کد مقاله | کد نشریه | سال انتشار | مقاله انگلیسی | نسخه تمام متن |
---|---|---|---|---|
562637 | 875423 | 2013 | 7 صفحه PDF | دانلود رایگان |
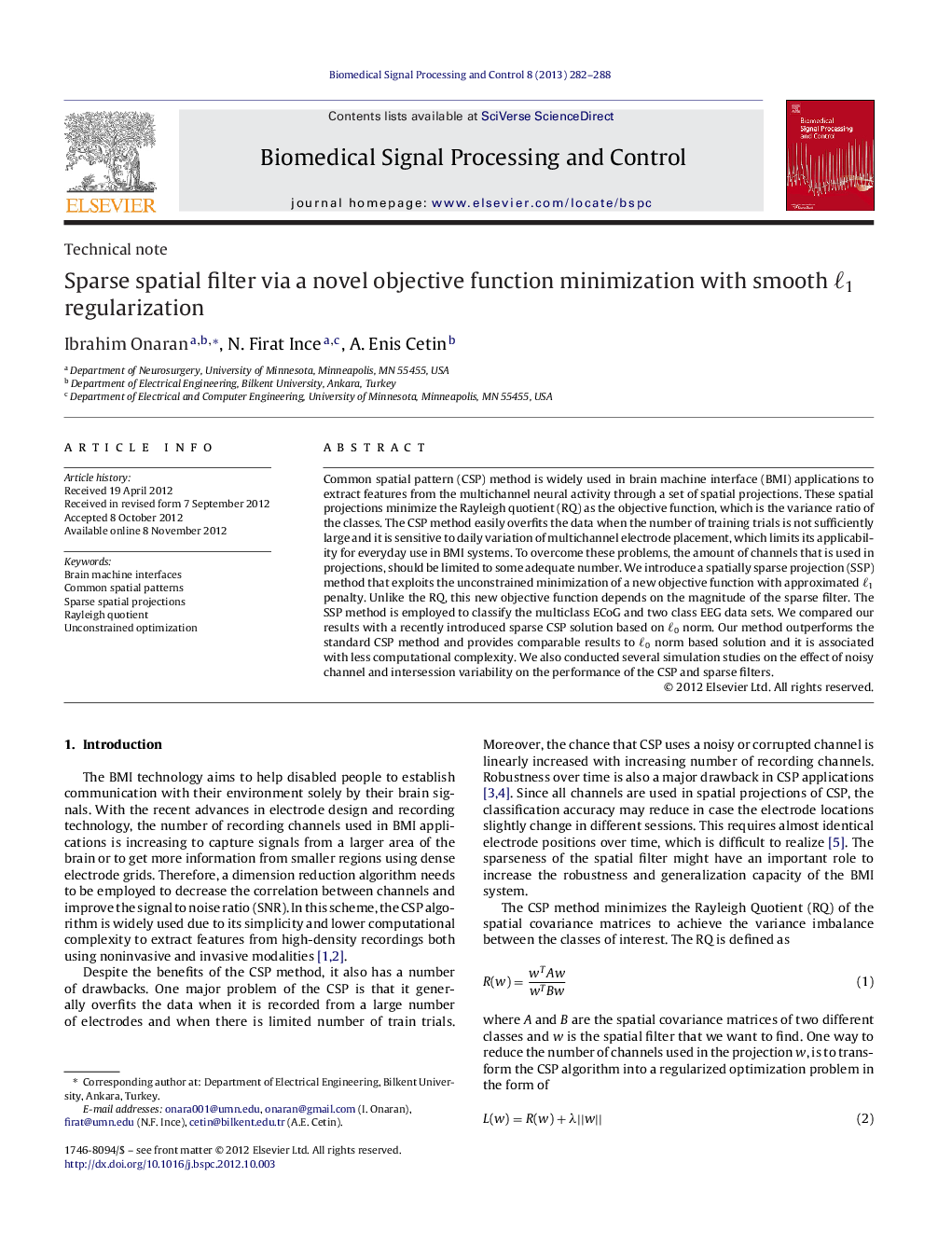
Common spatial pattern (CSP) method is widely used in brain machine interface (BMI) applications to extract features from the multichannel neural activity through a set of spatial projections. These spatial projections minimize the Rayleigh quotient (RQ) as the objective function, which is the variance ratio of the classes. The CSP method easily overfits the data when the number of training trials is not sufficiently large and it is sensitive to daily variation of multichannel electrode placement, which limits its applicability for everyday use in BMI systems. To overcome these problems, the amount of channels that is used in projections, should be limited to some adequate number. We introduce a spatially sparse projection (SSP) method that exploits the unconstrained minimization of a new objective function with approximated ℓ1 penalty. Unlike the RQ, this new objective function depends on the magnitude of the sparse filter. The SSP method is employed to classify the multiclass ECoG and two class EEG data sets. We compared our results with a recently introduced sparse CSP solution based on ℓ0 norm. Our method outperforms the standard CSP method and provides comparable results to ℓ0 norm based solution and it is associated with less computational complexity. We also conducted several simulation studies on the effect of noisy channel and intersession variability on the performance of the CSP and sparse filters.
► We introduce a spatially sparse projection (SSP) method.
► The method exploits the unconstrained minimization of a new objective function.
► ℓ1 penalty is used in minimization problem to provide sparse sapatial filters.
► ECoG and EEG data shows that our method gives better or comparable results to ℓ0.
► The complexity of the algoritm less than ℓ0 based sparse filters.
Journal: Biomedical Signal Processing and Control - Volume 8, Issue 3, May 2013, Pages 282–288