کد مقاله | کد نشریه | سال انتشار | مقاله انگلیسی | نسخه تمام متن |
---|---|---|---|---|
534391 | 870249 | 2010 | 5 صفحه PDF | دانلود رایگان |
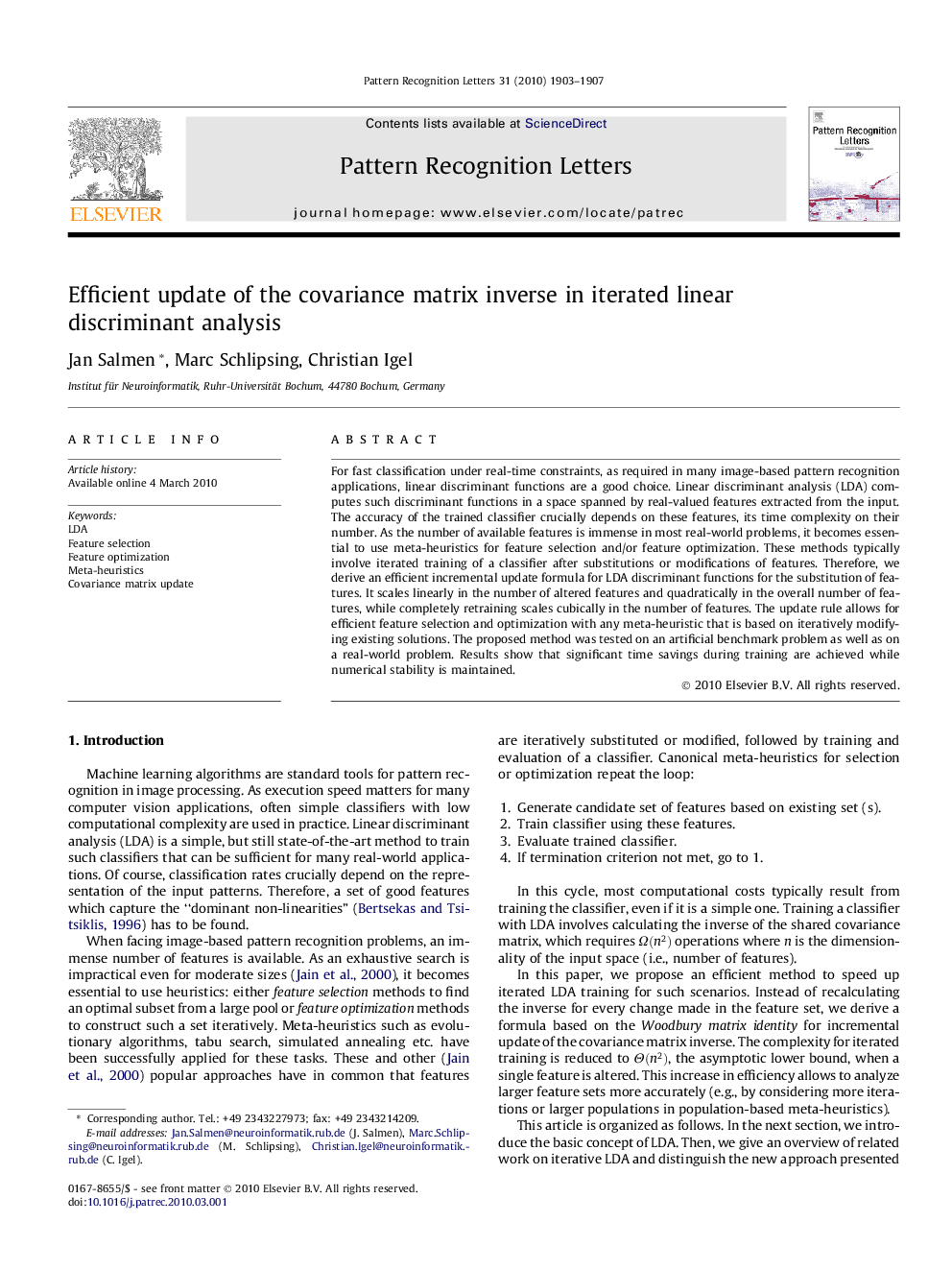
For fast classification under real-time constraints, as required in many image-based pattern recognition applications, linear discriminant functions are a good choice. Linear discriminant analysis (LDA) computes such discriminant functions in a space spanned by real-valued features extracted from the input. The accuracy of the trained classifier crucially depends on these features, its time complexity on their number. As the number of available features is immense in most real-world problems, it becomes essential to use meta-heuristics for feature selection and/or feature optimization. These methods typically involve iterated training of a classifier after substitutions or modifications of features. Therefore, we derive an efficient incremental update formula for LDA discriminant functions for the substitution of features. It scales linearly in the number of altered features and quadratically in the overall number of features, while completely retraining scales cubically in the number of features. The update rule allows for efficient feature selection and optimization with any meta-heuristic that is based on iteratively modifying existing solutions. The proposed method was tested on an artificial benchmark problem as well as on a real-world problem. Results show that significant time savings during training are achieved while numerical stability is maintained.
Journal: Pattern Recognition Letters - Volume 31, Issue 13, 1 October 2010, Pages 1903–1907