کد مقاله | کد نشریه | سال انتشار | مقاله انگلیسی | نسخه تمام متن |
---|---|---|---|---|
531805 | 869876 | 2016 | 10 صفحه PDF | دانلود رایگان |
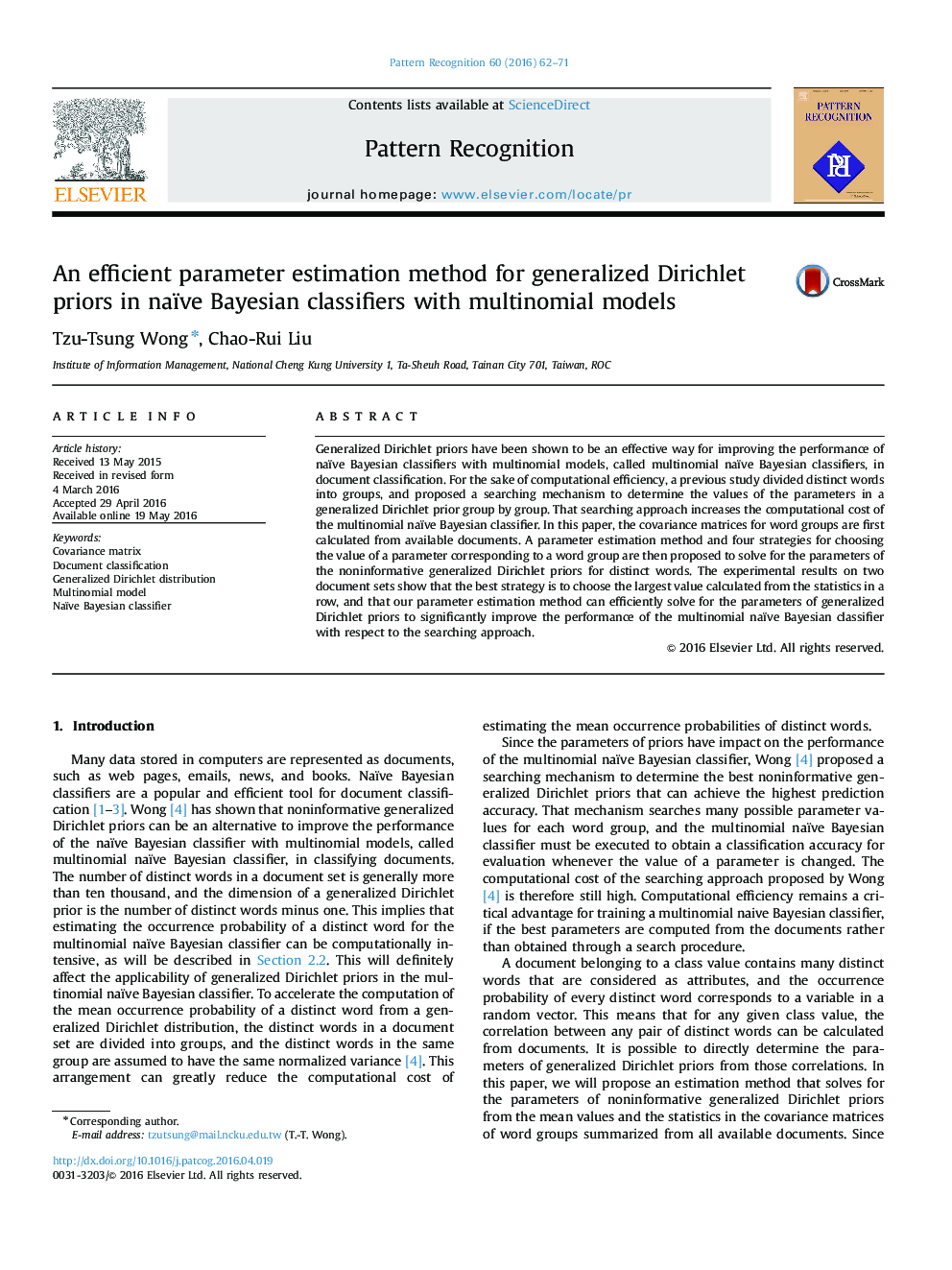
• An efficient method for constructing noninformative GD priors is proposed.
• The best strategy is to choose the largest candidate value for a parameter.
• A small default value is necessary for the distinct words with small frequencies.
• This method significantly improves the performance of naïve Bayesian classifiers.
Generalized Dirichlet priors have been shown to be an effective way for improving the performance of naïve Bayesian classifiers with multinomial models, called multinomial naïve Bayesian classifiers, in document classification. For the sake of computational efficiency, a previous study divided distinct words into groups, and proposed a searching mechanism to determine the values of the parameters in a generalized Dirichlet prior group by group. That searching approach increases the computational cost of the multinomial naïve Bayesian classifier. In this paper, the covariance matrices for word groups are first calculated from available documents. A parameter estimation method and four strategies for choosing the value of a parameter corresponding to a word group are then proposed to solve for the parameters of the noninformative generalized Dirichlet priors for distinct words. The experimental results on two document sets show that the best strategy is to choose the largest value calculated from the statistics in a row, and that our parameter estimation method can efficiently solve for the parameters of generalized Dirichlet priors to significantly improve the performance of the multinomial naïve Bayesian classifier with respect to the searching approach.
Journal: Pattern Recognition - Volume 60, December 2016, Pages 62–71