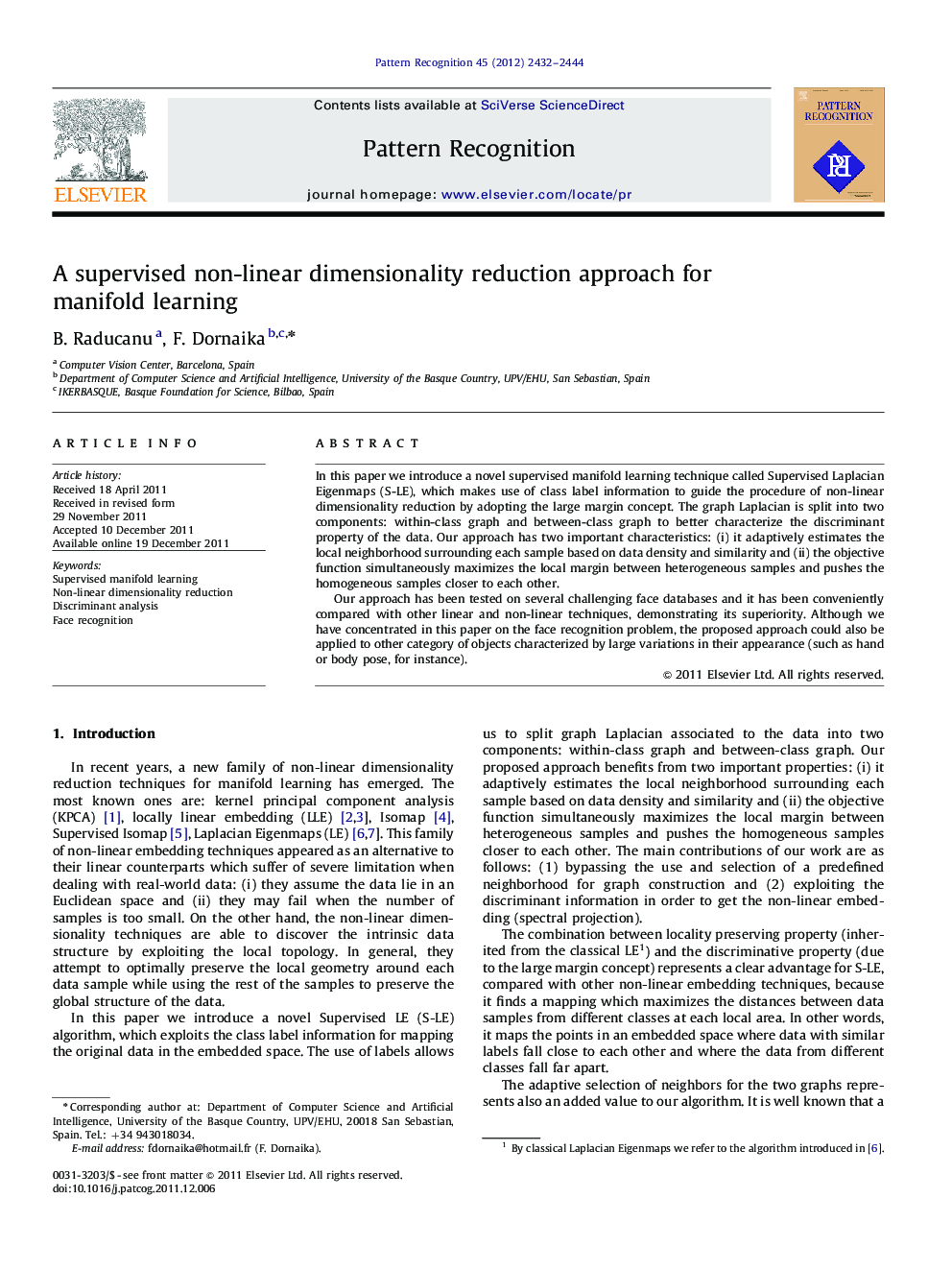
In this paper we introduce a novel supervised manifold learning technique called Supervised Laplacian Eigenmaps (S-LE), which makes use of class label information to guide the procedure of non-linear dimensionality reduction by adopting the large margin concept. The graph Laplacian is split into two components: within-class graph and between-class graph to better characterize the discriminant property of the data. Our approach has two important characteristics: (i) it adaptively estimates the local neighborhood surrounding each sample based on data density and similarity and (ii) the objective function simultaneously maximizes the local margin between heterogeneous samples and pushes the homogeneous samples closer to each other.Our approach has been tested on several challenging face databases and it has been conveniently compared with other linear and non-linear techniques, demonstrating its superiority. Although we have concentrated in this paper on the face recognition problem, the proposed approach could also be applied to other category of objects characterized by large variations in their appearance (such as hand or body pose, for instance).
► We propose a novel supervised non-linear dimensionality reduction (DR) approach that adopts the large margin concept.
► The approach adaptively estimates the local neighborhood surrounding each sample based on local density and similarity.
► It maximizes the local margin between heterogeneous samples and pushes the homogeneous samples closer to each other.
► For validation purposes, we applied our method to the face recognition problem.
► Results obtained on six face data sets show that our approach outperforms many recent linear and non-linear DR techniques.
Journal: Pattern Recognition - Volume 45, Issue 6, June 2012, Pages 2432–2444