کد مقاله | کد نشریه | سال انتشار | مقاله انگلیسی | نسخه تمام متن |
---|---|---|---|---|
532219 | 869923 | 2013 | 10 صفحه PDF | دانلود رایگان |
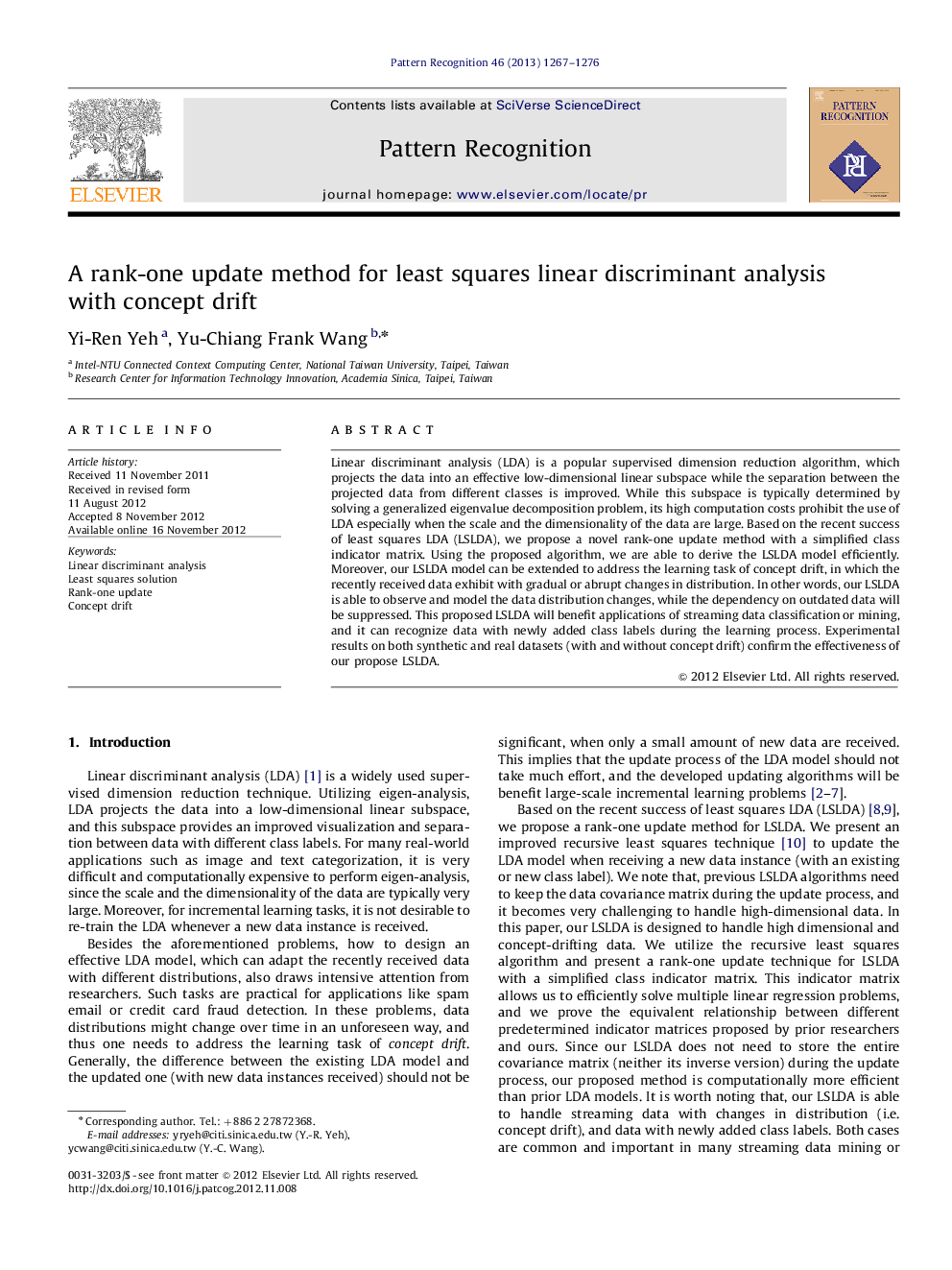
Linear discriminant analysis (LDA) is a popular supervised dimension reduction algorithm, which projects the data into an effective low-dimensional linear subspace while the separation between the projected data from different classes is improved. While this subspace is typically determined by solving a generalized eigenvalue decomposition problem, its high computation costs prohibit the use of LDA especially when the scale and the dimensionality of the data are large. Based on the recent success of least squares LDA (LSLDA), we propose a novel rank-one update method with a simplified class indicator matrix. Using the proposed algorithm, we are able to derive the LSLDA model efficiently. Moreover, our LSLDA model can be extended to address the learning task of concept drift, in which the recently received data exhibit with gradual or abrupt changes in distribution. In other words, our LSLDA is able to observe and model the data distribution changes, while the dependency on outdated data will be suppressed. This proposed LSLDA will benefit applications of streaming data classification or mining, and it can recognize data with newly added class labels during the learning process. Experimental results on both synthetic and real datasets (with and without concept drift) confirm the effectiveness of our propose LSLDA.
► A rank-one update method for least squares LDA (LSLDA) with concept drift is proposed.
► Our LSLDA with concept drift adapts to the distribution change of newly received data.
► A simplified class indicator matrix is proposed for our LSLDA model.
► We prove the equivalence between our proposed indicator matrix and prior ones.
► We verify the effectiveness of our LSLDA on problems with/without concept drift.
Journal: Pattern Recognition - Volume 46, Issue 5, May 2013, Pages 1267–1276