کد مقاله | کد نشریه | سال انتشار | مقاله انگلیسی | نسخه تمام متن |
---|---|---|---|---|
533873 | 870180 | 2014 | 15 صفحه PDF | دانلود رایگان |
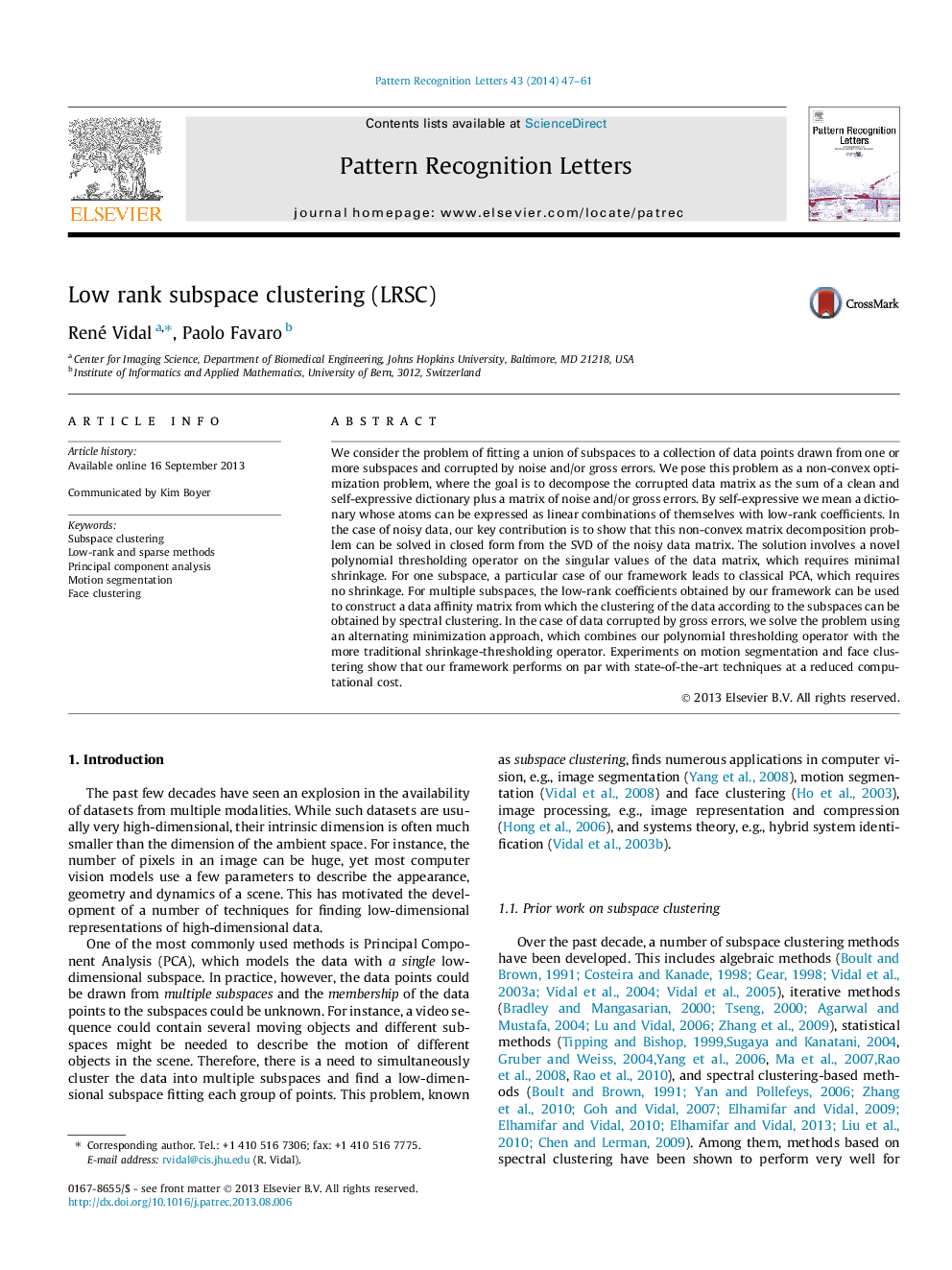
• We study the problem of clustering data lying approximately on multiple subspaces.
• We solve this problem by applying spectral clustering to a learned affinity matrix.
• We pose the affinity-learning problem as a non-convex matrix decomposition problem.
• We show that this problem can be solved in closed form from the SVD of the data.
• We evaluate our approach on the motion segmentation and face clustering problems.
We consider the problem of fitting a union of subspaces to a collection of data points drawn from one or more subspaces and corrupted by noise and/or gross errors. We pose this problem as a non-convex optimization problem, where the goal is to decompose the corrupted data matrix as the sum of a clean and self-expressive dictionary plus a matrix of noise and/or gross errors. By self-expressive we mean a dictionary whose atoms can be expressed as linear combinations of themselves with low-rank coefficients. In the case of noisy data, our key contribution is to show that this non-convex matrix decomposition problem can be solved in closed form from the SVD of the noisy data matrix. The solution involves a novel polynomial thresholding operator on the singular values of the data matrix, which requires minimal shrinkage. For one subspace, a particular case of our framework leads to classical PCA, which requires no shrinkage. For multiple subspaces, the low-rank coefficients obtained by our framework can be used to construct a data affinity matrix from which the clustering of the data according to the subspaces can be obtained by spectral clustering. In the case of data corrupted by gross errors, we solve the problem using an alternating minimization approach, which combines our polynomial thresholding operator with the more traditional shrinkage-thresholding operator. Experiments on motion segmentation and face clustering show that our framework performs on par with state-of-the-art techniques at a reduced computational cost.
Journal: Pattern Recognition Letters - Volume 43, 1 July 2014, Pages 47–61