کد مقاله | کد نشریه | سال انتشار | مقاله انگلیسی | نسخه تمام متن |
---|---|---|---|---|
536475 | 870534 | 2012 | 7 صفحه PDF | دانلود رایگان |
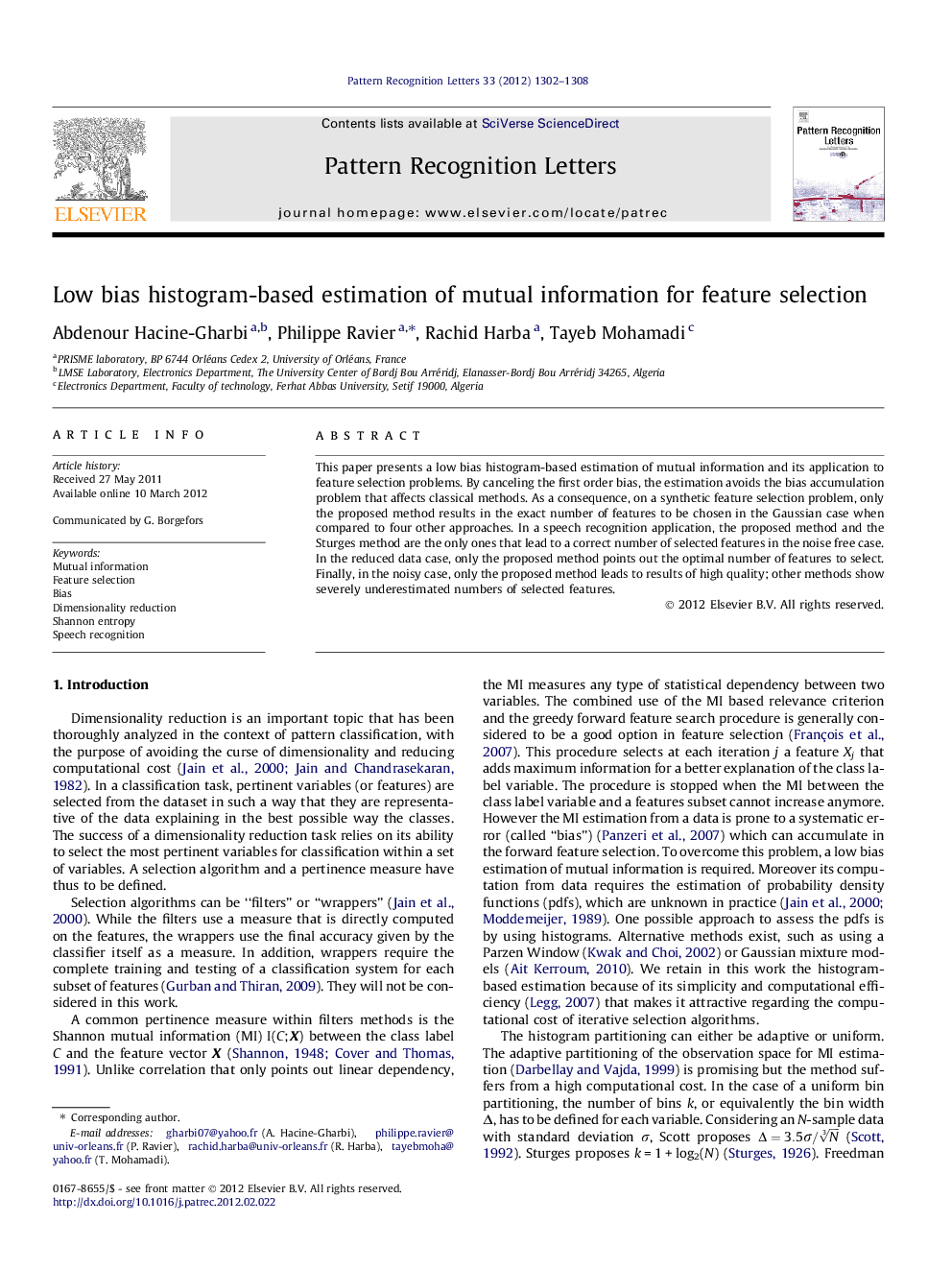
This paper presents a low bias histogram-based estimation of mutual information and its application to feature selection problems. By canceling the first order bias, the estimation avoids the bias accumulation problem that affects classical methods. As a consequence, on a synthetic feature selection problem, only the proposed method results in the exact number of features to be chosen in the Gaussian case when compared to four other approaches. In a speech recognition application, the proposed method and the Sturges method are the only ones that lead to a correct number of selected features in the noise free case. In the reduced data case, only the proposed method points out the optimal number of features to select. Finally, in the noisy case, only the proposed method leads to results of high quality; other methods show severely underestimated numbers of selected features.
► Low-bias histogram-based estimation of entropy and mutual information are proposed.
► Low-bias entropy and mutual information estimation improves the greedy algorithms efficiency.
► The proposed method permits to retrieve theoretical expected results when other methods fail.
► The correct number of features is selected in a speech recognition problem.
Journal: Pattern Recognition Letters - Volume 33, Issue 10, 15 July 2012, Pages 1302–1308