کد مقاله | کد نشریه | سال انتشار | مقاله انگلیسی | نسخه تمام متن |
---|---|---|---|---|
567126 | 1452043 | 2013 | 16 صفحه PDF | دانلود رایگان |
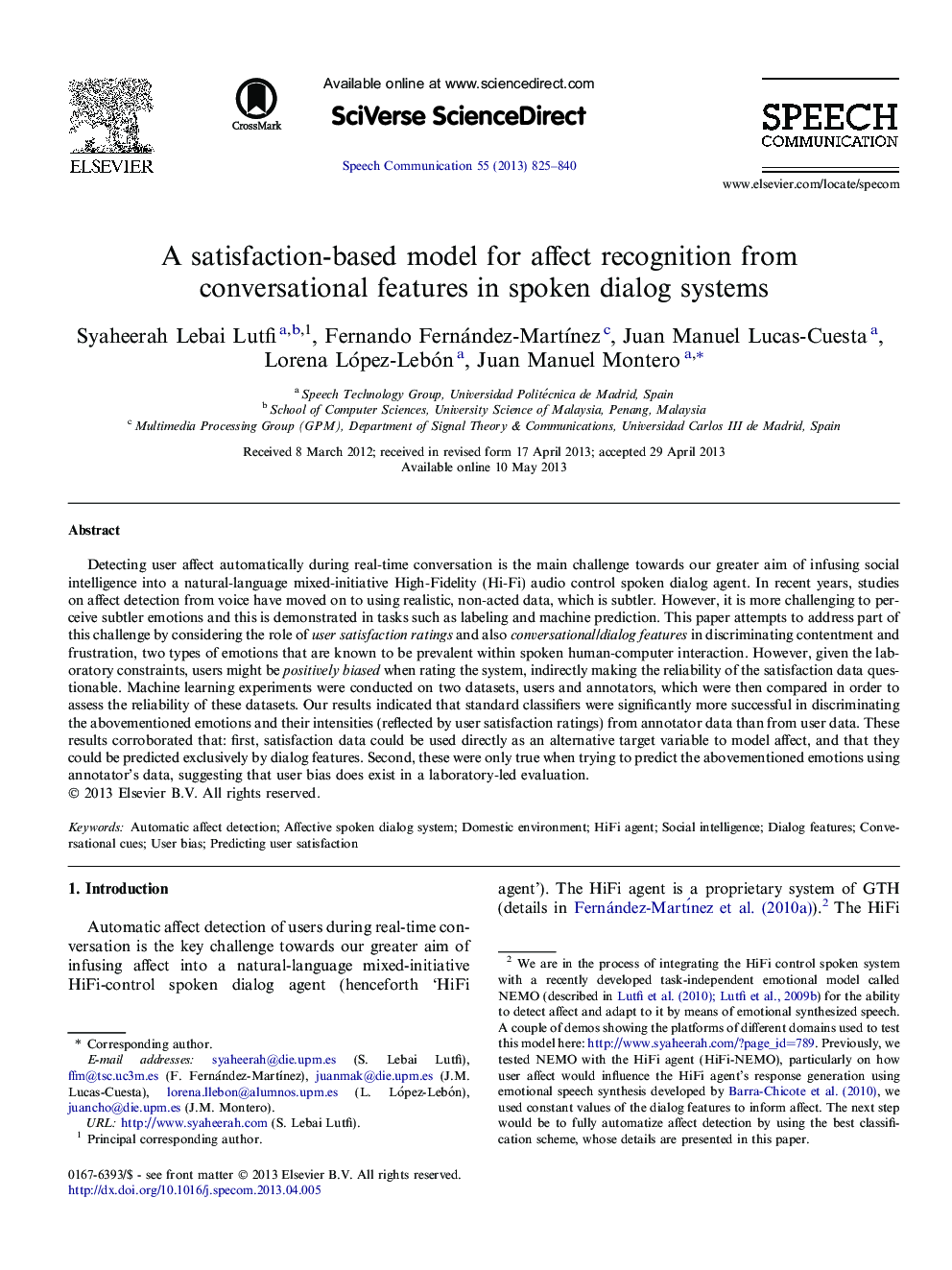
• It is possible to model affect using a corpus that was collected from an evaluation that was originally meant for non-emotion related assessment.
• Conversational features could be used as a single source to model user affect in a limited-task domestic agent.
• Affect could be inferred by directly predicting user satisfaction (modelling satisfaction).
• User bias is empirically detected within a laboratory-led evaluation in this paper.
• The effects of Contextual Turns on user satisfaction depend on user skill.
Detecting user affect automatically during real-time conversation is the main challenge towards our greater aim of infusing social intelligence into a natural-language mixed-initiative High-Fidelity (Hi-Fi) audio control spoken dialog agent. In recent years, studies on affect detection from voice have moved on to using realistic, non-acted data, which is subtler. However, it is more challenging to perceive subtler emotions and this is demonstrated in tasks such as labeling and machine prediction. This paper attempts to address part of this challenge by considering the role of user satisfaction ratings and also conversational/dialog features in discriminating contentment and frustration, two types of emotions that are known to be prevalent within spoken human-computer interaction. However, given the laboratory constraints, users might be positively biased when rating the system, indirectly making the reliability of the satisfaction data questionable. Machine learning experiments were conducted on two datasets, users and annotators, which were then compared in order to assess the reliability of these datasets. Our results indicated that standard classifiers were significantly more successful in discriminating the abovementioned emotions and their intensities (reflected by user satisfaction ratings) from annotator data than from user data. These results corroborated that: first, satisfaction data could be used directly as an alternative target variable to model affect, and that they could be predicted exclusively by dialog features. Second, these were only true when trying to predict the abovementioned emotions using annotator’s data, suggesting that user bias does exist in a laboratory-led evaluation.
Journal: Speech Communication - Volume 55, Issues 7–8, September 2013, Pages 825–840