کد مقاله | کد نشریه | سال انتشار | مقاله انگلیسی | نسخه تمام متن |
---|---|---|---|---|
530612 | 869779 | 2013 | 14 صفحه PDF | دانلود رایگان |
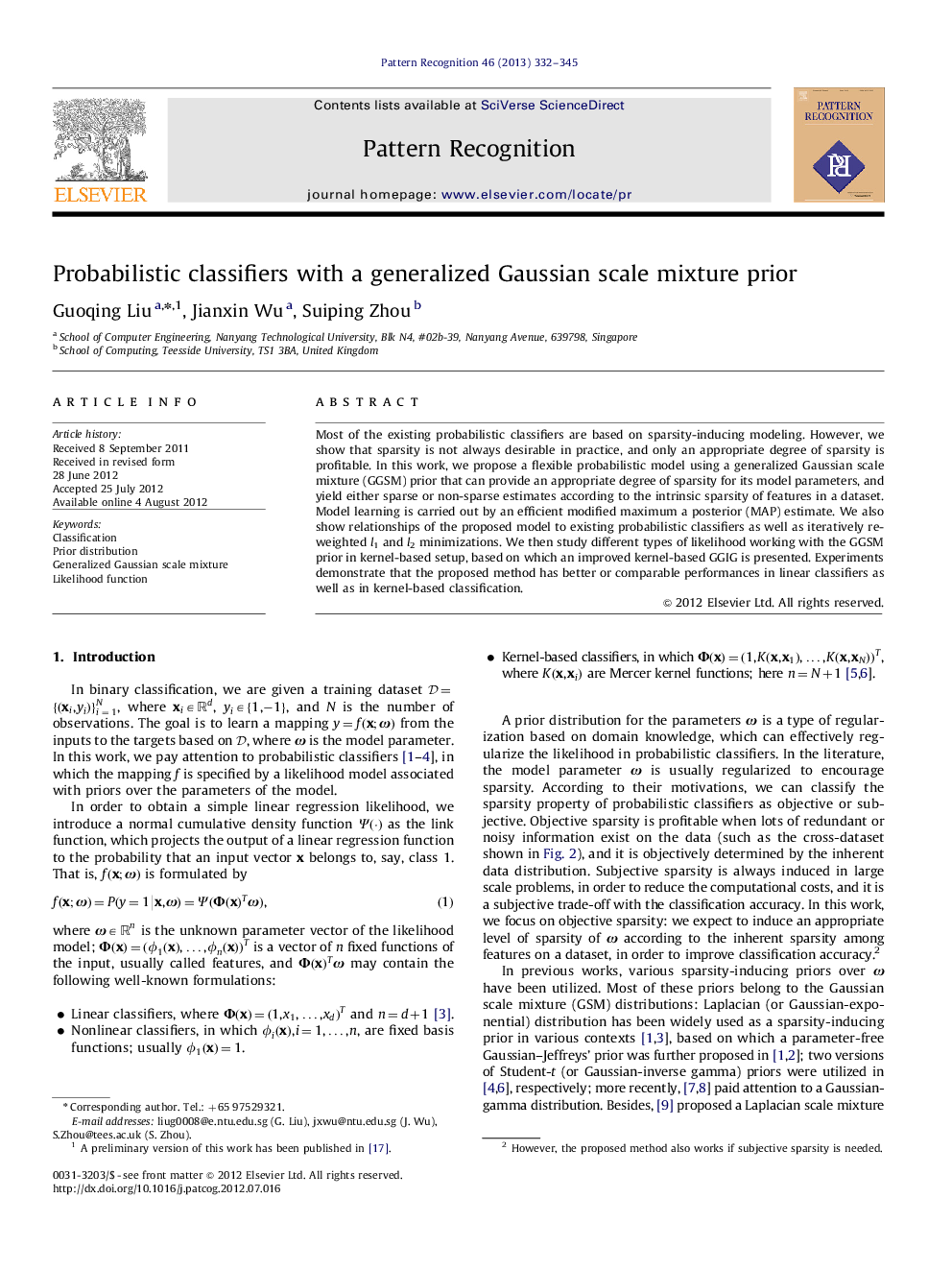
Most of the existing probabilistic classifiers are based on sparsity-inducing modeling. However, we show that sparsity is not always desirable in practice, and only an appropriate degree of sparsity is profitable. In this work, we propose a flexible probabilistic model using a generalized Gaussian scale mixture (GGSM) prior that can provide an appropriate degree of sparsity for its model parameters, and yield either sparse or non-sparse estimates according to the intrinsic sparsity of features in a dataset. Model learning is carried out by an efficient modified maximum a posterior (MAP) estimate. We also show relationships of the proposed model to existing probabilistic classifiers as well as iteratively re-weighted l1 and l2 minimizations. We then study different types of likelihood working with the GGSM prior in kernel-based setup, based on which an improved kernel-based GGIG is presented. Experiments demonstrate that the proposed method has better or comparable performances in linear classifiers as well as in kernel-based classification.
► Deal with binary classification.
► A flexible probabilistic model using a generalized Gaussian scale mixture prior.
► Provide an appropriate degree of sparsity for its model parameters.
► Unify existing probabilistic classifiers.
► Support kernel-based setup.
Journal: Pattern Recognition - Volume 46, Issue 1, January 2013, Pages 332–345