کد مقاله | کد نشریه | سال انتشار | مقاله انگلیسی | نسخه تمام متن |
---|---|---|---|---|
553616 | 873520 | 2012 | 9 صفحه PDF | دانلود رایگان |
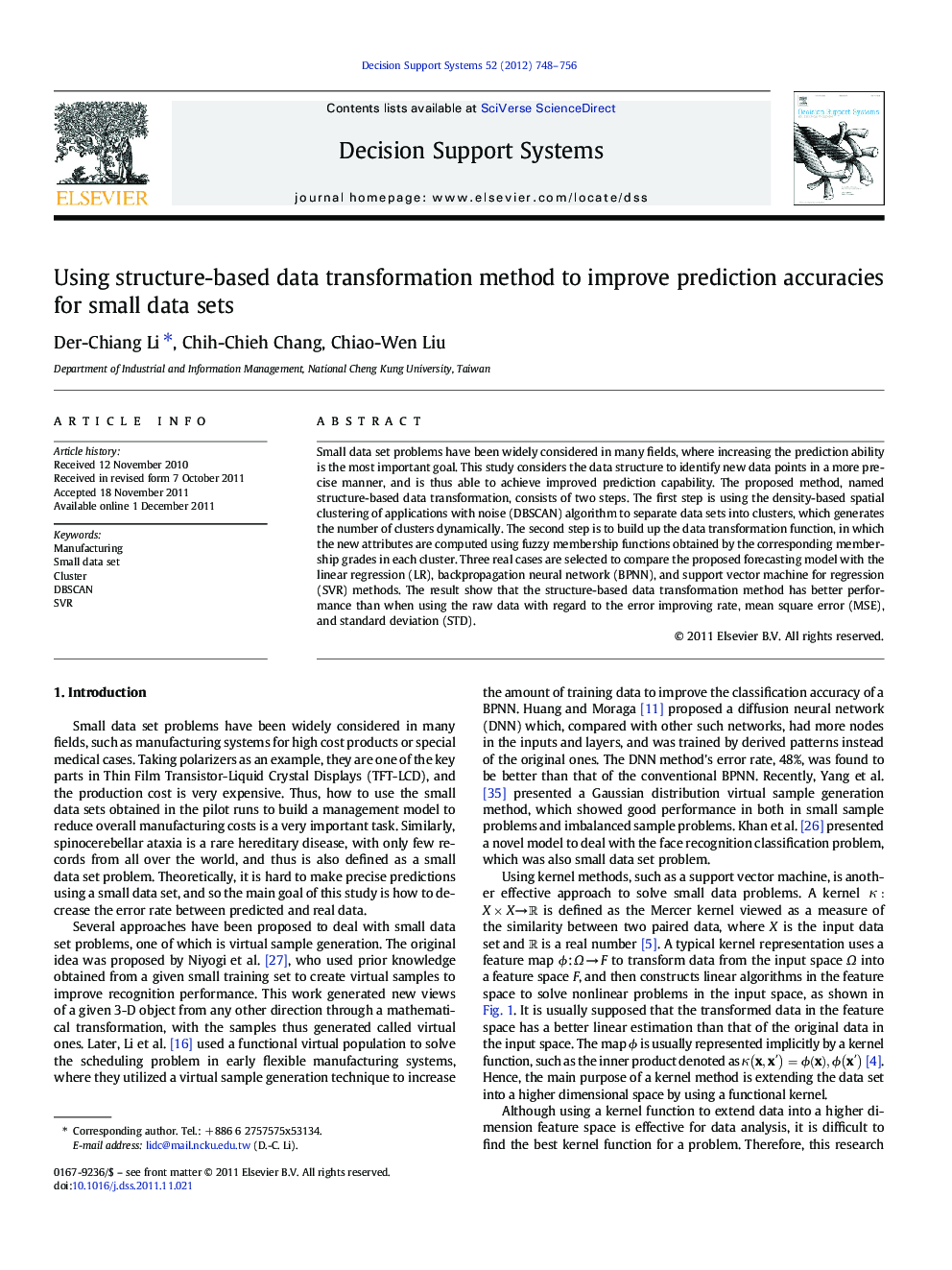
Small data set problems have been widely considered in many fields, where increasing the prediction ability is the most important goal. This study considers the data structure to identify new data points in a more precise manner, and is thus able to achieve improved prediction capability. The proposed method, named structure-based data transformation, consists of two steps. The first step is using the density-based spatial clustering of applications with noise (DBSCAN) algorithm to separate data sets into clusters, which generates the number of clusters dynamically. The second step is to build up the data transformation function, in which the new attributes are computed using fuzzy membership functions obtained by the corresponding membership grades in each cluster. Three real cases are selected to compare the proposed forecasting model with the linear regression (LR), backpropagation neural network (BPNN), and support vector machine for regression (SVR) methods. The result show that the structure-based data transformation method has better performance than when using the raw data with regard to the error improving rate, mean square error (MSE), and standard deviation (STD).
► This study presents structure-based data transformation to achieve improved prediction capability for small data sets.
► Using a function kernel to extend the data set into a higher dimensional space.
► Three real cases show better performance by using the proposed method.
Journal: Decision Support Systems - Volume 52, Issue 3, February 2012, Pages 748–756