کد مقاله | کد نشریه | سال انتشار | مقاله انگلیسی | نسخه تمام متن |
---|---|---|---|---|
533464 | 870118 | 2012 | 11 صفحه PDF | دانلود رایگان |
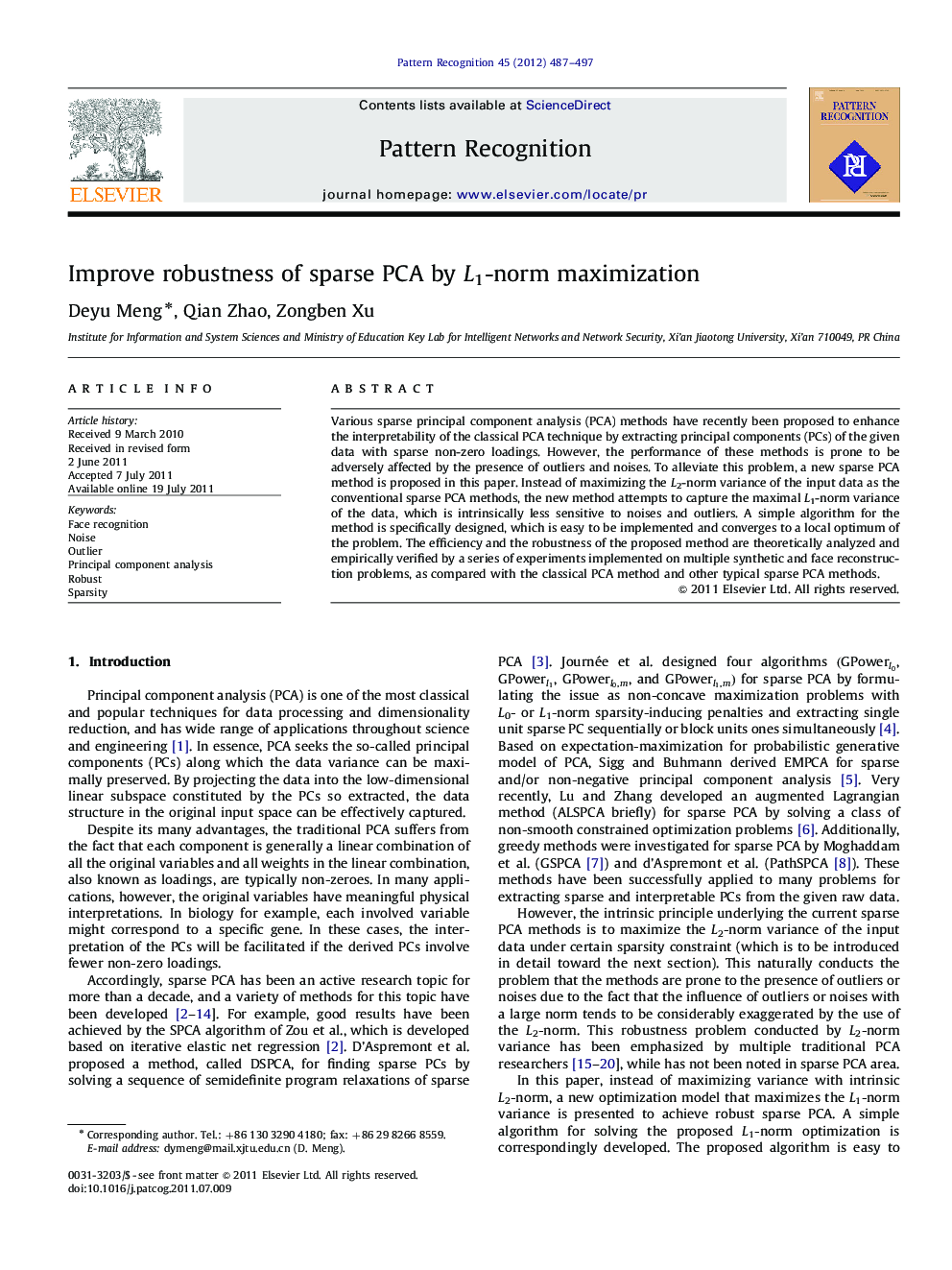
Various sparse principal component analysis (PCA) methods have recently been proposed to enhance the interpretability of the classical PCA technique by extracting principal components (PCs) of the given data with sparse non-zero loadings. However, the performance of these methods is prone to be adversely affected by the presence of outliers and noises. To alleviate this problem, a new sparse PCA method is proposed in this paper. Instead of maximizing the L2-norm variance of the input data as the conventional sparse PCA methods, the new method attempts to capture the maximal L1-norm variance of the data, which is intrinsically less sensitive to noises and outliers. A simple algorithm for the method is specifically designed, which is easy to be implemented and converges to a local optimum of the problem. The efficiency and the robustness of the proposed method are theoretically analyzed and empirically verified by a series of experiments implemented on multiple synthetic and face reconstruction problems, as compared with the classical PCA method and other typical sparse PCA methods.
► A new model is proposed to improve the robustness of sparse PCA by maximizing L1-norm data variance.
► A simple algorithm for the proposed model is designed.
► The robustness of the method is empirically verified by a series of experiments.
Journal: Pattern Recognition - Volume 45, Issue 1, January 2012, Pages 487–497