کد مقاله | کد نشریه | سال انتشار | مقاله انگلیسی | نسخه تمام متن |
---|---|---|---|---|
555957 | 1451269 | 2015 | 13 صفحه PDF | دانلود رایگان |
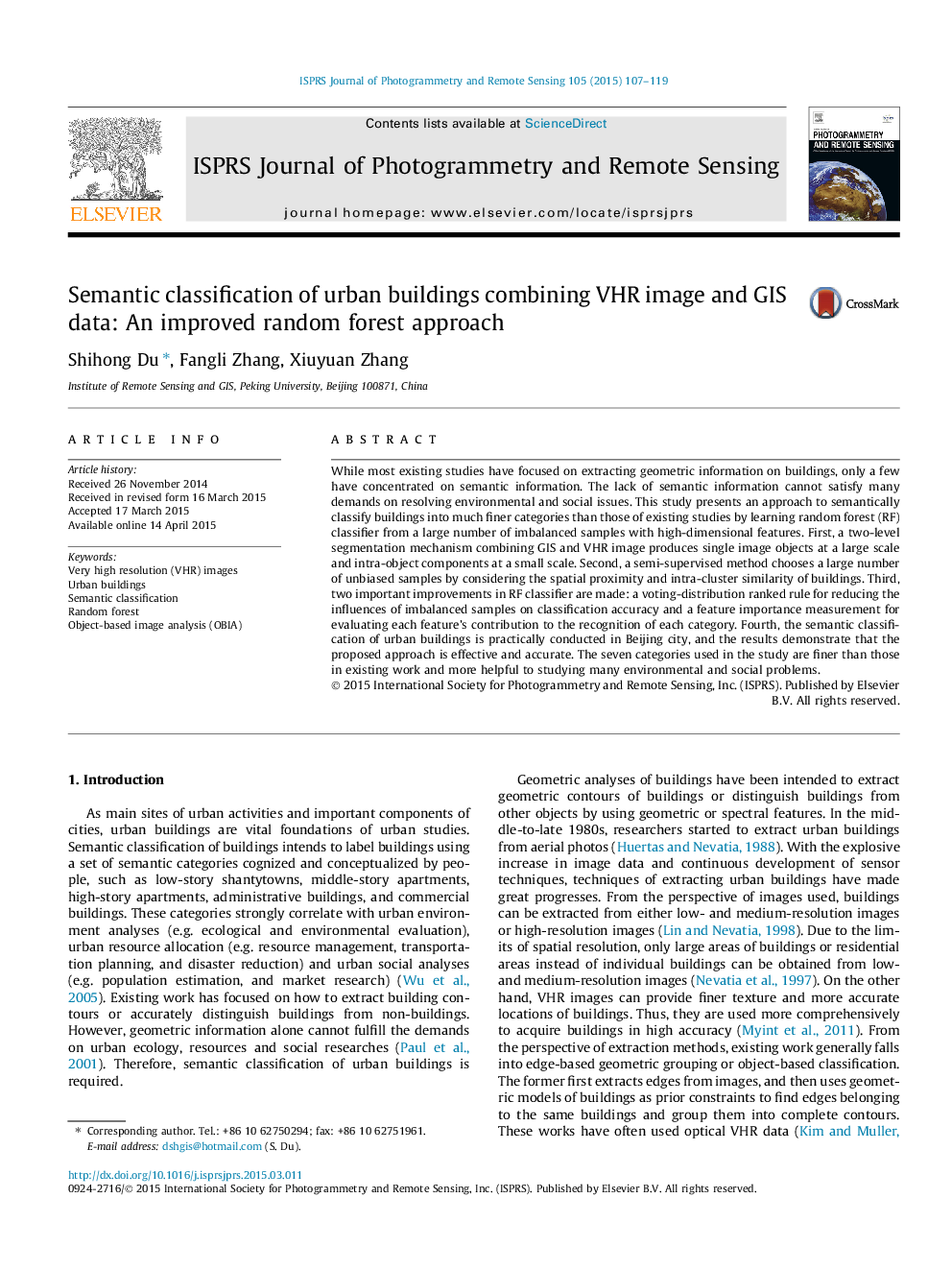
While most existing studies have focused on extracting geometric information on buildings, only a few have concentrated on semantic information. The lack of semantic information cannot satisfy many demands on resolving environmental and social issues. This study presents an approach to semantically classify buildings into much finer categories than those of existing studies by learning random forest (RF) classifier from a large number of imbalanced samples with high-dimensional features. First, a two-level segmentation mechanism combining GIS and VHR image produces single image objects at a large scale and intra-object components at a small scale. Second, a semi-supervised method chooses a large number of unbiased samples by considering the spatial proximity and intra-cluster similarity of buildings. Third, two important improvements in RF classifier are made: a voting-distribution ranked rule for reducing the influences of imbalanced samples on classification accuracy and a feature importance measurement for evaluating each feature’s contribution to the recognition of each category. Fourth, the semantic classification of urban buildings is practically conducted in Beijing city, and the results demonstrate that the proposed approach is effective and accurate. The seven categories used in the study are finer than those in existing work and more helpful to studying many environmental and social problems.
Journal: ISPRS Journal of Photogrammetry and Remote Sensing - Volume 105, July 2015, Pages 107–119