کد مقاله | کد نشریه | سال انتشار | مقاله انگلیسی | نسخه تمام متن |
---|---|---|---|---|
533279 | 870092 | 2010 | 17 صفحه PDF | دانلود رایگان |
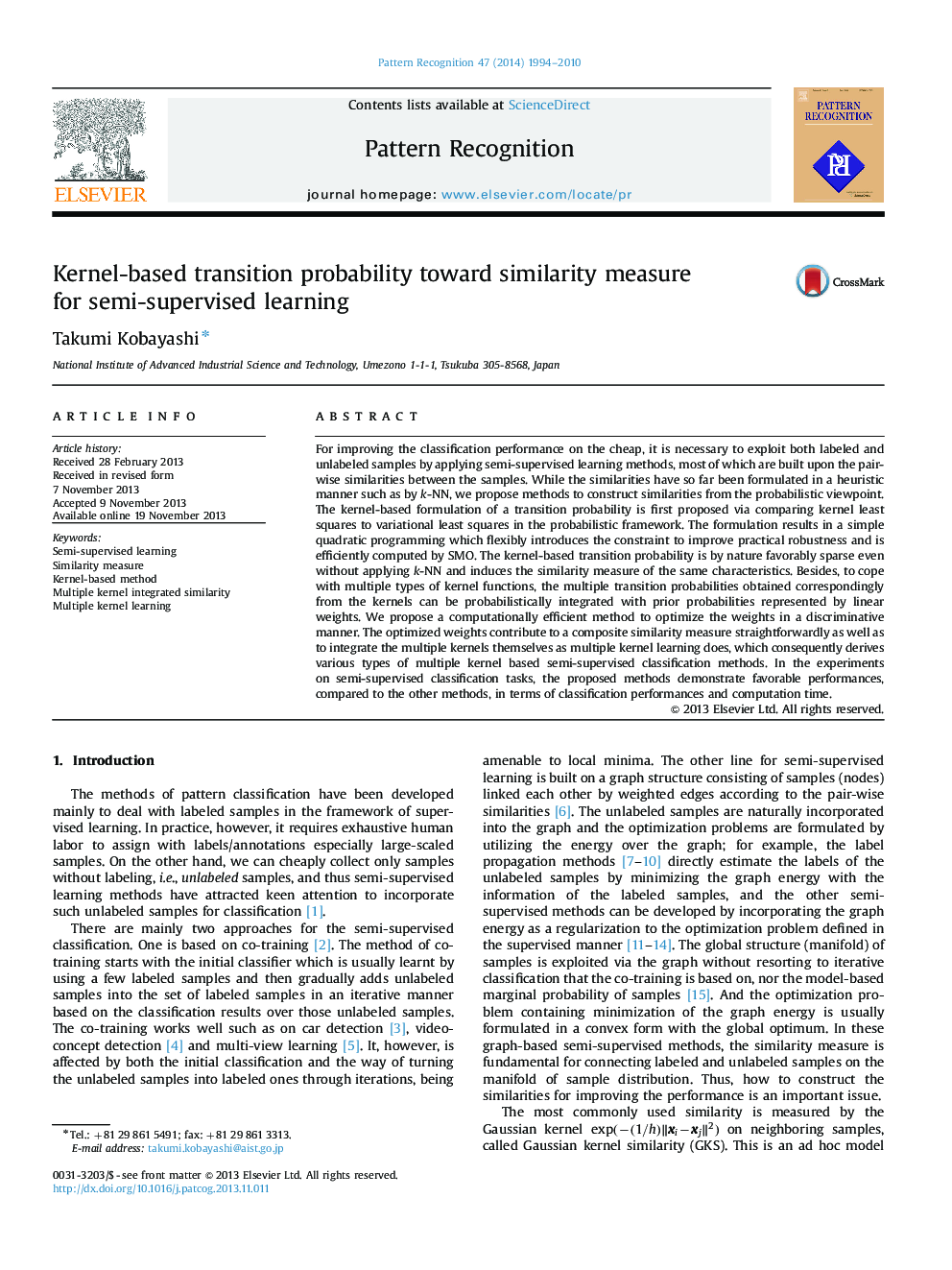
• We propose novel similarity measure from the probabilistic viewpoint.
• Kernel-based transition probabilities (KTPs) are fundamental to derive the similarity.
• A computationally efficient method is presented for computing KTP.
• Multiple kernels are probabilistically combined into novel similarity/kernel via KTP.
• The experimental results on various datasets exhibit favorable performance.
For improving the classification performance on the cheap, it is necessary to exploit both labeled and unlabeled samples by applying semi-supervised learning methods, most of which are built upon the pair-wise similarities between the samples. While the similarities have so far been formulated in a heuristic manner such as by k-NN, we propose methods to construct similarities from the probabilistic viewpoint. The kernel-based formulation of a transition probability is first proposed via comparing kernel least squares to variational least squares in the probabilistic framework. The formulation results in a simple quadratic programming which flexibly introduces the constraint to improve practical robustness and is efficiently computed by SMO. The kernel-based transition probability is by nature favorably sparse even without applying k-NN and induces the similarity measure of the same characteristics. Besides, to cope with multiple types of kernel functions, the multiple transition probabilities obtained correspondingly from the kernels can be probabilistically integrated with prior probabilities represented by linear weights. We propose a computationally efficient method to optimize the weights in a discriminative manner. The optimized weights contribute to a composite similarity measure straightforwardly as well as to integrate the multiple kernels themselves as multiple kernel learning does, which consequently derives various types of multiple kernel based semi-supervised classification methods. In the experiments on semi-supervised classification tasks, the proposed methods demonstrate favorable performances, compared to the other methods, in terms of classification performances and computation time.
Journal: Pattern Recognition - Volume 47, Issue 5, May 2014, Pages 1994–2010