کد مقاله | کد نشریه | سال انتشار | مقاله انگلیسی | نسخه تمام متن |
---|---|---|---|---|
562909 | 1451964 | 2014 | 12 صفحه PDF | دانلود رایگان |
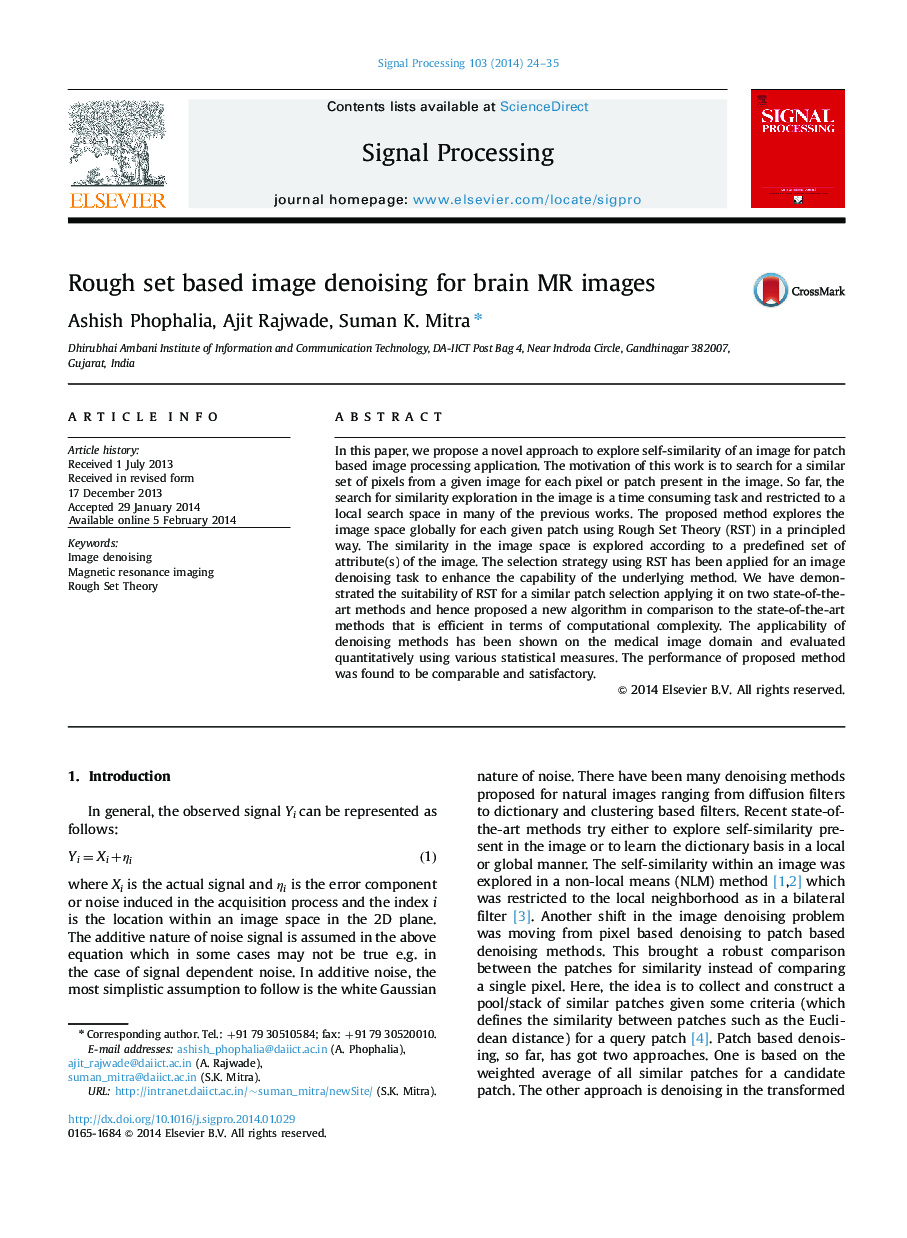
• The soft computing, in the precise Rough Set Theory (RST) based Medical Image Denoising problem, is addressed in the work.
• RST based framework is proposed to find similar patches from the single image for denoising purpose.
• The proposal is to release the local search constraint for similar patches from state-of-the-art methods.
• The performance of all the proposals has been compared with state-of-the-art methods and found to be satisfactory.
In this paper, we propose a novel approach to explore self-similarity of an image for patch based image processing application. The motivation of this work is to search for a similar set of pixels from a given image for each pixel or patch present in the image. So far, the search for similarity exploration in the image is a time consuming task and restricted to a local search space in many of the previous works. The proposed method explores the image space globally for each given patch using Rough Set Theory (RST) in a principled way. The similarity in the image space is explored according to a predefined set of attribute(s) of the image. The selection strategy using RST has been applied for an image denoising task to enhance the capability of the underlying method. We have demonstrated the suitability of RST for a similar patch selection applying it on two state-of-the-art methods and hence proposed a new algorithm in comparison to the state-of-the-art methods that is efficient in terms of computational complexity. The applicability of denoising methods has been shown on the medical image domain and evaluated quantitatively using various statistical measures. The performance of proposed method was found to be comparable and satisfactory.
Journal: Signal Processing - Volume 103, October 2014, Pages 24–35