کد مقاله | کد نشریه | سال انتشار | مقاله انگلیسی | نسخه تمام متن |
---|---|---|---|---|
535479 | 870349 | 2008 | 7 صفحه PDF | دانلود رایگان |
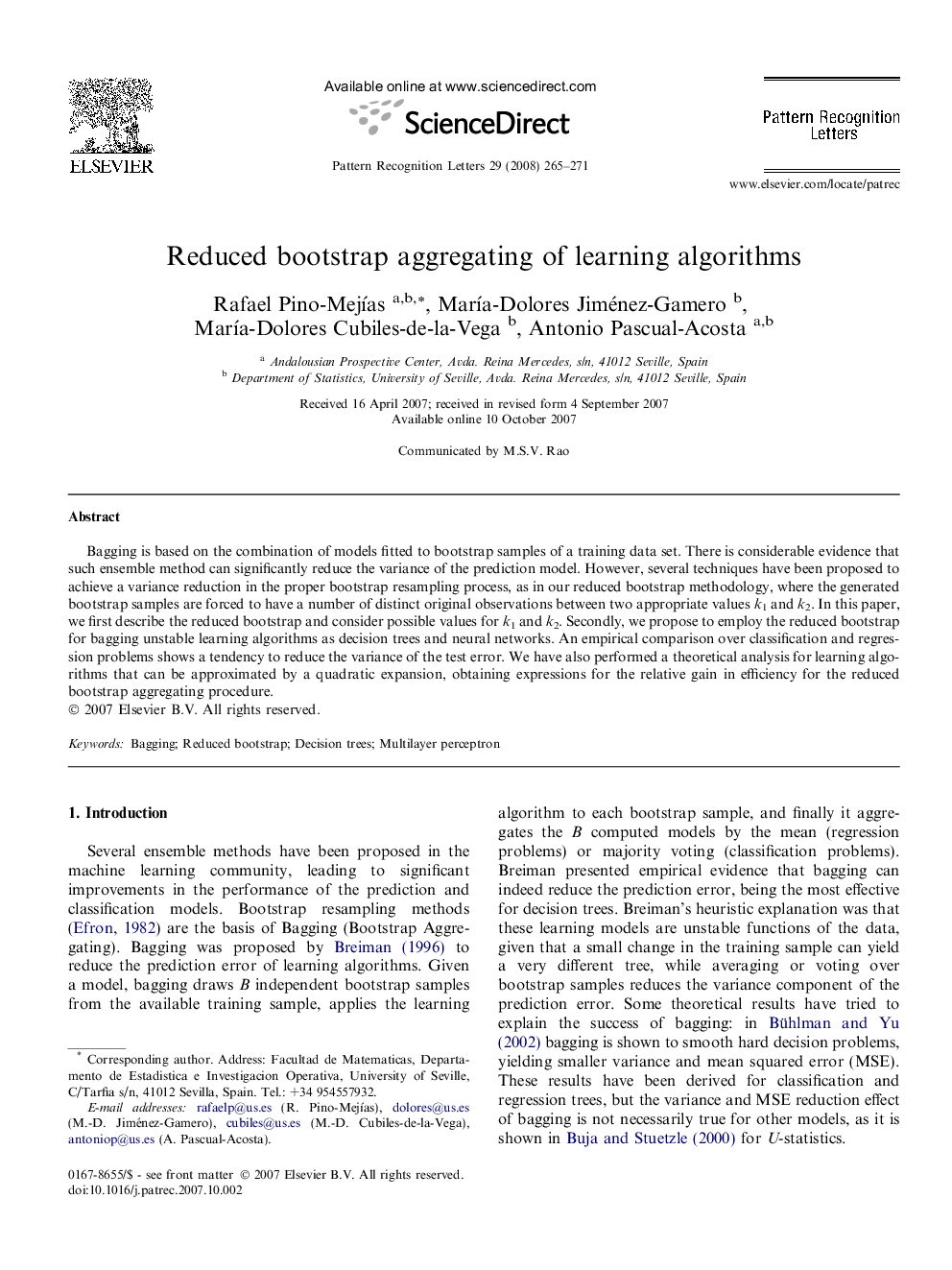
Bagging is based on the combination of models fitted to bootstrap samples of a training data set. There is considerable evidence that such ensemble method can significantly reduce the variance of the prediction model. However, several techniques have been proposed to achieve a variance reduction in the proper bootstrap resampling process, as in our reduced bootstrap methodology, where the generated bootstrap samples are forced to have a number of distinct original observations between two appropriate values k1 and k2. In this paper, we first describe the reduced bootstrap and consider possible values for k1 and k2. Secondly, we propose to employ the reduced bootstrap for bagging unstable learning algorithms as decision trees and neural networks. An empirical comparison over classification and regression problems shows a tendency to reduce the variance of the test error. We have also performed a theoretical analysis for learning algorithms that can be approximated by a quadratic expansion, obtaining expressions for the relative gain in efficiency for the reduced bootstrap aggregating procedure.
Journal: Pattern Recognition Letters - Volume 29, Issue 3, 1 February 2008, Pages 265–271