کد مقاله | کد نشریه | سال انتشار | مقاله انگلیسی | نسخه تمام متن |
---|---|---|---|---|
563233 | 875482 | 2009 | 19 صفحه PDF | دانلود رایگان |
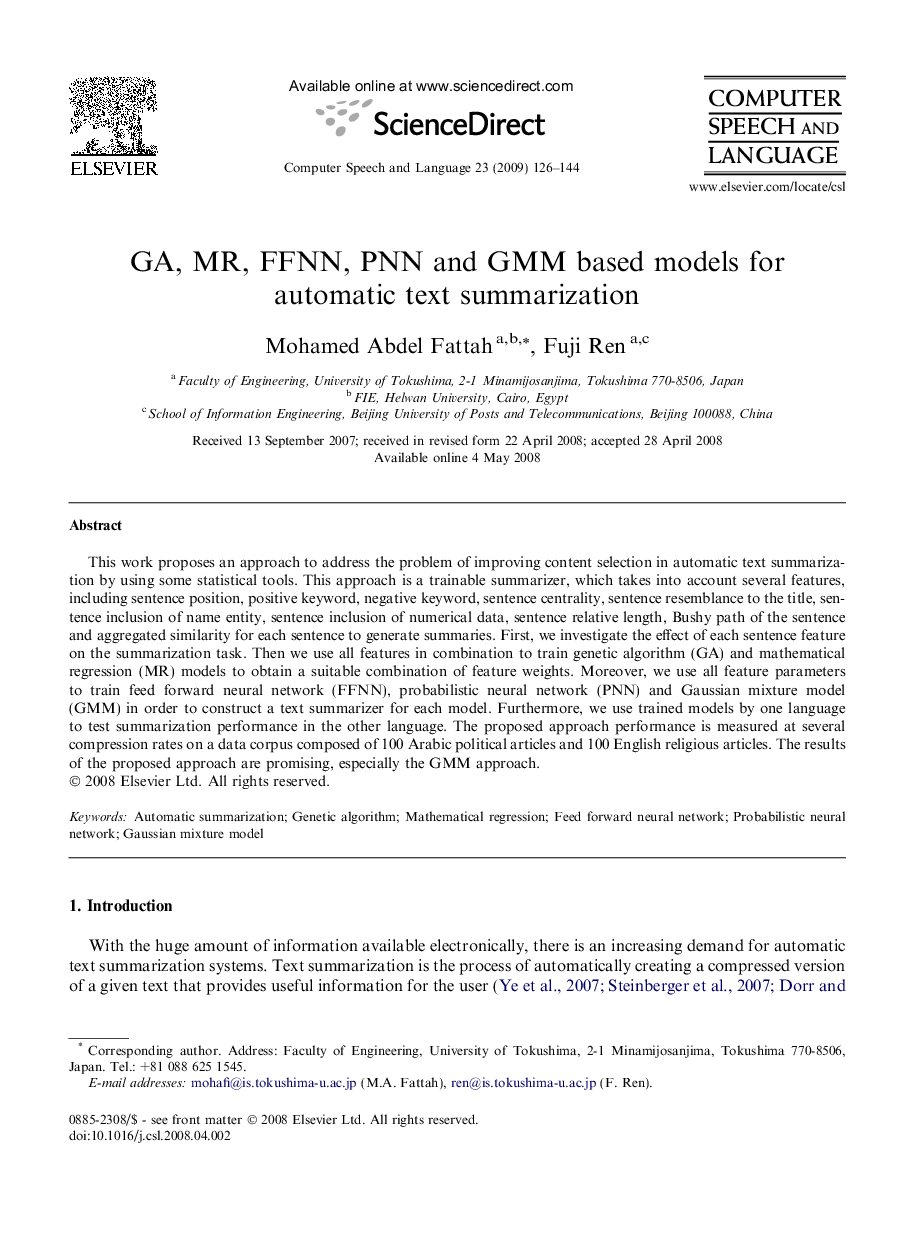
This work proposes an approach to address the problem of improving content selection in automatic text summarization by using some statistical tools. This approach is a trainable summarizer, which takes into account several features, including sentence position, positive keyword, negative keyword, sentence centrality, sentence resemblance to the title, sentence inclusion of name entity, sentence inclusion of numerical data, sentence relative length, Bushy path of the sentence and aggregated similarity for each sentence to generate summaries. First, we investigate the effect of each sentence feature on the summarization task. Then we use all features in combination to train genetic algorithm (GA) and mathematical regression (MR) models to obtain a suitable combination of feature weights. Moreover, we use all feature parameters to train feed forward neural network (FFNN), probabilistic neural network (PNN) and Gaussian mixture model (GMM) in order to construct a text summarizer for each model. Furthermore, we use trained models by one language to test summarization performance in the other language. The proposed approach performance is measured at several compression rates on a data corpus composed of 100 Arabic political articles and 100 English religious articles. The results of the proposed approach are promising, especially the GMM approach.
Journal: Computer Speech & Language - Volume 23, Issue 1, January 2009, Pages 126–144