کد مقاله | کد نشریه | سال انتشار | مقاله انگلیسی | نسخه تمام متن |
---|---|---|---|---|
567498 | 876090 | 2012 | 10 صفحه PDF | دانلود رایگان |
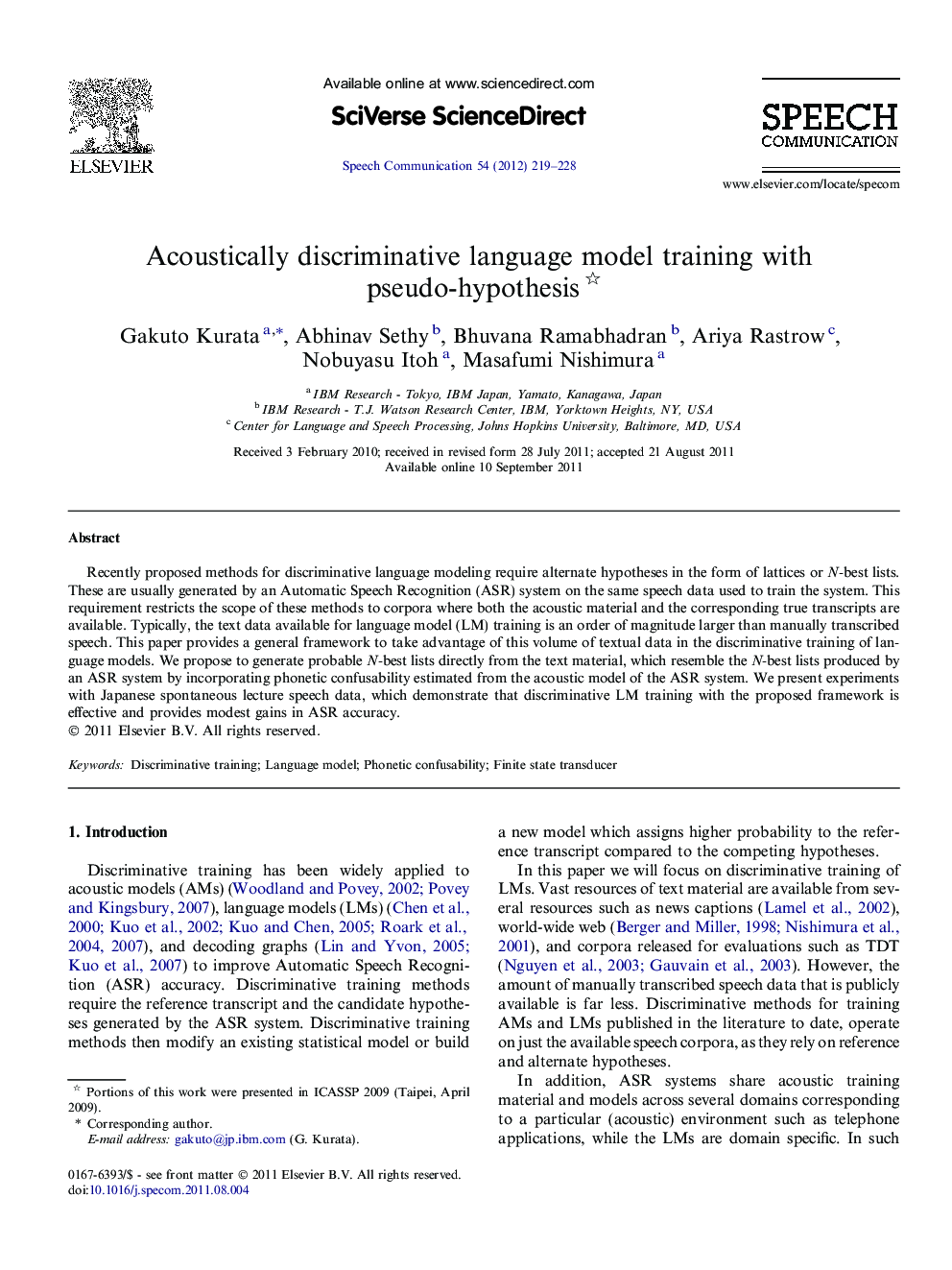
Recently proposed methods for discriminative language modeling require alternate hypotheses in the form of lattices or N-best lists. These are usually generated by an Automatic Speech Recognition (ASR) system on the same speech data used to train the system. This requirement restricts the scope of these methods to corpora where both the acoustic material and the corresponding true transcripts are available. Typically, the text data available for language model (LM) training is an order of magnitude larger than manually transcribed speech. This paper provides a general framework to take advantage of this volume of textual data in the discriminative training of language models. We propose to generate probable N-best lists directly from the text material, which resemble the N-best lists produced by an ASR system by incorporating phonetic confusability estimated from the acoustic model of the ASR system. We present experiments with Japanese spontaneous lecture speech data, which demonstrate that discriminative LM training with the proposed framework is effective and provides modest gains in ASR accuracy.
► We propose a discriminative language model training which does not require audio data.
► We generate the pseudo ASR hypothesis only from textual resources.
► Experiments on a Japanese task showed modest gains in ASR accuracy.
Journal: Speech Communication - Volume 54, Issue 2, February 2012, Pages 219–228