کد مقاله | کد نشریه | سال انتشار | مقاله انگلیسی | نسخه تمام متن |
---|---|---|---|---|
563657 | 875517 | 2011 | 13 صفحه PDF | دانلود رایگان |
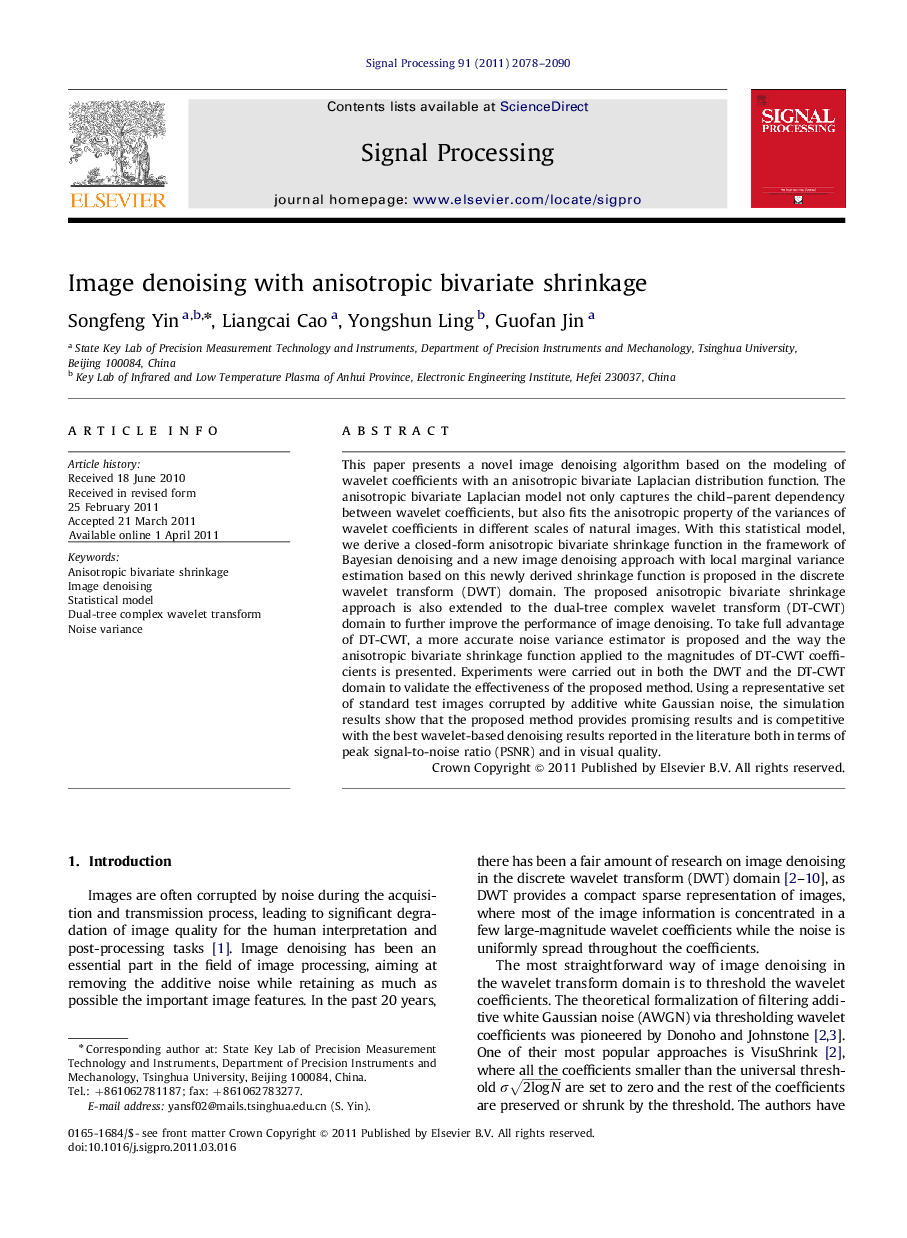
This paper presents a novel image denoising algorithm based on the modeling of wavelet coefficients with an anisotropic bivariate Laplacian distribution function. The anisotropic bivariate Laplacian model not only captures the child–parent dependency between wavelet coefficients, but also fits the anisotropic property of the variances of wavelet coefficients in different scales of natural images. With this statistical model, we derive a closed-form anisotropic bivariate shrinkage function in the framework of Bayesian denoising and a new image denoising approach with local marginal variance estimation based on this newly derived shrinkage function is proposed in the discrete wavelet transform (DWT) domain. The proposed anisotropic bivariate shrinkage approach is also extended to the dual-tree complex wavelet transform (DT-CWT) domain to further improve the performance of image denoising. To take full advantage of DT-CWT, a more accurate noise variance estimator is proposed and the way the anisotropic bivariate shrinkage function applied to the magnitudes of DT-CWT coefficients is presented. Experiments were carried out in both the DWT and the DT-CWT domain to validate the effectiveness of the proposed method. Using a representative set of standard test images corrupted by additive white Gaussian noise, the simulation results show that the proposed method provides promising results and is competitive with the best wavelet-based denoising results reported in the literature both in terms of peak signal-to-noise ratio (PSNR) and in visual quality.
Journal: Signal Processing - Volume 91, Issue 8, August 2011, Pages 2078–2090