کد مقاله | کد نشریه | سال انتشار | مقاله انگلیسی | نسخه تمام متن |
---|---|---|---|---|
567499 | 876090 | 2012 | 16 صفحه PDF | دانلود رایگان |
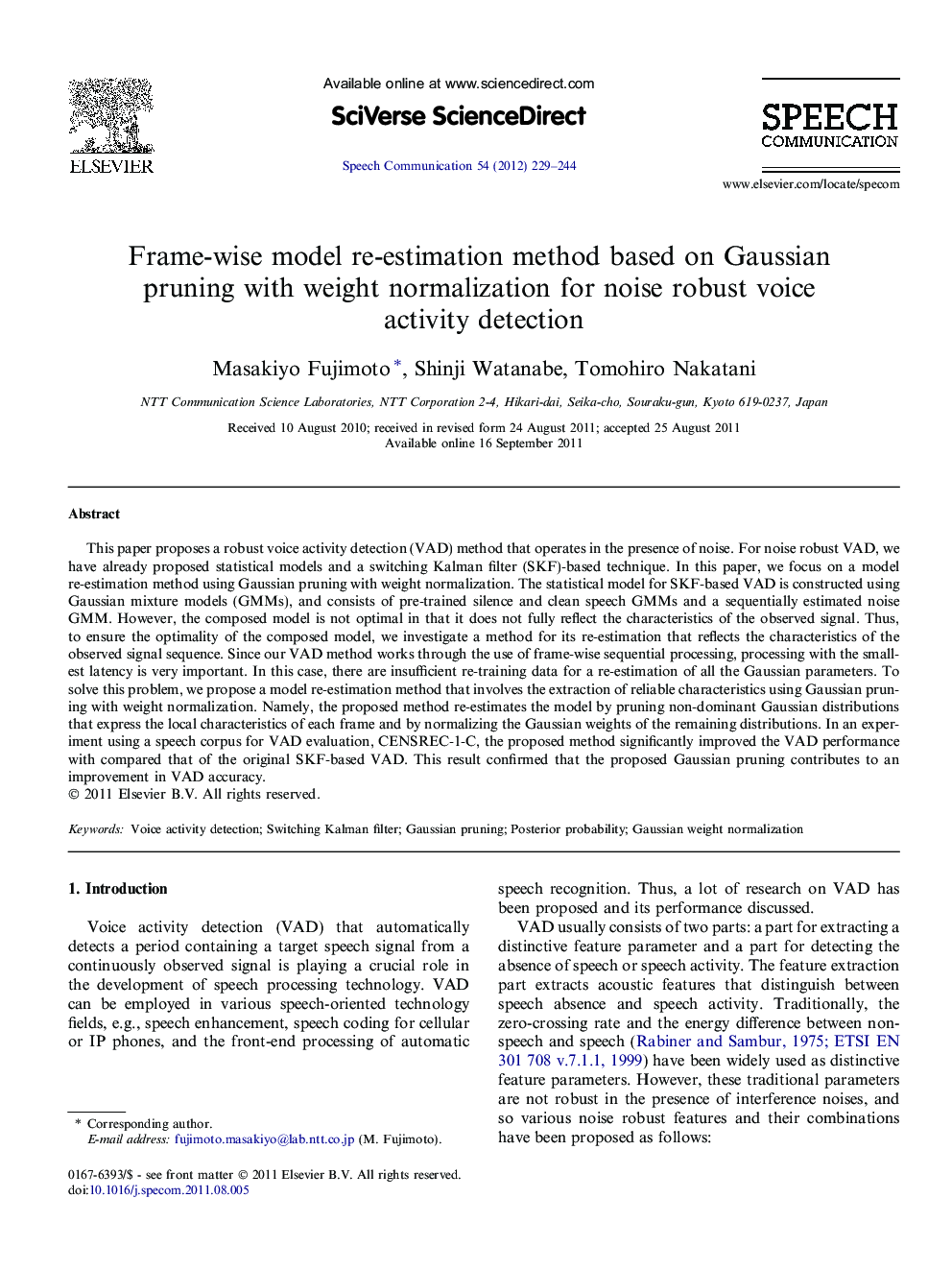
This paper proposes a robust voice activity detection (VAD) method that operates in the presence of noise. For noise robust VAD, we have already proposed statistical models and a switching Kalman filter (SKF)-based technique. In this paper, we focus on a model re-estimation method using Gaussian pruning with weight normalization. The statistical model for SKF-based VAD is constructed using Gaussian mixture models (GMMs), and consists of pre-trained silence and clean speech GMMs and a sequentially estimated noise GMM. However, the composed model is not optimal in that it does not fully reflect the characteristics of the observed signal. Thus, to ensure the optimality of the composed model, we investigate a method for its re-estimation that reflects the characteristics of the observed signal sequence. Since our VAD method works through the use of frame-wise sequential processing, processing with the smallest latency is very important. In this case, there are insufficient re-training data for a re-estimation of all the Gaussian parameters. To solve this problem, we propose a model re-estimation method that involves the extraction of reliable characteristics using Gaussian pruning with weight normalization. Namely, the proposed method re-estimates the model by pruning non-dominant Gaussian distributions that express the local characteristics of each frame and by normalizing the Gaussian weights of the remaining distributions. In an experiment using a speech corpus for VAD evaluation, CENSREC-1-C, the proposed method significantly improved the VAD performance with compared that of the original SKF-based VAD. This result confirmed that the proposed Gaussian pruning contributes to an improvement in VAD accuracy.
► We propose a model re-estimation method for statistical model-based VAD.
► The method extracts reliable features by Gaussian pruning with weight normalization.
► Gaussian pruning reduces non-dominant Gaussian distributions at each frame.
► The remaining distributions are enhanced by normalizing the Gaussian weights.
► The accurate VAD decision is obtained by likelihoods of the remaining distributions.
Journal: Speech Communication - Volume 54, Issue 2, February 2012, Pages 229–244