کد مقاله | کد نشریه | سال انتشار | مقاله انگلیسی | نسخه تمام متن |
---|---|---|---|---|
565424 | 1451859 | 2016 | 8 صفحه PDF | دانلود رایگان |
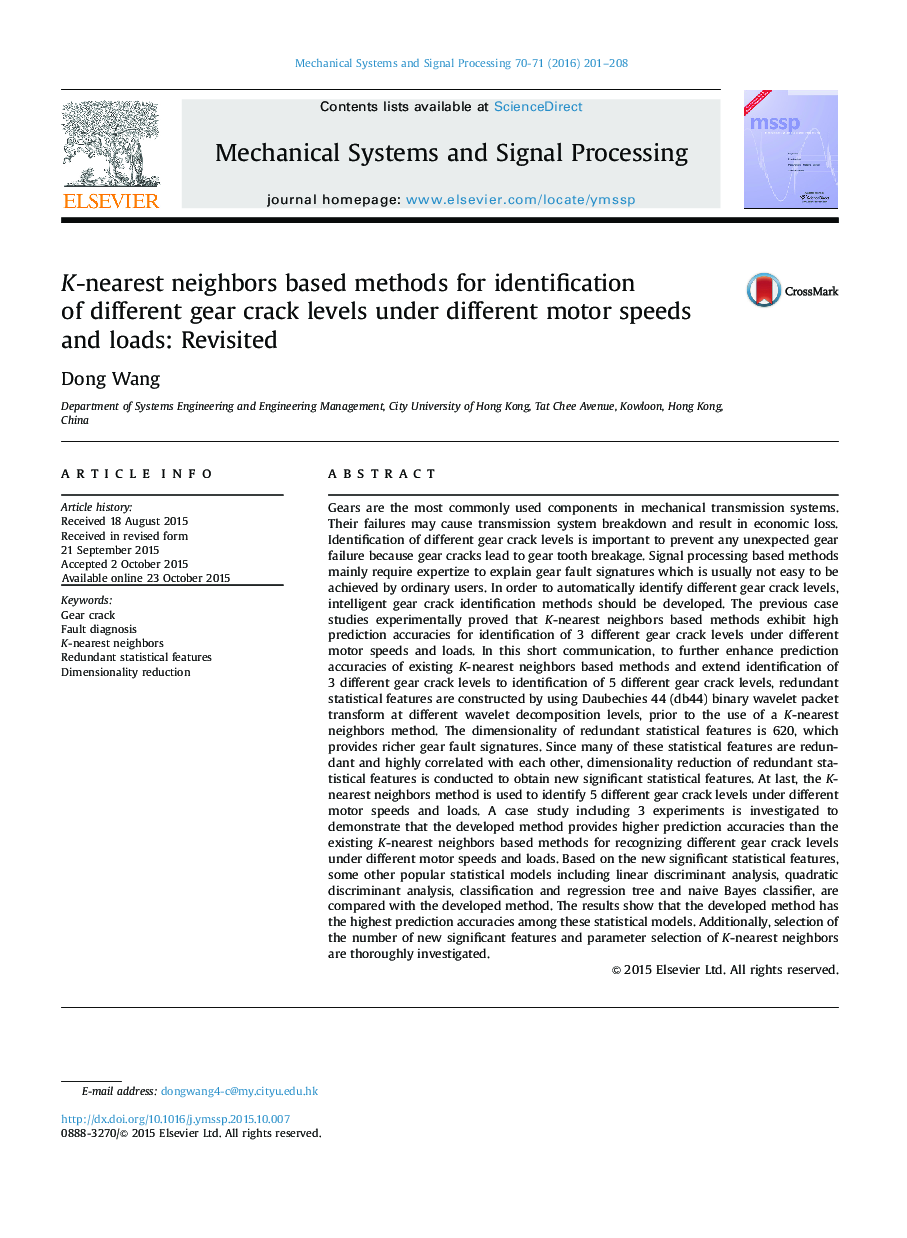
• 620 redundant statistical features are extracted.
• New significant features are generated from 620 redundant features.
• The developed method is better than other 4 K-nearest neighbors based methods.
• Selection of the number of new significant features is investigated.
• Parameter selection of K-nearest neighbors is investigated.
Gears are the most commonly used components in mechanical transmission systems. Their failures may cause transmission system breakdown and result in economic loss. Identification of different gear crack levels is important to prevent any unexpected gear failure because gear cracks lead to gear tooth breakage. Signal processing based methods mainly require expertize to explain gear fault signatures which is usually not easy to be achieved by ordinary users. In order to automatically identify different gear crack levels, intelligent gear crack identification methods should be developed. The previous case studies experimentally proved that K-nearest neighbors based methods exhibit high prediction accuracies for identification of 3 different gear crack levels under different motor speeds and loads. In this short communication, to further enhance prediction accuracies of existing K-nearest neighbors based methods and extend identification of 3 different gear crack levels to identification of 5 different gear crack levels, redundant statistical features are constructed by using Daubechies 44 (db44) binary wavelet packet transform at different wavelet decomposition levels, prior to the use of a K-nearest neighbors method. The dimensionality of redundant statistical features is 620, which provides richer gear fault signatures. Since many of these statistical features are redundant and highly correlated with each other, dimensionality reduction of redundant statistical features is conducted to obtain new significant statistical features. At last, the K-nearest neighbors method is used to identify 5 different gear crack levels under different motor speeds and loads. A case study including 3 experiments is investigated to demonstrate that the developed method provides higher prediction accuracies than the existing K-nearest neighbors based methods for recognizing different gear crack levels under different motor speeds and loads. Based on the new significant statistical features, some other popular statistical models including linear discriminant analysis, quadratic discriminant analysis, classification and regression tree and naive Bayes classifier, are compared with the developed method. The results show that the developed method has the highest prediction accuracies among these statistical models. Additionally, selection of the number of new significant features and parameter selection of K-nearest neighbors are thoroughly investigated.
Journal: Mechanical Systems and Signal Processing - Volumes 70–71, March 2016, Pages 201–208