کد مقاله | کد نشریه | سال انتشار | مقاله انگلیسی | نسخه تمام متن |
---|---|---|---|---|
562893 | 1451958 | 2015 | 13 صفحه PDF | دانلود رایگان |
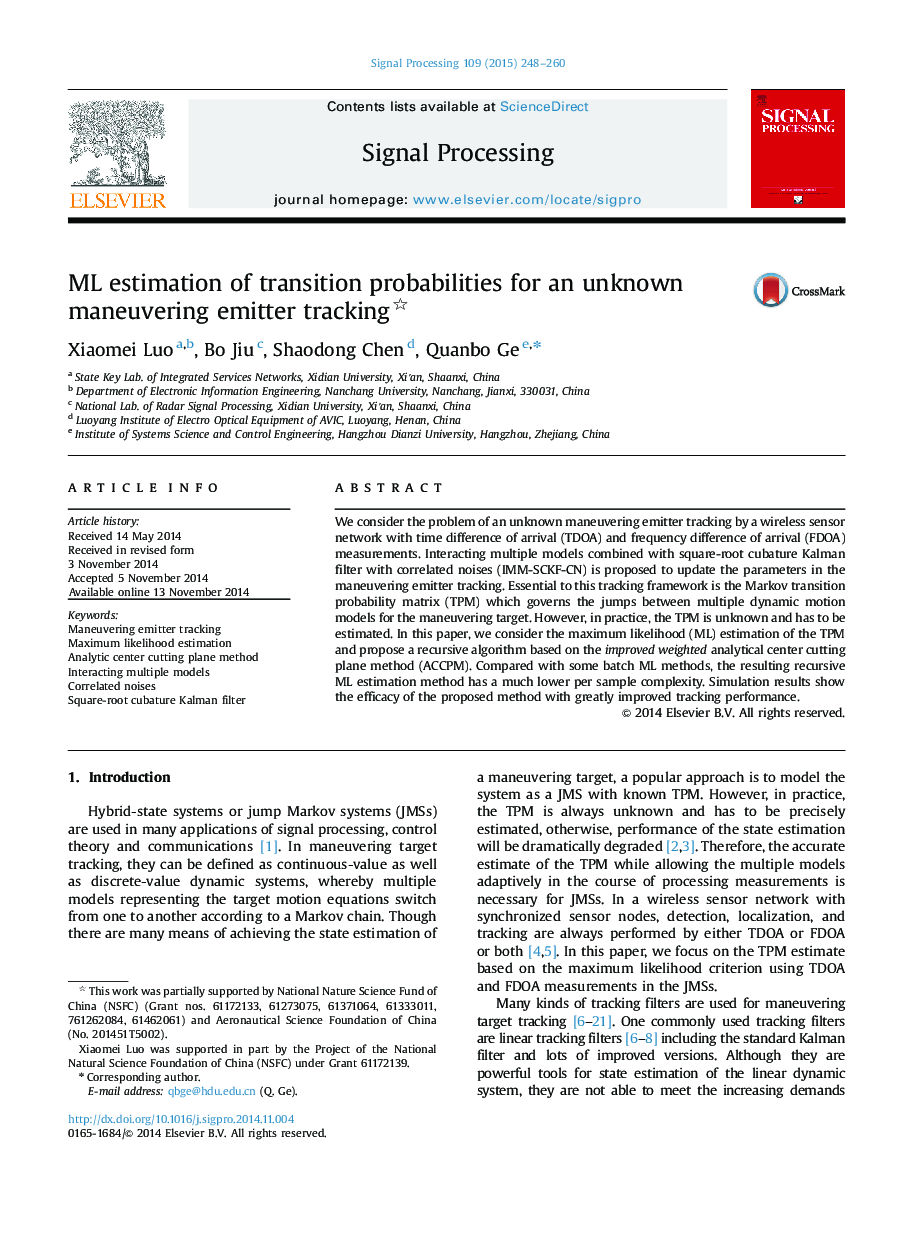
This paper studies estimation of the TPM of an unknown maneuvering emitter tracking by a wireless sensor network with TDOA and FDOA measurements. The work includes mainly two phases. Firstly, it uses SCKF instead of EKF which is used in our previous work as mode matching filter in the IMM algorithm. Therefore the corresponding IMM-SCKF-CN algorithm is proposed to update the parameters in the optimization problem for the TPM estimate. Afterwards, it extends our previous ML TPM estimate work. An improved weighted analytical center cutting plane method (ACCPM) is proposed to update the ML TPM estimate.
• Due to the drawbacks of EKF and advantages of SCKF, SCKF as mode matching was applied in the IMM algorithm.
• Due to the correlation existed between process and measurement noise in the field of target tracking in reality, SCKF-CN was applied to deal with this situation.
• IMM-SCKF-CN was devised to update the parameters in the optimization problem for the TPM estimate.
• The weighted ACCPM was proposed to recursively update the ML TPM estimate.
• Four simulation examples were demonstrated.
We consider the problem of an unknown maneuvering emitter tracking by a wireless sensor network with time difference of arrival (TDOA) and frequency difference of arrival (FDOA) measurements. Interacting multiple models combined with square-root cubature Kalman filter with correlated noises (IMM-SCKF-CN) is proposed to update the parameters in the maneuvering emitter tracking. Essential to this tracking framework is the Markov transition probability matrix (TPM) which governs the jumps between multiple dynamic motion models for the maneuvering target. However, in practice, the TPM is unknown and has to be estimated. In this paper, we consider the maximum likelihood (ML) estimation of the TPM and propose a recursive algorithm based on the improved weighted analytical center cutting plane method (ACCPM). Compared with some batch ML methods, the resulting recursive ML estimation method has a much lower per sample complexity. Simulation results show the efficacy of the proposed method with greatly improved tracking performance.
Journal: Signal Processing - Volume 109, April 2015, Pages 248–260