کد مقاله | کد نشریه | سال انتشار | مقاله انگلیسی | نسخه تمام متن |
---|---|---|---|---|
565489 | 875764 | 2008 | 9 صفحه PDF | دانلود رایگان |
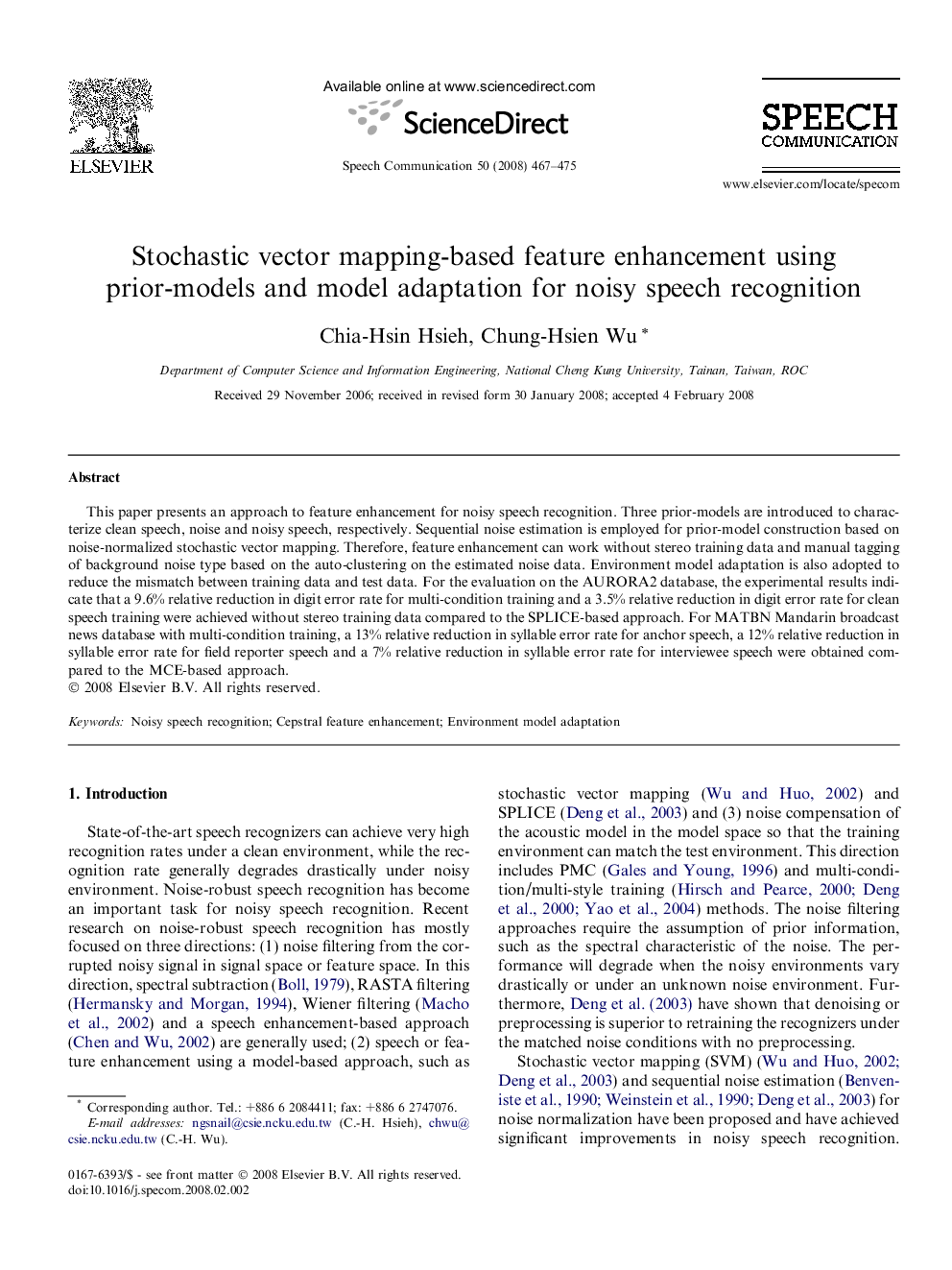
This paper presents an approach to feature enhancement for noisy speech recognition. Three prior-models are introduced to characterize clean speech, noise and noisy speech, respectively. Sequential noise estimation is employed for prior-model construction based on noise-normalized stochastic vector mapping. Therefore, feature enhancement can work without stereo training data and manual tagging of background noise type based on the auto-clustering on the estimated noise data. Environment model adaptation is also adopted to reduce the mismatch between training data and test data. For the evaluation on the AURORA2 database, the experimental results indicate that a 9.6% relative reduction in digit error rate for multi-condition training and a 3.5% relative reduction in digit error rate for clean speech training were achieved without stereo training data compared to the SPLICE-based approach. For MATBN Mandarin broadcast news database with multi-condition training, a 13% relative reduction in syllable error rate for anchor speech, a 12% relative reduction in syllable error rate for field reporter speech and a 7% relative reduction in syllable error rate for interviewee speech were obtained compared to the MCE-based approach.
Journal: Speech Communication - Volume 50, Issue 6, June 2008, Pages 467–475