کد مقاله | کد نشریه | سال انتشار | مقاله انگلیسی | نسخه تمام متن |
---|---|---|---|---|
536407 | 870510 | 2013 | 7 صفحه PDF | دانلود رایگان |
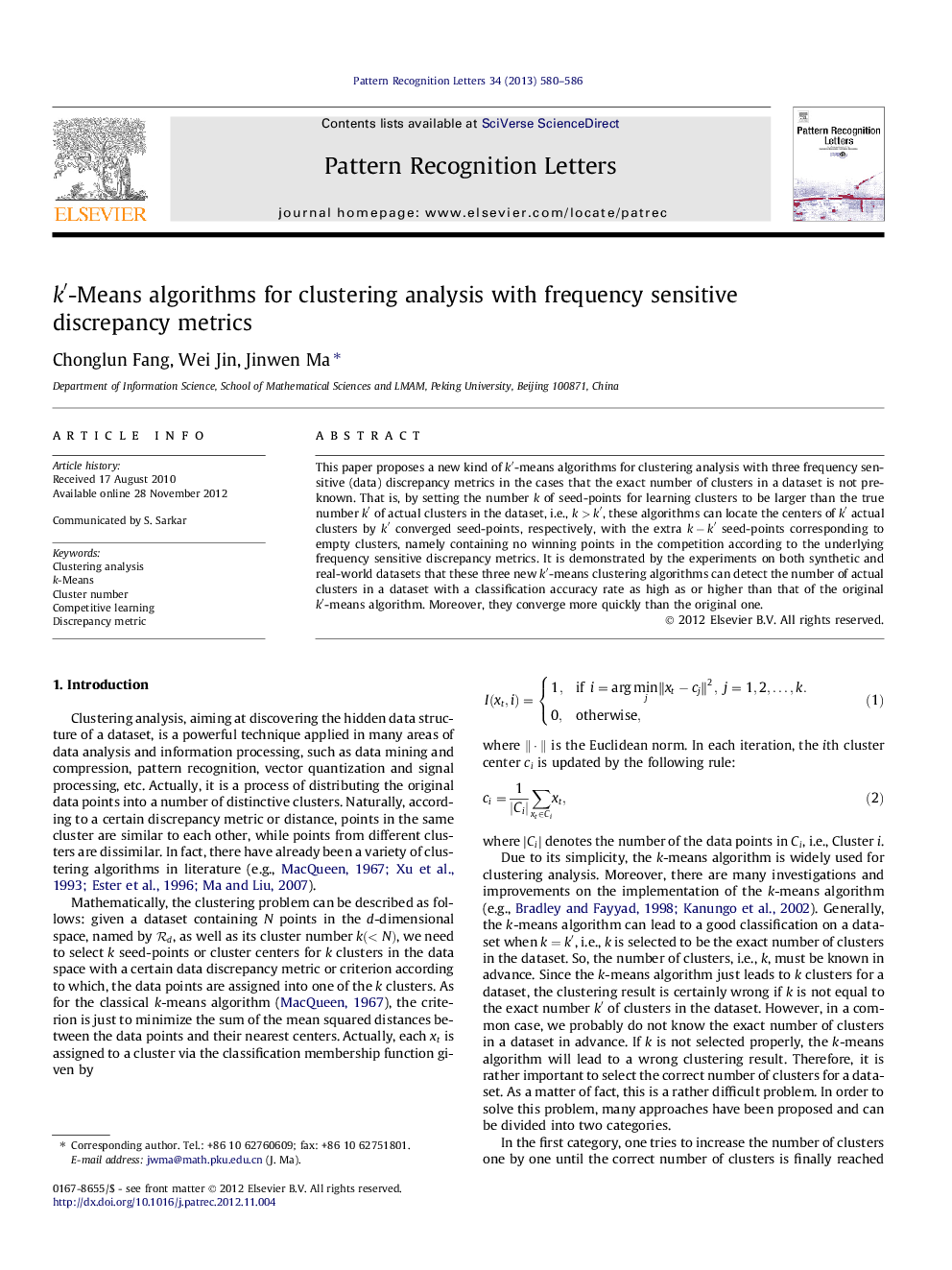
This paper proposes a new kind of k′k′-means algorithms for clustering analysis with three frequency sensitive (data) discrepancy metrics in the cases that the exact number of clusters in a dataset is not pre-known. That is, by setting the number k of seed-points for learning clusters to be larger than the true number k′k′ of actual clusters in the dataset, i.e., k>k′k>k′, these algorithms can locate the centers of k′k′ actual clusters by k′k′ converged seed-points, respectively, with the extra k-k′k-k′ seed-points corresponding to empty clusters, namely containing no winning points in the competition according to the underlying frequency sensitive discrepancy metrics. It is demonstrated by the experiments on both synthetic and real-world datasets that these three new k′k′-means clustering algorithms can detect the number of actual clusters in a dataset with a classification accuracy rate as high as or higher than that of the original k′k′-means algorithm. Moreover, they converge more quickly than the original one.
► We propose three new k′k′-means algorithms based on frequency sensitive discrepancy metrics.
► They are able to detect the number of actual clusters in a dataset automatically.
► They can obtain a better classification accuracy rate on a real-world dataset than the original k′k′-means algorithm.
► They converge more quickly than the original k′k′-means algorithm.
Journal: Pattern Recognition Letters - Volume 34, Issue 5, 1 April 2013, Pages 580–586