کد مقاله | کد نشریه | سال انتشار | مقاله انگلیسی | نسخه تمام متن |
---|---|---|---|---|
552070 | 873171 | 2013 | 11 صفحه PDF | دانلود رایگان |
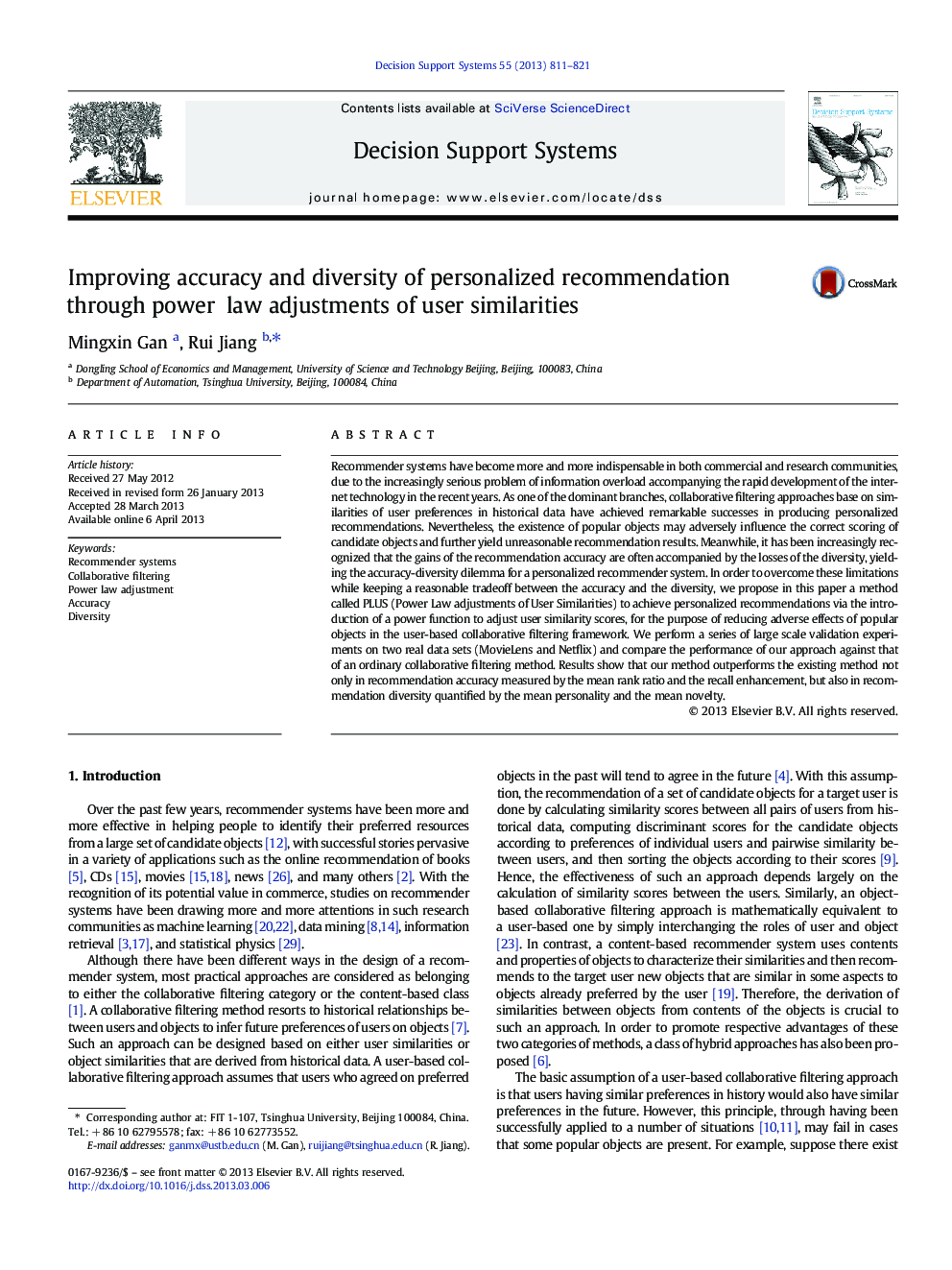
• The existence of popular objects may yield unreasonable recommendation results.
• We propose a method called PLUS to adjust user similarities using a power function.
• We show the superior performance of PLUS by large-scale validation experiments.
• PLUS achieves a reasonable tradeoff between recommendation accuracy and diversity.
• PLUS is robust to similarity measures and consistent between different data sets.
Recommender systems have become more and more indispensable in both commercial and research communities, due to the increasingly serious problem of information overload accompanying the rapid development of the internet technology in the recent years. As one of the dominant branches, collaborative filtering approaches base on similarities of user preferences in historical data have achieved remarkable successes in producing personalized recommendations. Nevertheless, the existence of popular objects may adversely influence the correct scoring of candidate objects and further yield unreasonable recommendation results. Meanwhile, it has been increasingly recognized that the gains of the recommendation accuracy are often accompanied by the losses of the diversity, yielding the accuracy-diversity dilemma for a personalized recommender system. In order to overcome these limitations while keeping a reasonable tradeoff between the accuracy and the diversity, we propose in this paper a method called PLUS (Power Law adjustments of User Similarities) to achieve personalized recommendations via the introduction of a power function to adjust user similarity scores, for the purpose of reducing adverse effects of popular objects in the user-based collaborative filtering framework. We perform a series of large scale validation experiments on two real data sets (MovieLens and Netflix) and compare the performance of our approach against that of an ordinary collaborative filtering method. Results show that our method outperforms the existing method not only in recommendation accuracy measured by the mean rank ratio and the recall enhancement, but also in recommendation diversity quantified by the mean personality and the mean novelty.
Journal: Decision Support Systems - Volume 55, Issue 3, June 2013, Pages 811–821