کد مقاله | کد نشریه | سال انتشار | مقاله انگلیسی | نسخه تمام متن |
---|---|---|---|---|
533126 | 870061 | 2016 | 14 صفحه PDF | دانلود رایگان |
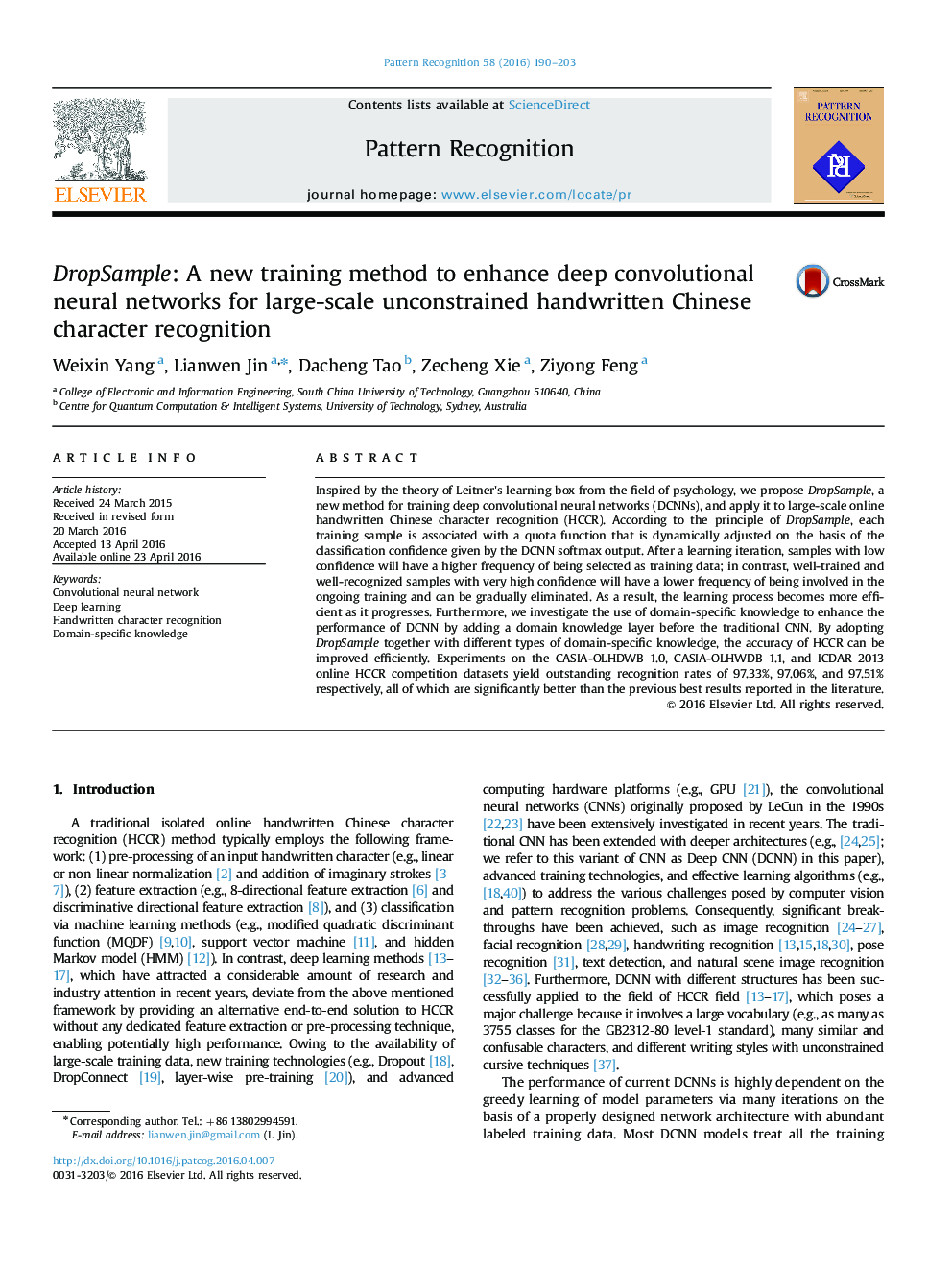
• We propose a novel and efficient training method for CNN on large-scale data.
• DropSample adaptively selects training samples and is robust to noisy data.
• The incorporation of domain-specific knowledge enhances the performance of CNN.
• New state-of-the-art results are reported on 3 online handwritten Chinese character datasets.
Inspired by the theory of Leitner׳s learning box from the field of psychology, we propose DropSample, a new method for training deep convolutional neural networks (DCNNs), and apply it to large-scale online handwritten Chinese character recognition (HCCR). According to the principle of DropSample, each training sample is associated with a quota function that is dynamically adjusted on the basis of the classification confidence given by the DCNN softmax output. After a learning iteration, samples with low confidence will have a higher frequency of being selected as training data; in contrast, well-trained and well-recognized samples with very high confidence will have a lower frequency of being involved in the ongoing training and can be gradually eliminated. As a result, the learning process becomes more efficient as it progresses. Furthermore, we investigate the use of domain-specific knowledge to enhance the performance of DCNN by adding a domain knowledge layer before the traditional CNN. By adopting DropSample together with different types of domain-specific knowledge, the accuracy of HCCR can be improved efficiently. Experiments on the CASIA-OLHDWB 1.0, CASIA-OLHWDB 1.1, and ICDAR 2013 online HCCR competition datasets yield outstanding recognition rates of 97.33%, 97.06%, and 97.51% respectively, all of which are significantly better than the previous best results reported in the literature.
Journal: Pattern Recognition - Volume 58, October 2016, Pages 190–203