کد مقاله | کد نشریه | سال انتشار | مقاله انگلیسی | نسخه تمام متن |
---|---|---|---|---|
534813 | 870292 | 2010 | 14 صفحه PDF | دانلود رایگان |
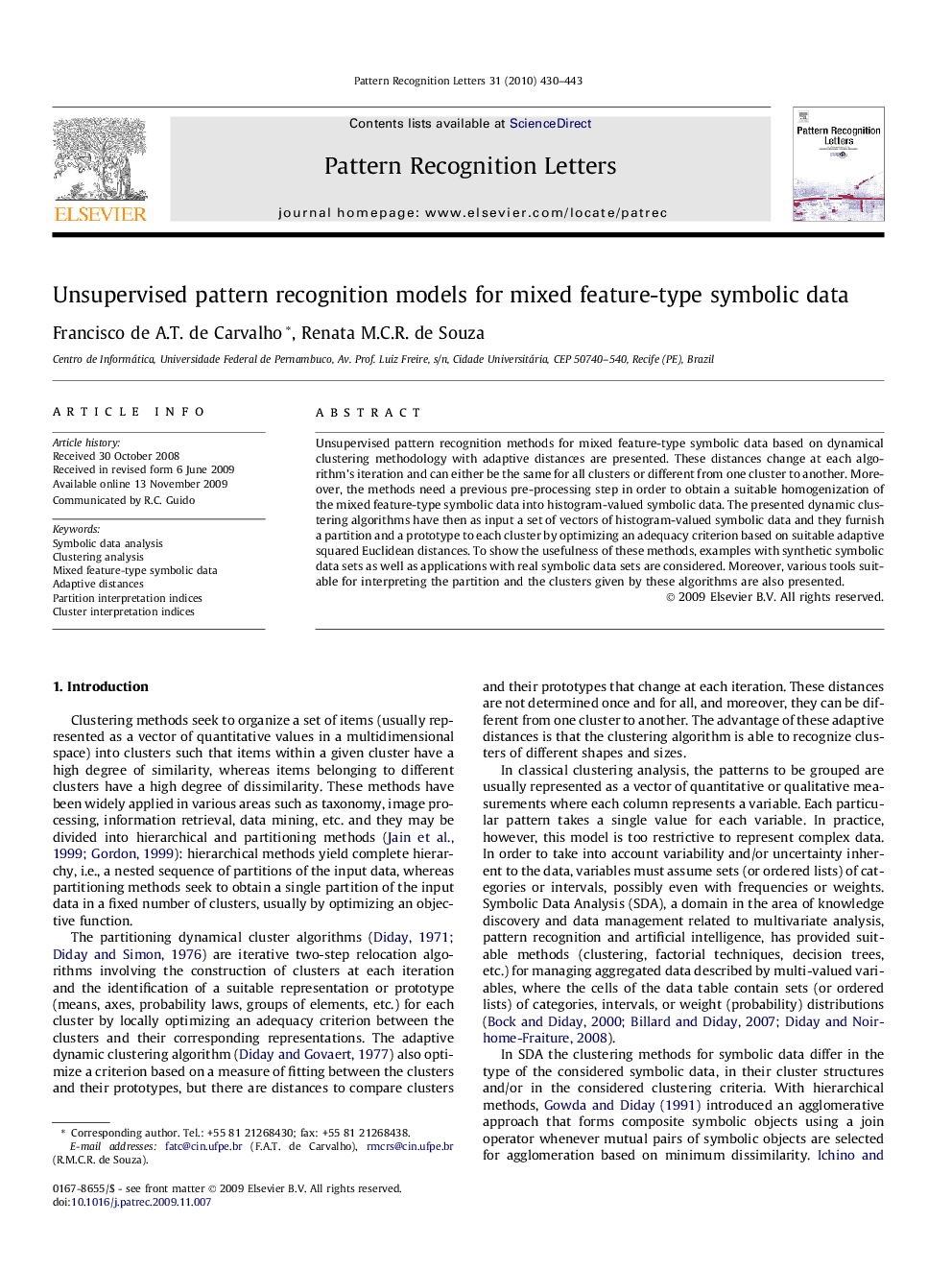
Unsupervised pattern recognition methods for mixed feature-type symbolic data based on dynamical clustering methodology with adaptive distances are presented. These distances change at each algorithm’s iteration and can either be the same for all clusters or different from one cluster to another. Moreover, the methods need a previous pre-processing step in order to obtain a suitable homogenization of the mixed feature-type symbolic data into histogram-valued symbolic data. The presented dynamic clustering algorithms have then as input a set of vectors of histogram-valued symbolic data and they furnish a partition and a prototype to each cluster by optimizing an adequacy criterion based on suitable adaptive squared Euclidean distances. To show the usefulness of these methods, examples with synthetic symbolic data sets as well as applications with real symbolic data sets are considered. Moreover, various tools suitable for interpreting the partition and the clusters given by these algorithms are also presented.
Journal: Pattern Recognition Letters - Volume 31, Issue 5, 1 April 2010, Pages 430–443