کد مقاله | کد نشریه | سال انتشار | مقاله انگلیسی | نسخه تمام متن |
---|---|---|---|---|
535126 | 870323 | 2009 | 9 صفحه PDF | دانلود رایگان |
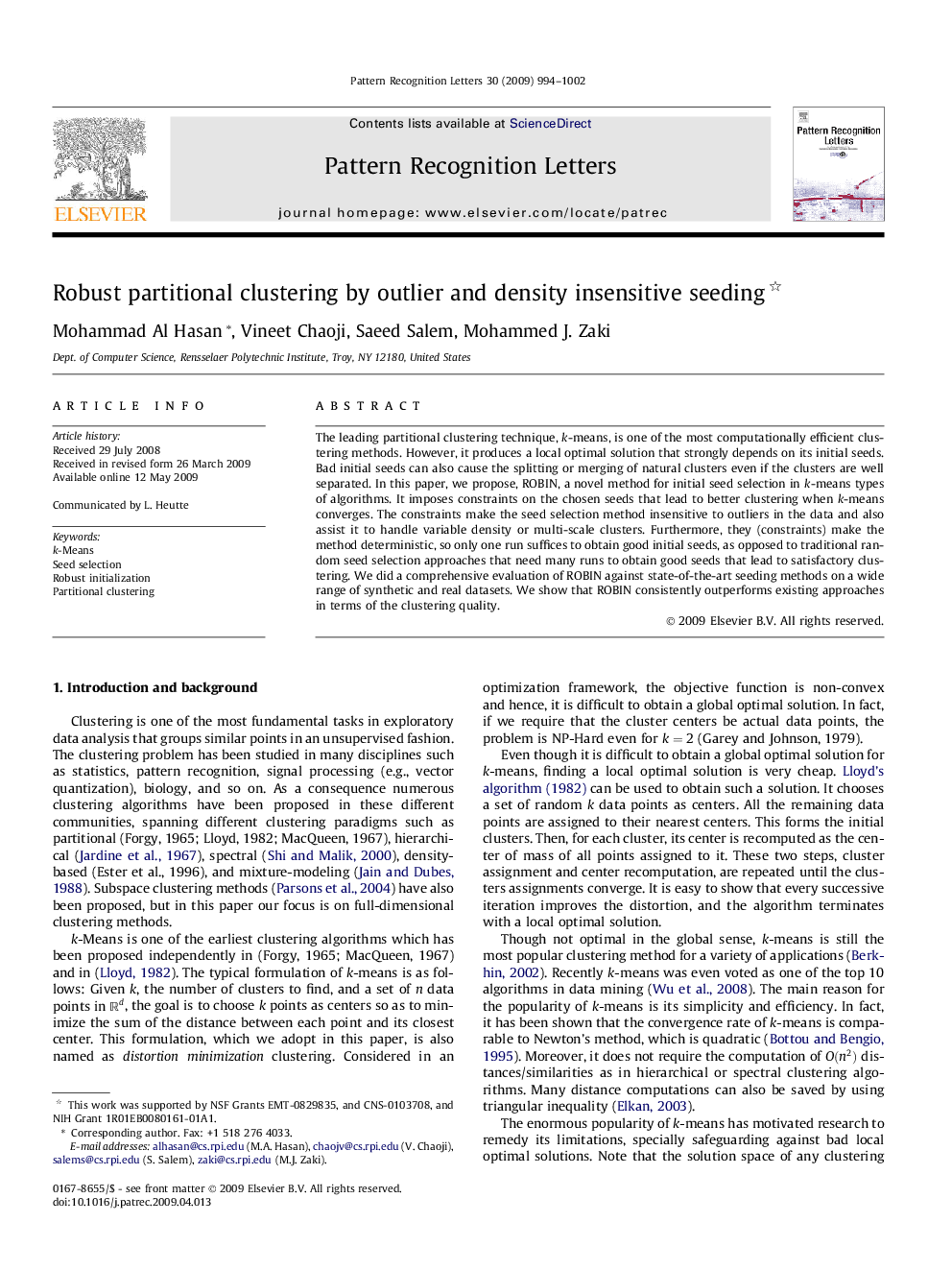
The leading partitional clustering technique, k-means, is one of the most computationally efficient clustering methods. However, it produces a local optimal solution that strongly depends on its initial seeds. Bad initial seeds can also cause the splitting or merging of natural clusters even if the clusters are well separated. In this paper, we propose, ROBIN, a novel method for initial seed selection in k-means types of algorithms. It imposes constraints on the chosen seeds that lead to better clustering when k-means converges. The constraints make the seed selection method insensitive to outliers in the data and also assist it to handle variable density or multi-scale clusters. Furthermore, they (constraints) make the method deterministic, so only one run suffices to obtain good initial seeds, as opposed to traditional random seed selection approaches that need many runs to obtain good seeds that lead to satisfactory clustering. We did a comprehensive evaluation of ROBIN against state-of-the-art seeding methods on a wide range of synthetic and real datasets. We show that ROBIN consistently outperforms existing approaches in terms of the clustering quality.
Journal: Pattern Recognition Letters - Volume 30, Issue 11, 1 August 2009, Pages 994–1002